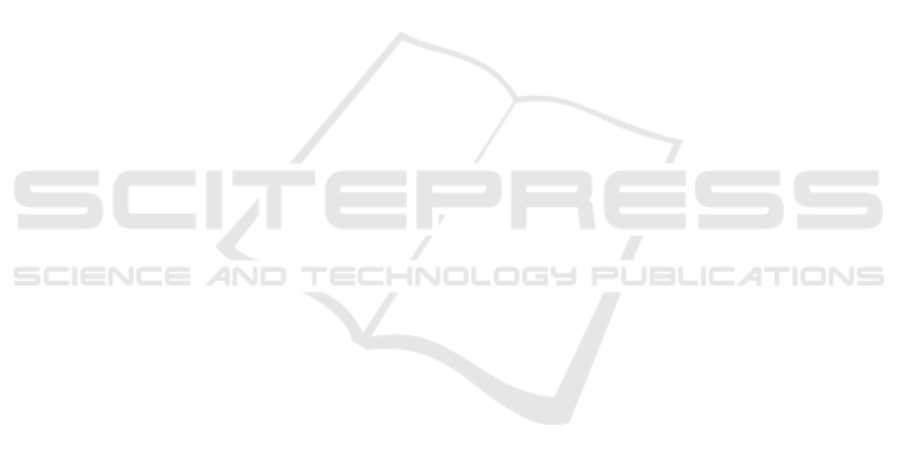
Erk, K. (2012). Vector space models of word meaning and
phrase meaning: A survey. Language and Linguistics
Compass, 6(10):635–653.
Etzioni, O., Cafarella, M., Downey, D., Kok, S., Popescu,
A.-M., Shaked, T., Soderland, S., Weld, D. S., and
Yates, A. (2004). Web-scale information extraction
in knowitall: (preliminary results). In Proceedings of
the 13th international conference on World Wide Web,
pages 100–110.
Faruqui, M., Dodge, J., Jauhar, S. K., Dyer, C., Hovy, E.,
and Smith, N. A. (2014). Retrofitting word vectors to
semantic lexicons. arXiv preprint arXiv:1411.4166.
Firth, J. R. (1957). A synopsis of linguistic theory 1930-55.
Studies in Linguistic Analysis, Special vol:1–32.
Gross, M. (1994). Computational approaches to the lexicon.
chapter Constructing Lexicon-Grammars, pages 213–
263. Oxford University Press.
Harris, Z. S. (1954). Distributional structure. Word, 10(2-
3):146–162.
Hearst, M. (1998). Wordnet: An electronic lexical database
and some of its applications. Automated Discovery of
WordNet Relations.
Hearst, M. A. (1992). Automatic acquisition of hyponyms
from large text corpora. In Coling 1992 volume 2:
The 15th international conference on computational
linguistics.
Kanerva, P., Kristoferson, J., and Holst, A. (2000). Random
indexing of text samples for latent semantic analysis.
In Proceedings of the Annual Meeting of the Cognitive
Science Society, volume 22.
Khoja, S. and Garside, R. (1999). Stemming arabic text.
Lancaster, UK, Computing Department, Lancaster
University.
Kiela, D., Hill, F., and Clark, S. (2015). Specializing word
embeddings for similarity or relatedness. In Proceed-
ings of the 2015 Conference on Empirical Methods in
Natural Language Processing, pages 2044–2048.
Kohonen, T. (1982). Self-organized formation of topolog-
ically correct feature maps. Biological cybernetics,
43(1):59–69.
Lazaridou, A., Baroni, M., et al. (2015). A multitask objec-
tive to inject lexical contrast into distributional seman-
tics. In Proceedings of the 53rd Annual Meeting of the
Association for Computational Linguistics and the 7th
International Joint Conference on Natural Language
Processing (Volume 2: Short Papers), pages 21–26.
Lebboss, G. (2016). Contribution
`
a l’analyse s
´
emantique
des textes arabes. PhD thesis, Paris 8.
Lebboss, G., Bernard, G., Aliane, N., Abdallah, A., and
Hajjar, M. (2019). Evaluating methods for building
arabic semantic resources with big corpora. In Stud-
ies in Computational Intelligence, volume 829, pages
179–197. Springer International Publishing.
Lebboss, G., Bernard, G., Aliane, N., and Hajjar, M. (2017).
Towards the enrichment of Arabic WordNet with big
corpora. In IJCCI, pages 101–109.
Levy, O. and Goldberg, Y. (2014). Dependency-based word
embeddings. In Proceedings of the 52nd Annual Meet-
ing of the Association for Computational Linguistics
(Volume 2: Short Papers), pages 302–308.
Levy, O., Goldberg, Y., and Dagan, I. (2015a). Improv-
ing distributional similarity with lessons learned from
word embeddings. Transactions of the Association for
Computational Linguistics, 3:211–225.
Levy, O., Remus, S., Biemann, C., and Dagan, I. (2015b).
Do supervised distributional methods really learn lex-
ical inference relations? In Proceedings of the 2015
Conference of the North American Chapter of the As-
sociation for Computational Linguistics: Human Lan-
guage Technologies, pages 970–976.
Lin, D. (1998). Automatic retrieval and clustering of simi-
lar words. In 36th Annual Meeting of the Association
for Computational Linguistics and 17th International
Conference on Computational Linguistics, Volume 2,
pages 768–774.
Lin, D., Zhao, S., Qin, L., and Zhou, M. (2003). Identifying
synonyms among distributionally similar words. In
IJCAI, volume 3, pages 1492–1493. Citeseer.
Liu, Q., Jiang, H., Wei, S., Ling, Z.-H., and Hu, Y. (2015).
Learning semantic word embeddings based on ordi-
nal knowledge constraints. In Proceedings of the 53rd
Annual Meeting of the Association for Computational
Linguistics and the 7th International Joint Conference
on Natural Language Processing (Volume 1: Long Pa-
pers), pages 1501–1511.
Lund, K., Burgess, C., and Atchley, R. A. (1995). Semantic
and associative priming in high-dimensional semantic
space. In Proceedings of the 17th annual conference
of the Cognitive Science Society, volume 17, pages
660–665.
Melka, J. and Mariage, J.-J. (2017). Efficient implementa-
tion of self-organizing map for sparse input data. In
IJCCI, pages 54–63.
Mikolov, T., Chen, K., Corrado, G., and Dean, J. (2013a).
Efficient estimation of word representations in vector
space. arXiv preprint arXiv:1301.3781.
Mikolov, T., Yih, W.-t., and Zweig, G. (2013b). Linguis-
tic regularities in continuous space word representa-
tions. In Proceedings of the 2013 conference of the
north american chapter of the association for com-
putational linguistics: Human language technologies,
pages 746–751.
Miller, G. A. (1957). Some effects of intermittent silence.
The American Journal of Psychology, 70(2):311.
Mnih, A. and Hinton, G. E. (2008). A scalable hierarchi-
cal distributed language model. Advances in neural
information processing systems, 21:1081–1088.
Mnih, A. and Kavukcuoglu, K. (2013). Learning word em-
beddings efficiently with noise-contrastive estimation.
Advances in neural information processing systems,
26:2265–2273.
Mrk
ˇ
si
´
c, N., S
´
eaghdha, D. O., Thomson, B., Ga
ˇ
si
´
c, M.,
Rojas-Barahona, L., Su, P.-H., Vandyke, D., Wen,
T.-H., and Young, S. (2016). Counter-fitting word
vectors to linguistic constraints. arXiv preprint
arXiv:1603.00892.
Murphy, B., Talukdar, P., and Mitchell, T. (2012). Learn-
ing effective and interpretable semantic models using
non-negative sparse embedding. In Proceedings of
COLING 2012, pages 1933–1950.
Nozza, D., Fersini, E., and Messina, E. (2016). Unsuper-
vised irony detection: a probabilistic model with word
Unsupervised Grammatical Pattern Discovery from Arabic Extra Large Corpora
219