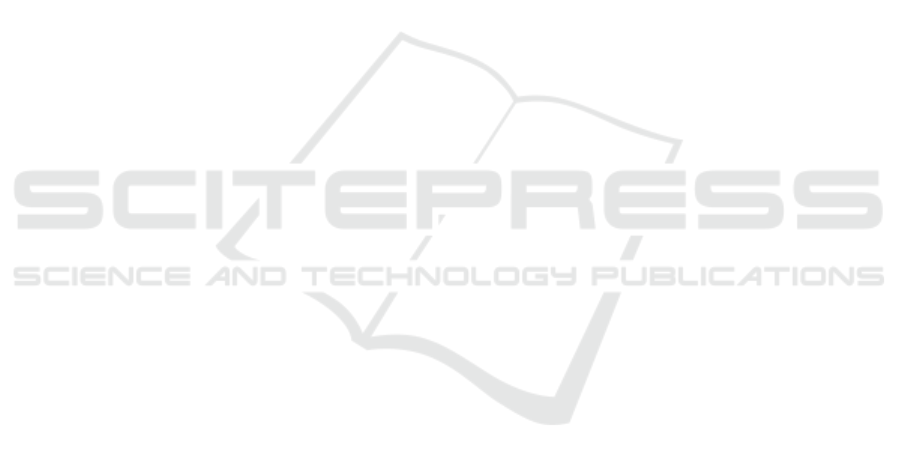
Fayyad, U., Piatetsky-Shapiro, G., and Smyth, P. (1996).
The kdd process for extracting useful knowledge from
volumes of data. Comunications of the ACM.
G. Chandrashekar, F. S. (2014). A survey on feature selec-
tion methods. Computers & Electrical Engineering,
41(1):16–28.
H. Liu, H. M. (2012). Feature selection for knowledge dis-
covery and data mining. Springer Science & Business
Media, 454.
Hall, P., Pittelkow, Y., and Ghosh, M. (2008). Theoreti-
cal measures of relative performance of classifiers for
high dimensional data with small sample sizes. Jour-
nal of the Royal Statistical Society: Series B (Statisti-
cal Methodology), 70(1):159–173.
Haoyue Liu, Meng Chu Zhou, Q. L. (2019). An embedded
feature selection method for imbalanced data classifi-
cation. IEEE, JOURNAL OF AUTOMATICA SINICA,
6.
Hashemian, H. M. (2010). State-of-the-art predictive main-
tenance techniques. IEEE Transactions on Instrumen-
tation and measurement, 60(1):226–236.
Kaisler, S., Armour, F., Espinosa, J. A., and Money, W.
(2013). Big data: Issues and challenges moving for-
ward. In 2013 46th Hawaii International Conference
on System Sciences, pages 995–1004. IEEE.
Katal, A., Wazid, M., and Goudar, R. H. (2013). Big data:
issues, challenges, tools and good practices. In 2013
Sixth international conference on contemporary com-
puting (IC3), pages 404–409. IEEE.
Ke Yana, D. Z. (2015). Feature selection and analysis on
correlated gas sensor data with recursive feature elim-
ination. Elsevier, 212.
Khan, W. Z., Rehman, M., Zangoti, H. M., Afzal, M. K.,
Armi, N., and Salah, K. (2020). Industrial internet of
things: Recent advances, enabling technologies and
open challenges. Computers & Electrical Engineer-
ing, 81:106522.
Lei Yu leiyu, H. L. (2003). Feature selection for high-
dimensional data: A fast correlation-based filter solu-
tion. Proc. Int. Conf. Machine Learning, 3:856–863.
Lu, M. (2019). Embedded feature selection accounting for
unknown data heterogeneity. Elsevier, Expert Systems
with Applications, 119.
Lu, Y. (2017). Industry 4.0: A survey on technologies, ap-
plications and open research issues. Journal of indus-
trial information integration, 6:1–10.
Miner, S. E. Seema methdology, sas institute.
Mourtzis, D., Vlachou, E., and Milas, N. (2016). Industrial
big data as a result of iot adoption in manufacturing.
Procedia cirp, 55:290–295.
NADA ALMUGREN, H. A. (2019). A survey on hy-
brid feature selection methods in microarray gene ex-
pression data for cancer classification. IEEE Access,
7:78533–78548.
Nedelcu, E., Portase, R., Tolas, R., Muresan, R., Dinsore-
anu, M., and Potolea, R. (2017). Artifact detection in
eeg using machine learning. In 2017 13th IEEE Inter-
national Conference on Intelligent Computer Commu-
nication and Processing (ICCP), pages 77–83. IEEE.
Olariu, E., Tolas, R., Portase, R., Dinsoreanu, M., and Po-
tolea, R. (2020). Modern approaches to preprocessing
industrial data. In 2020 16th IEEE International Con-
ference on Intelligent Computer Communication and
Processing (ICCP). IEEE.
Pech, M., Vrchota, J., and Bedn
´
a
ˇ
r, J. (2021). Predictive
maintenance and intelligent sensors in smart factory.
Sensors, 21(4):1470.
Powell, M. J. D. et al. (1981). Approximation theory and
methods. Cambridge university press.
Puech, T., Boussard, M., D’Amato, A., and Millerand, G.
(2019). A fully automated periodicity detection in
time series. In International Workshop on Advanced
Analysis and Learning on Temporal Data, pages 43–
54. Springer.
Ratner, B. (2017). Statistical and Machine-Learning Data
Mining:: Techniques for Better Predictive Modeling
and Analysis of Big Data. CRC Press.
Shilaskar, S. and Ghatol, A. (2013). Feature selection for
medical diagnosis: Evaluation for cardiovascular dis-
eases. Expert Syst. with Applications, 40.
Sipos, R., Fradkin, D., Moerchen, F., and Wang, Z. (2014).
Log-based predictive maintenance. In Proceedings of
the 20th ACM SIGKDD international conference on
knowledge discovery and data mining, pages 1867–
1876.
Smith, P., Apple, D., Beyerlein, S., and Holmes, C. (2006).
Methodology for creating methodologies. Faculty
Guidebook. Lisle, Illinois: Pacific Crest, pages 371–
4.
Storcheus, D., Rostamizadeh, A., and Kumar, S. (2015). A
survey of modern questions and challenges in feature
extraction. In Feature Extraction: Modern Questions
and Challenges, pages 1–18.
Tolas, R., Portase, R., Iosif, A., and Potolea, R. (2021). Pe-
riodicity detection algorithm and applications on iot
data. In 20th International Symposium on Parallel and
Distributed Computing (ISPDC 2021).
Uddin, M. F., Gupta, N., et al. (2014). Seven v’s of big data
understanding big data to extract value. In Proceed-
ings of the 2014 zone 1 conference of the American
Society for Engineering Education, pages 1–5. IEEE.
V. Bol
´
on-Canedo, N. S
´
anchez-Marono, A. A.-B. J. M. B.
F. H. (2014). A review of microarray datasets and ap-
plied feature selection methods. Information Sciences,
282:1110135.
Wang, P., Vachtsevanos, G., et al. (2001). Fault prognostics
using dynamic wavelet neural networks. AI EDAM,
15(4):349–365.
Wirth, R. and Hipp, J. (2000). Crisp-dm: Towards a stan-
dard process model for data mining. Proceedings of
the 4th International Conference on the Practical Ap-
plications of Knowledge Discovery and Data Mining.
KDIR 2021 - 13th International Conference on Knowledge Discovery and Information Retrieval
198