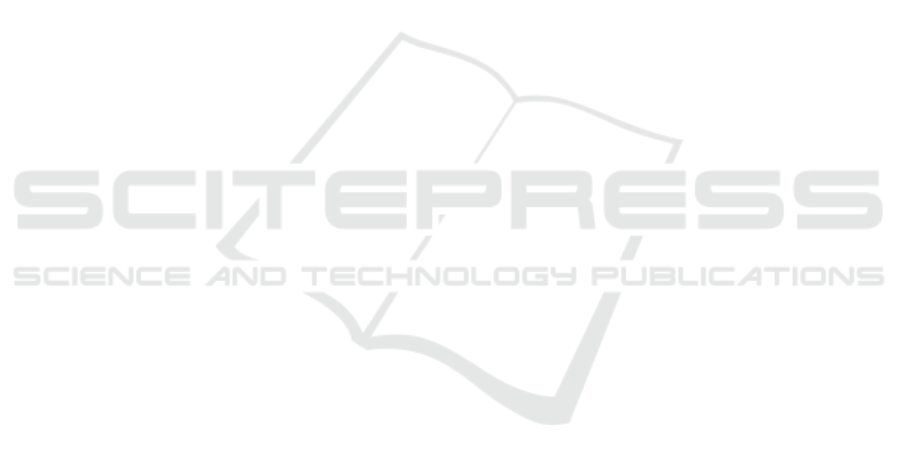
dimensional data, different granularities may exist.
Choosing the appropriate level of detail or granularity
is crucial. To mitigate this challenge, we plan to ex-
tend the AVAR-based approach to represent different
levels of resolution by creating a hierarchical struc-
ture of spatio-temporal data. In addition, we will fo-
cus whether and when to recalculate the characteristic
timescale, as the AVAR estimator would be useful in
an incremental scenario when data keeps coming in.
Furthermore, we intend to construct dynamic AVAR
estimators which can cope with local changes in the
temporal and spatial characteristic sizes.
REFERENCES
Allan, D. W. (1966). Statistics of atomic frequency stan-
dards. Proceedings of the IEEE, 54(2):221–230.
Bezdek, J. C., Ehrlich, R., and Full, W. (1984). FCM:
The fuzzy c-means clustering algorithm. Computers
& Geosciences, 10(2-3):191–203.
Dua, D. and Graff, C. (2019). UCI machine learning repos-
itory, 2017. URL http://archive.ics.uci.edu/ml.
Gray, J., Chaudhuri, S., Bosworth, A., Layman, A., Re-
ichart, D., Venkatrao, M., Pellow, F., and Pirahesh, H.
(1997). Data cube: A relational aggregation operator
generalizing group-by, cross-tab, and sub-totals. Data
Mining and Knowledge Discovery, 1(1):29–53.
Haeri, H., Beal, C. E., and Jerath, K. (2021). Near-
optimal moving average estimation at characteristic
timescales: An Allan variance approach. IEEE Con-
trol Systems Letters, 5(5):1531–1536.
Hartigan, J. A. (1975). Clustering algorithms. John Wiley
& Sons, Inc.
Helsen, J., Peeters, C., Doro, P., Ververs, E., and Jordaens,
P. J. (2017). Wind farm operation and maintenance
optimization using big data. In 2017 IEEE Third Inter-
national Conference on Big Data Computing Service
and Applications (BigDataService), pages 179–184.
Henrikson, J. (1999). Completeness and total boundedness
of the hausdorff metric. MIT Undergraduate Journal
of Mathematics, 1:69–80.
IEEE (2019). IEEE standard for floating-point arithmetic.
IEEE Std 754-2019 (Revision of IEEE 754-2008),
pages 1–84.
Januzaj, E., Kriegel, H.-P., and Pfeifle, M. (2004). Dbdc:
Density based distributed clustering. In Interna-
tional Conference on Extending Database Technol-
ogy, pages 88–105. Springer.
Jerath, K., Brennan, S., and Lagoa, C. (2018). Bridging the
gap between sensor noise modeling and sensor char-
acterization. Measurement, 116:350 – 366.
Johnston, W. (2001). Model Visualization, page 223–227.
Morgan Kaufmann Publishers Inc., San Francisco,
CA, USA.
Kaufmann, L. (1987). Clustering by means of medoids. In
Proc. Statistical Data Analysis Based on the L1 Norm
Conference, Neuchatel, 1987, pages 405–416.
Keogh, E. and Mueen, A. (2017). Curse of dimensional-
ity. In Encyclopedia of Machine Learning and Data
Mining, pages 314–315.
Kile, H. and Uhlen, K. (2012). Data reduction via clustering
and averaging for contingency and reliability analysis.
International Journal of Electrical Power & Energy
Systems, 43(1):1435–1442.
Kodinariya, T. M. and Makwana, P. R. (2013). Review on
determining number of cluster in k-means clustering.
International Journal, 1(6):90–95.
Liu, H. and Motoda, H. (2002). On issues of instance selec-
tion. Data Min. Knowl. Discov., 6:115–130.
Lumini, A. and Nanni, L. (2006). A clustering method
for automatic biometric template selection. Pattern
Recognition, 39(3):495–497.
MacQueen, J. et al. (1967). Some methods for classification
and analysis of multivariate observations. In Proceed-
ings of the Fifth Berkeley symposium on mathematical
statistics and probability, volume 1, pages 281–297.
Oakland, CA, USA.
Madigan, D. and Nason, M. (2002). Data reduction: sam-
pling. In Handbook of data mining and knowledge
discovery, pages 205–208.
Mishra, A. D. and Garg, D. (2008). Selection of best sorting
algorithm. International Journal of intelligent infor-
mation Processing, 2(2):363–368.
Olvera-L
´
opez, J. A., Carrasco-Ochoa, J. A., and Mart
´
ınez-
Trinidad, J. F. (2010). A new fast prototype selection
method based on clustering. Pattern Analysis and Ap-
plications, 13(2):131–141.
Pedrycz, W. (2001). Granular computing: an introduc-
tion. In Proceedings joint 9th IFSA world congress
and 20th NAFIPS international conference (Cat. No.
01TH8569), volume 3, pages 1349–1354. IEEE.
Prasad Maddipatla, S., Haeri, H., Jerath, K., and Bren-
nan, S. (To appear in October 2021). Fast Allan vari-
ance (FAVAR) and dynamic fast Allan variance (D-
FAVAR) algorithms for both regularly and irregularly
sampled data. Modeling, Estimation and Control Con-
ference.
Rehman, M. H., Liew, C. S., Abbas, A., Jayaraman, P. P.,
Wah, T. Y., and Khan, S. U. (2016). Big data reduction
methods: a survey. Data Science and Engineering,
1(4):265–284.
Sesia, I. and Tavella, P. (2008). Estimating the Allan vari-
ance in the presence of long periods of missing data
and outliers. Metrologia, 45(6):S134.
Sun, X., Liu, L., Geng, C., and Yang, S. (2019). Fast data re-
duction with granulation-based instances importance
labeling. IEEE Access, 7:33587–33597.
Zadeh, L. A. (1997). Toward a theory of fuzzy information
granulation and its centrality in human reasoning and
fuzzy logic. Fuzzy sets and systems, 90(2):111–127.
KMIS 2021 - 13th International Conference on Knowledge Management and Information Systems
28