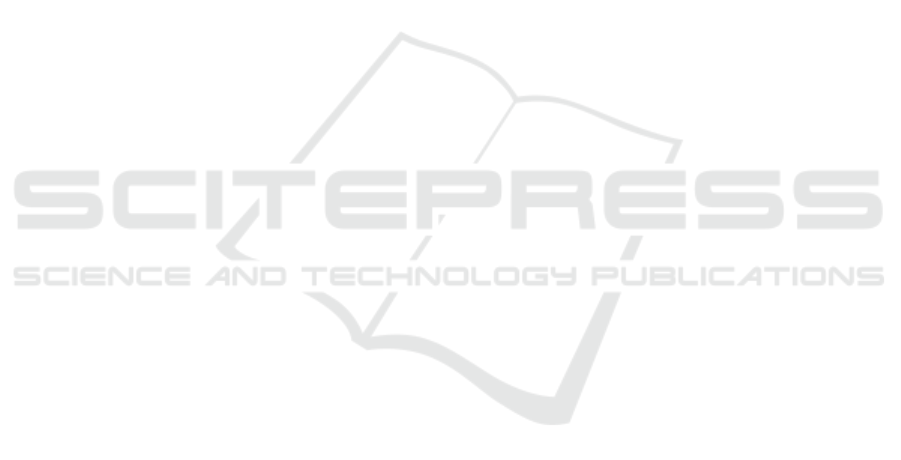
IEEE Congress on Evolutionary Computation (CEC),
pages 1–8. IEEE.
Del Vescovo, G. and Rizzi, A. (2007a). Automatic classi-
fication of graphs by symbolic histograms. In 2007
IEEE International Conference on Granular Comput-
ing (GRC 2007), pages 410–410.
Del Vescovo, G. and Rizzi, A. (2007b). Online handwrit-
ing recognition by the symbolic histograms approach.
In 2007 IEEE International Conference on Granular
Computing (GRC 2007), pages 686–686. IEEE.
Di Noia, A., Martino, A., Montanari, P., and Rizzi, A.
(2020). Supervised machine learning techniques and
genetic optimization for occupational diseases risk
prediction. Soft Computing, 24(6):4393–4406.
Ding, W., Lin, C.-T., Chen, S., Zhang, X., and Hu, B.
(2018). Multiagent-consensus-mapreduce-based at-
tribute reduction using co-evolutionary quantum pso
for big data applications. Neurocomputing, 272:136–
153.
Dorri, A., Kanhere, S. S., and Jurdak, R. (2018). Multi-
agent systems: A survey. Ieee Access, 6:28573–
28593.
Giampieri, M., Baldini, L., De Santis, E., and Rizzi, A.
(2020). Facing big data by an agent-based multi-
modal evolutionary approach to classification. In 2020
International Joint Conference on Neural Networks
(IJCNN), pages 1–8.
Giampieri, M., De Santis, E., Rizzi, A., and Frattale Masci-
oli, F. M. (2018). A supervised classification system
based on evolutive multi-agent clustering for smart
grids faults prediction. In 2018 International Joint
Conference on Neural Networks (IJCNN), pages 1–8.
González-Briones, A., Villarrubia, G., De Paz, J. F., and
Corchado, J. M. (2018). A multi-agent system for the
classification of gender and age from images. Com-
puter Vision and Image Understanding, 172:98–106.
˙
Inkaya, T., Kayalıgil, S., and Özdemirel, N. E. (2015).
Ant colony optimization based clustering methodol-
ogy. Applied Soft Computing, 28:301–311.
Lombardo, G., Fornacciari, P., Mordonini, M., Tomaiuolo,
M., and Poggi, A. (2019). A multi-agent architecture
for data analysis. Future Internet, 11(2):49.
Martino, A., Giampieri, M., Luzi, M., and Rizzi, A.
(2019a). Data mining by evolving agents for clus-
ters discovery and metric learning. In Esposito, A.,
Faundez-Zanuy, M., Morabito, F. C., and Pasero, E.,
editors, Neural Advances in Processing Nonlinear Dy-
namic Signals, pages 23–35. Springer International
Publishing, Cham. Italian Workshop on Neural Nets
2017.
Martino, A. and Rizzi, A. (2021). An enhanced filtering-
based information granulation procedure for graph
embedding and classification. IEEE Access, 9:15426–
15440.
Martino, A., Rizzi, A., and Frattale Mascioli, F. M.
(2019b). Efficient approaches for solving the large-
scale k-medoids problem: Towards structured data.
In Sabourin, C., Merelo, J. J., Madani, K., and War-
wick, K., editors, Computational Intelligence: 9th In-
ternational Joint Conference, IJCCI 2017 Funchal-
Madeira, Portugal, November 1-3, 2017 Revised Se-
lected Papers, pages 199–219. Springer International
Publishing, Cham.
Merelo, J. J., Chelly, Z., Mora, A., Fernández-Ares, A.,
Esparcia-Alcázar, A. I., Cotta, C., de las Cuevas, P.,
and Rico, N. (2016). A statistical approach to deal-
ing with noisy fitness in evolutionary algorithms. In
Merelo, J. J., Rosa, A., Cadenas, J. M., Dourado, A.,
Madani, K., and Filipe, J., editors, Computational In-
telligence, pages 79–95. Springer International Pub-
lishing, Cham.
Modi, P. J. and Shen, W.-M. (2001). Collaborative multia-
gent learning for classification tasks. In Proceedings
of the Fifth International Conference on Autonomous
Agents, AGENTS ’01, page 37–38, New York, NY,
USA. Association for Computing Machinery.
Mostafa, J., Ke, W., and Fu, Y. (2005). Automated text
classification using a multi-agent framework. In Pro-
ceedings of the 5th ACM/IEEE-CS Joint Conference
on Digital Libraries (JCDL ’05), pages 157–158.
Ogston, E., Overeinder, B., Van Steen, M., and Brazier, F.
(2003). A method for decentralized clustering in large
multi-agent systems. In Proceedings of the second
international joint conference on Autonomous agents
and multiagent systems, pages 789–796. ACM.
Pan, X. and Chen, H. (2012). Multi-agent evolutionary
clustering algorithm based on manifold distance. In
Computational Intelligence and Security (CIS), 2012
Eighth International Conference on, pages 123–127.
IEEE.
Pan, X. and Jiao, L. (2011). A granular agent evolutionary
algorithm for classification. Applied Soft Computing,
11(3):3093–3105.
Panait, L. and Luke, S. (2005). Cooperative multi-agent
learning: The state of the art. Autonomous Agents and
Multi-Agent Systems, 11(3):387–434.
Park, J. and Oh, K. (2006). Multi-agent systems for intel-
ligent clustering. In Proc. of World Academy of Sci-
ence, Engineering and Technology, volume 11, pages
97–102.
Preuss, M. (2015). Multimodal optimization by means of
evolutionary algorithms. Springer, 1 edition.
Riesen, K. and Bunke, H. (2008). Iam graph database
repository for graph based pattern recognition and ma-
chine learning. In Joint IAPR International Work-
shops on Statistical Techniques in Pattern Recognition
(SPR) and Structural and Syntactic Pattern Recogni-
tion (SSPR), pages 287–297. Springer.
Rizk, Y., Awad, M., and Tunstel, E. W. (2018). Deci-
sion making in multiagent systems: A survey. IEEE
Transactions on Cognitive and Developmental Sys-
tems, 10(3):514–529.
Stone, P. and Veloso, M. (2000). Multiagent systems: A sur-
vey from a machine learning perspective. Autonomous
Robots, 8(3):345–383.
Theodoridis, S. and Koutroumbas, K. (2008). Pattern
Recognition. Academic Press, 4 edition.
Wong, K. (2015). Evolutionary multimodal optimization:
A short survey. CoRR, abs/1508.00457.
A Multi-agent Approach for Graph Classification
343