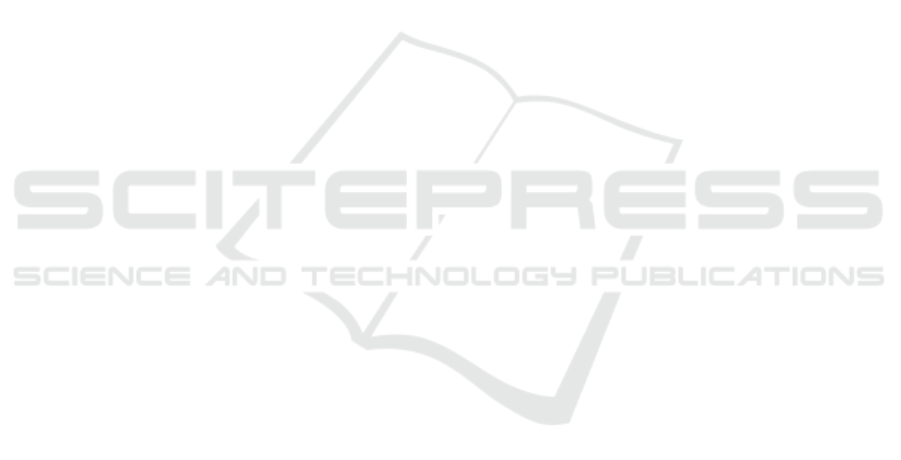
Khemais, Z., Nesrine, D., Mohamed, M., et al. (2016).
Credit scoring and default risk prediction: A compar-
ative study between discriminant analysis & logistic
regression. International Journal of Economics and
Finance, 8(4):39.
Kim, Y. and Sohn, S. (2012). Stock fraud detection us-
ing peer group analysis. Expert Systems with Applica-
tions, 39(10):8986–8992.
King, G. and Zeng, L. (2001). Logistic regression in rare
events data. Political analysis, 9(2):137–163.
Lee, B. K. and Sohn, S. Y. (2017). A credit scoring model
for smes based on accounting ethics. Sustainability,
9(9).
Leevy, J. L., Khoshgoftaar, T. M., Bauder, R. A., and Seliya,
N. (2018). A survey on addressing high-class imbal-
ance in big data. Journal of Big Data, 5(1):42.
Lei, K., Xie, Y., Zhong, S., Dai, J., Yang, M., and Shen,
Y. (2019). Generative adversarial fusion network for
class imbalance credit scoring. Neural Computing and
Applications, pages 1–12.
Liu, C., Huang, H., and Lu, S. (2019). Research on personal
credit scoring model based on artificial intelligence. In
International Conference on Application of Intelligent
Systems in Multi-modal Information Analytics, pages
466–473. Springer.
Luo, C., Wu, D., and Wu, D. (2017). A deep learn-
ing approach for credit scoring using credit default
swaps. Engineering Applications of Artificial Intel-
ligence, 65:465–470.
Malekipirbazari, M. and Aksakalli, V. (2015). Risk assess-
ment in social lending via random forests. Expert Sys-
tems with Applications, 42(10):4621–4631.
Roy, A. G. and Urolagin, S. (2019). Credit risk assess-
ment using decision tree and support vector machine
based data analytics. In Creative Business and So-
cial Innovations for a Sustainable Future, pages 79–
84. Springer.
Roy, P. and Shaw, K. (2021). A credit scoring model for
smes using ahp and topsis. International Journal of
Finance and Economics.
Saia, R. and Carta, S. (2016a). An entropy based algorithm
for credit scoring. In International Conference on Re-
search and Practical Issues of Enterprise Information
Systems, pages 263–276. Springer.
Saia, R. and Carta, S. (2016b). Introducing a vector space
model to perform a proactive credit scoring. In In-
ternational Joint Conference on Knowledge Discov-
ery, Knowledge Engineering, and Knowledge Man-
agement, pages 125–148. Springer.
Saia, R. and Carta, S. (2016c). A linear-dependence-based
approach to design proactive credit scoring models. In
KDIR, pages 111–120.
Saia, R. and Carta, S. (2017a). Evaluating credit card trans-
actions in the frequency domain for a proactive fraud
detection approach. In SECRYPT, pages 335–342.
Saia, R. and Carta, S. (2017b). A fourier spectral pattern
analysis to design credit scoring models. In Proceed-
ings of the 1st International Conference on Internet of
Things and Machine Learning, page 18. ACM.
Saia, R., Carta, S., et al. (2017). A frequency-domain-
based pattern mining for credit card fraud detection.
In IoTBDS, pages 386–391.
Saia, R., Carta, S., and Fenu, G. (2018a). A wavelet-based
data analysis to credit scoring. In Proceedings of the
2nd International Conference on Digital Signal Pro-
cessing, pages 176–180. ACM.
Saia, R., Carta, S., and Recupero, D. R. (2018b). A
probabilistic-driven ensemble approach to perform
event classification in intrusion detection system. In
KDIR, pages 139–146.
Saia, R., Carta, S., Recupero, D. R., Fenu, G., and Saia, M.
(2019a). A discretized enriched technique to enhance
machine learning performance in credit scoring. In
KDIR, pages 202–213.
Saia, R., Carta, S., Recupero, D. R., Fenu, G., and Stan-
ciu, M. (2019b). A discretized extended feature space
(defs) model to improve the anomaly detection per-
formance in network intrusion detection systems. In
KDIR, pages 322–329.
Siddiqi, N. (2017). Intelligent credit scoring: Building and
implementing better credit risk scorecards. John Wi-
ley & Sons.
Sloan, R. H. and Warner, R. (2018). When is an algorithm
transparent? predictive analytics, privacy, and public
policy. IEEE Security & Privacy, 16(3):18–25.
Sohn, S. Y., Kim, D. H., and Yoon, J. H. (2016). Technol-
ogy credit scoring model with fuzzy logistic regres-
sion. Applied Soft Computing, 43:150–158.
Thomas, L., Crook, J., and Edelman, D. (2017). Credit
Scoring and Its Applications, Second Edition. Society
for Industrial and Applied Mathematics, Philadelphia,
PA.
Tripathi, D., Edla, D. R., and Cheruku, R. (2018). Hy-
brid credit scoring model using neighborhood rough
set and multi-layer ensemble classification. Journal
of Intelligent & Fuzzy Systems, 34(3):1543–1549.
Weiss, G. M. (2004). Mining with rarity: A unifying frame-
work. SIGKDD Explor. Newsl., 6(1):7–19.
Xia, Y., He, L., Li, Y.-G., Fu, Y., and Xu, Y. (2020). A dy-
namic credit scoring model based on survival gradient
boosting decision tree approach. Technological and
Economic Development of Economy, pages 1–24.
Zhang, W., He, H., and Zhang, S. (2019). A novel multi-
stage hybrid model with enhanced multi-population
niche genetic algorithm: An application in credit scor-
ing. Expert Systems with Applications, 121:221–232.
Zhang, X., Yang, Y., and Zhou, Z. (2018). A novel credit
scoring model based on optimized random forest. In
2018 IEEE 8th Annual Computing and Communica-
tion Workshop and Conference (CCWC), pages 60–
65. IEEE.
From Payment Services Directive 2 (PSD2) to Credit Scoring: A Case Study on an Italian Banking Institution
171