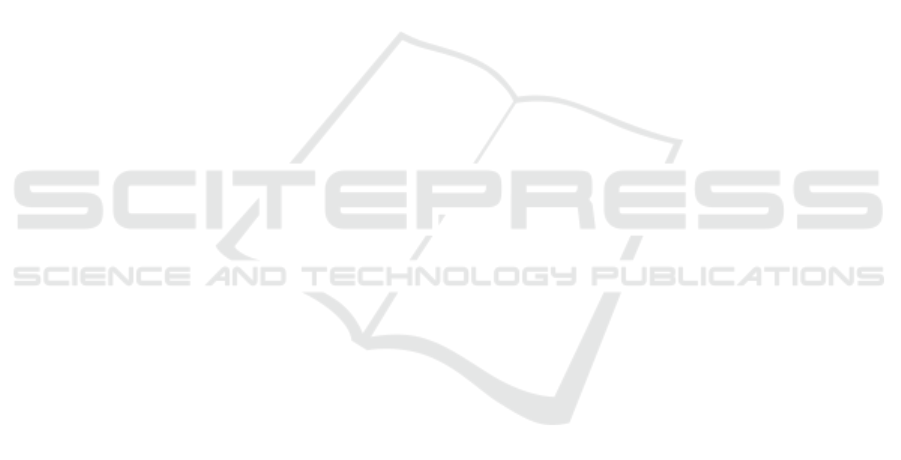
ately designed to be simple and domain independent,
requiring no domain knowledge or specific calibra-
tion. This makes SF more easy to implement than
other techniques and offers the possibility of being
more generally applicable.
However, one of the potential weaknesses ob-
served in SF is the highly-fluctuating behaviour in-
duced in populations (i.e., the “cull” effect), which
might lead to sudden disengagement in other more re-
alistic domains. Thus, we believe that SF deserves
further exploration; although it has shown suitable
performance in a simple domain, experiments in more
complex domains such as maze navigation or sort-
ing networks (Cartlidge and Ait-Boudaoud, 2011) are
necessary to demonstrate its reliability. Furthermore,
a robust comparison against other state-of-art tech-
niques will be performed. Finally, we intend to ex-
plore the effects that SF has on other coevolutionary
pathologies, such as overspecialisation and cycling.
ACKNOWLEDGEMENTS
Hugo Alcaraz-Herrera’s PhD is supported by The
Mexican Council of Science and Technology (Con-
sejo Nacional de Ciencia y Tecnolog
´
ıa - CONACyT).
John Cartlidge is sponsored by Refinitiv.
REFERENCES
Akinola, A. and Wineberg, M. (2020). Using implicit multi-
objectives properties to mitigate against forgetfulness
in coevolutionary algorithms. In Genetic and Evo-
lutionary Computation Conference, GECCO, pages
769–777.
Bari, A. G., Gaspar, A., Wiegand, R. P., and Bucci, A.
(2018). Selection methods to relax strict acceptance
condition in test-based coevolution. In Congress on
Evolutionary Computation, CEC, pages 1–8.
Bullock, S., Cartlidge, J., and Thompson, M. (2002).
Prospects for computational steering of evolutionary
computation. In Workshop Proceedings 8th Int. Conf.
on Artificial Life, ALIFE, pages 131–137.
Cartlidge, J. and Ait-Boudaoud, D. (2011). Autonomous
virulence adaptation improves coevolutionary opti-
mization. IEEE Transactions on Evolutionary Com-
putation, 15(2):215–229.
Cartlidge, J. and Bullock, S. (2002). Learning lessons from
the common cold: How reducing parasite virulence
improves coevolutionary optimization. In Congress
on Evolutionary Computation, CEC, pages 1420–
1425 vol.2.
Cartlidge, J. and Bullock, S. (2003). Caring versus shar-
ing: How to maintain engagement and diversity in
coevolving populations. In Banzhaf, W., Ziegler, J.,
Christaller, T., Dittrich, P., and Kim, J. T., editors, Ad-
vances in Artificial Life, pages 299–308, Berlin, Hei-
delberg. Springer Berlin Heidelberg.
Cartlidge, J. and Bullock, S. (2004a). Combating coevolu-
tionary disengagement by reducing parasite virulence.
Evolutionary Computation, 12(2):193–222.
Cartlidge, J. and Bullock, S. (2004b). Unpicking tartan
CIAO plots: Understanding irregular coevolutionary
cycling. Adaptive Behavior, 12(2):69–92.
de Jong, E. D. (2007). A monotonic archive for pareto-
coevolution. Evolutionary Computation, 15(1):61–93.
Ficici, S. G. and Pollack, J. B. (1998). Challenges in
coevolutionary learning: Arms-race dynamics, open-
endedness, and mediocre stable states. In Interna-
tional Conference on Artificial Life, ALIFE, pages
238–247.
Garcia, D., Lugo, A. E., Hemberg, E., and O’Reilly, U.-
M. (2017). Investigating coevolutionary archive based
genetic algorithms on cyber defense networks. In
Genetic and Evolutionary Computation Conference
Companion, GECCO, pages 1455–1462.
Hillis, W. D. (1990). Co-evolving parasites improve simu-
lated evolution as an optimization procedure. Phys. D,
42(1–3):228–234.
Miguel Antonio, L. and Coello Coello, C. A. (2018). Co-
evolutionary multiobjective evolutionary algorithms:
Survey of the state-of-the-art. IEEE Transactions on
Evolutionary Computation, 22(6):851–865.
Pagie, L. and Mitchel, M. (2002). A comparison of evo-
lutionary and coevolutionary search. International
Journal of Computational Intelligence and Applica-
tion, 2(1):53–59.
Popovici, E., Bucci, A., Wiegand, R. P., and De Jong, E. D.
(2012). Coevolutionary principles. In Handbook of
Natural Computing, pages 987–1033. Springer Berlin
Heidelberg, Berlin, Heidelberg.
Rosin, C. D. (1997). Coevolutionary Search Among Adver-
saries. PhD thesis, Department of Computer Science,
University of California, San Diego, California.
Rosin, C. D. and Belew, R. K. (1997). New methods for
competitive coevolution. Evolutionary Computation,
5(1):1–29.
Simione, L. and Nolfi, S. (2021). Long-term progress and
behavior complexification in competitive coevolution.
Artificial Life, 26(4):409–430.
Watson, R. A. and Pollack, J. B. (2001). Coevolutionary
dynamics in a minimal substrate. In Genetic and Evo-
lutionary Computation Conference, GECCO, pages
702–709.
Wiegand, R. P. and Sarma, J. (2004). Spatial embedding
and loss of gradient in cooperative coevolutionary al-
gorithms. In Parallel Problem Solving from Nature,
PPSN, pages 912–921.
Williams, N. and Mitchell, M. (2005). Investigating the suc-
cess of spatial coevolution. In Genetic and Evolution-
ary Computation Conference, GECCO, pages 523–
530.
Substitution of the Fittest: A Novel Approach for Mitigating Disengagement in Coevolutionary Genetic Algorithms
67