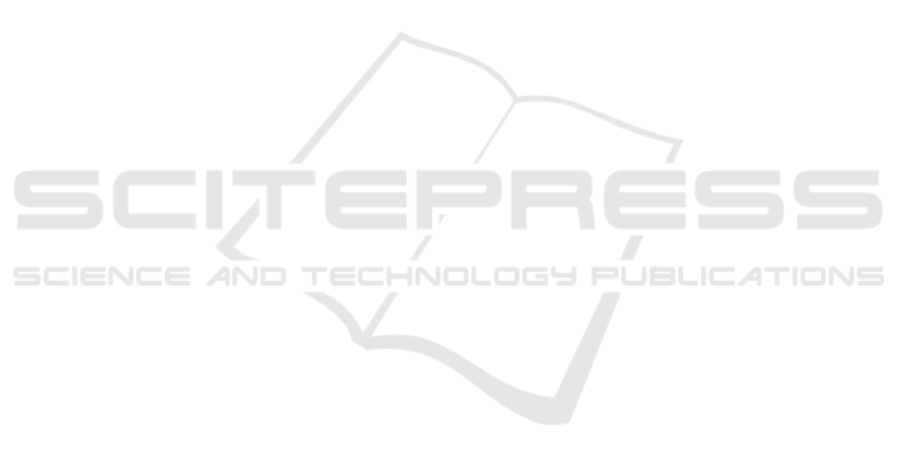
Jose, S., Malathi, D., Reddy, B., and Jayaseeli, D. (2018). A
survey on anomaly based host intrusion detection sys-
tem. In Journal of Physics: Conference Series, vol-
ume 1000, page 012049. IOP Publishing.
Kanimozhi, V. and Jacob, T. P. (2019). Artificial intelli-
gence based network intrusion detection with hyper-
parameter optimization tuning on the realistic cy-
ber dataset cse-cic-ids2018 using cloud computing.
In 2019 International Conference on Communication
and Signal Processing (ICCSP), pages 0033–0036.
IEEE.
Khraisat, A., Gondal, I., Vamplew, P., and Kamruzzaman,
J. (2019). Survey of intrusion detection systems:
techniques, datasets and challenges. Cybersecurity,
2(1):20.
Latha, S. and Prakash, S. J. (2017). A survey on network at-
tacks and intrusion detection systems. In 2017 4th In-
ternational Conference on Advanced Computing and
Communication Systems (ICACCS), pages 1–7. IEEE.
Le, T.-T.-H., Kim, Y., Kim, H., et al. (2019). Network intru-
sion detection based on novel feature selection model
and various recurrent neural networks. Applied Sci-
ences, 9(7):1392.
Li, Y. and Lu, Y. (2019). Lstm-ba: Ddos detection approach
combining lstm and bayes. In 2019 Seventh Interna-
tional Conference on Advanced Cloud and Big Data
(CBD), pages 180–185. IEEE.
Li, Z., Das, A., and Zhou, J. (2005). Usaid: Unifying
signature-based and anomaly-based intrusion detec-
tion. In Pacific-Asia Conference on Knowledge Dis-
covery and Data Mining, pages 702–712. Springer.
Liao, H.-J., Lin, C.-H. R., Lin, Y.-C., and Tung, K.-Y.
(2013). Intrusion detection system: A comprehensive
review. Journal of Network and Computer Applica-
tions, 36(1):16–24.
Longo, R., Podda, A. S., and Saia, R. (2020). Analysis of a
consensus protocol for extending consistent subchains
on the bitcoin blockchain. Computation, 8(3):67.
Luker, M. A. and Petersen, R. J. (2003). Computer and
network security in higher education. Jossey-Bass San
Francisco, CA.
Mazini, M., Shirazi, B., and Mahdavi, I. (2019). Anomaly
network-based intrusion detection system using a re-
liable hybrid artificial bee colony and adaboost algo-
rithms. Journal of King Saud University-Computer
and Information Sciences, 31(4):541–553.
Meyer, M. L. B. and Labit, Y. (2020). Combining machine
learning and behavior analysis techniques for network
security. In 2020 International Conference on Infor-
mation Networking (ICOIN), pages 580–583. IEEE.
Modi, C., Patel, D., Borisaniya, B., Patel, H., Patel, A., and
Rajarajan, M. (2013). A survey of intrusion detection
techniques in cloud. Journal of network and computer
applications, 36(1):42–57.
Munaiah, N., Meneely, A., Wilson, R., and Short, B. (2016).
Are intrusion detection studies evaluated consistently?
a systematic literature review.
Potluri, S. and Diedrich, C. (2016). High performance in-
trusion detection and prevention systems: A survey. In
ECCWS2016-Proceedings fo the 15th European Con-
ference on Cyber Warfare and Security, page 260.
Academic Conferences and publishing limited.
Radhakrishnan, K., Menon, R. R., and Nath, H. V. (2019).
A survey of zero-day malware attacks and its detection
methodology. In TENCON 2019-2019 IEEE Region
10 Conference (TENCON), pages 533–539. IEEE.
Rapanta, C., Botturi, L., Goodyear, P., Gu
`
ardia, L., and
Koole, M. (2020). Online university teaching during
and after the covid-19 crisis: Refocusing teacher pres-
ence and learning activity. Postdigital Science and Ed-
ucation, 2(3):923–945.
Rehman, R., Hazarika, G., and Chetia, G. (2011). Malware
threats and mitigation strategies: a survey. Journal
of Theoretical and Applied Information Technology,
29(2):69–73.
Saia, R. (2017). A discrete wavelet transform approach to
fraud detection. In International Conference on Net-
work and System Security, pages 464–474. Springer.
Saia, R. and Carta, S. (2016). A linear-dependence-based
approach to design proactive credit scoring models. In
KDIR, pages 111–120.
Saia, R. and Carta, S. (2017a). Evaluating credit card trans-
actions in the frequency domain for a proactive fraud
detection approach. In SECRYPT, pages 335–342.
Saia, R. and Carta, S. (2017b). A fourier spectral pattern
analysis to design credit scoring models. In Proceed-
ings of the 1st International Conference on Internet of
Things and Machine Learning, page 18. ACM.
Saia, R., Carta, S., et al. (2017). A frequency-domain-
based pattern mining for credit card fraud detection.
In IoTBDS, pages 386–391.
Saia, R., Carta, S., and Fenu, G. (2018a). A wavelet-based
data analysis to credit scoring. In Proceedings of the
2nd International Conference on Digital Signal Pro-
cessing, pages 176–180. ACM.
Saia, R., Carta, S., and Recupero, D. R. (2018b). A
probabilistic-driven ensemble approach to perform
event classification in intrusion detection system. In
KDIR, pages 139–146.
Saia, R., Carta, S., Recupero, D. R., and Fenu, G. (2020).
A feature space transformation to intrusion detection
systems. In KDIR. ScitePress.
Saia, R., Carta, S., Recupero, D. R., Fenu, G., and Stan-
ciu, M. (2019). A discretized extended feature space
(defs) model to improve the anomaly detection per-
formance in network intrusion detection systems. In
KDIR, pages 322–329.
Salahdine, F. and Kaabouch, N. (2019). Social engineering
attacks: A survey. Future Internet, 11(4):89.
Samrin, R. and Vasumathi, D. (2017). Review on anomaly
based network intrusion detection system. In 2017
International Conference on Electrical, Electronics,
Communication, Computer, and Optimization Tech-
niques (ICEECCOT), pages 141–147. IEEE.
Tidjon, L. N., Frappier, M., and Mammar, A. (2019).
Intrusion detection systems: A cross-domain
overview. IEEE Communications Surveys & Tutori-
als, 21(4):3639–3681.
Decomposing Training Data to Improve Network Intrusion Detection Performance
247