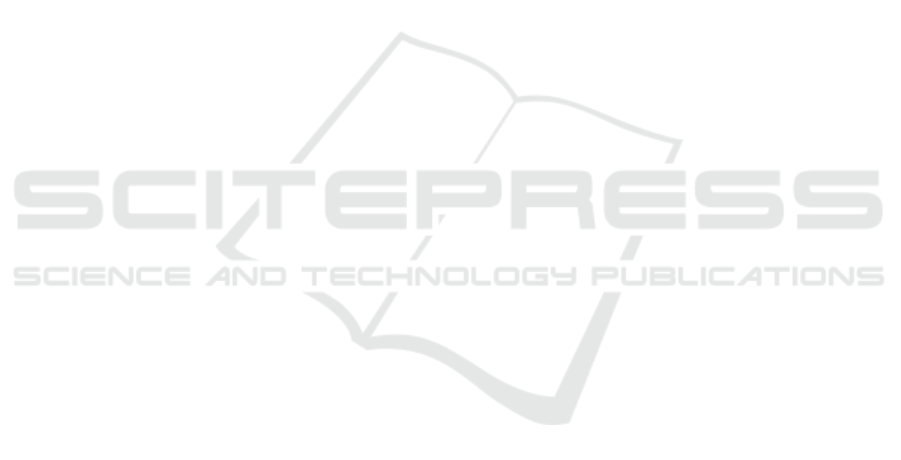
Silver Coast (LIFE16 ENV/IT/000337). Such a help
is crucial to achieve natural and historical preserva-
tion of Italy, especially of touristic areas.
REFERENCES
Aguercif, T., Tighzert, L., Mendil, B., and Fonlupt, C.
(2017). Rate learning-based fish school search al-
gorithm for global optimization. In 2017 6th Inter-
national Conference on Systems and Control (ICSC),
pages 520–525.
Akiyama, T., Kobayashi, Y., Kishigami, J., and Muto, K.
(2018). Cnn-based boat detection model for alert
system using surveillance video camera. In 2018
IEEE 7th Global Conference on Consumer Electron-
ics (GCCE), pages 669–670.
Bianchi, F. M., De Santis, E., Rizzi, A., and Sadeghian,
A. (2015). Short-term electric load forecasting using
echo state networks and pca decomposition. Ieee Ac-
cess, 3:1931–1943.
Bojarski, M., Testa, D., Dworakowski, D., Firner, B., Flepp,
B., Goyal, P., Jackel, L., Monfort, M., Muller, U.,
Zhang, J., Zhang, X., Zhao, J., and Zieba, K. (2016).
End to end learning for self-driving cars. ArXiv,
abs/1604.07316.
Boujelben, M., Ayedi, D., Rekik, C., and Derbel, N. (2017).
Fuzzy logic controller for mobile robot navigation to
avoid dynamic and static obstacles. In 2017 14th In-
ternational Multi-Conference on Systems, Signals De-
vices (SSD), pages 293–298.
Boujelben, M., Rekik, C., and Derbel, N. (2013). Hierar-
chical fuzzy controller to avoid mobile obstacle for a
mobile robot. In 10th International Multi-Conferences
on Systems, Signals Devices 2013 (SSD13), pages 1–
8.
Brehmer, P., Sarr
´
e, A., Gonzalez, L., Cotel, P., Hermand, J.-
P., and Franc¸ois, G. (2013). Aggregative and school-
ing behaviour of small pelagic fish schools through
echo type characteristics. In 2013 IEEE/OES Acous-
tics in Underwater Geosciences Symposium, pages 1–
6.
Cai, L. and Sun, Q. (2017). A regular expression group-
ing algorithm based on artificial fish school algorithm.
In 2017 7th IEEE International Conference on Elec-
tronics Information and Emergency Communication
(ICEIEC), pages 556–559.
Cherroun, L. and Boumehraz, M. (2012). Intelligent sys-
tems based on reinforcement learning and fuzzy logic
approaches, ”application to mobile robotic”. In 2012
International Conference on Information Technology
and e-Services, pages 1–6.
Chu, J., Gu, W., and Chen, X. (2008). Study on adaptive
control of the propelling and turning manoeuvre of an
autonomous water vehicle for ocean observation. In
OCEANS 2008, pages 1–4.
De Santis, E., Livi, L., Sadeghian, A., and Rizzi, A. (2015).
Modeling and recognition of smart grid faults by a
combined approach of dissimilarity learning and one-
class classification. Neurocomputing, 170:368–383.
De Santis, E., Paschero, M., Rizzi, A., and Masci-
oli, F. M. F. (2018). Evolutionary optimization of
an affine model for vulnerability characterization in
smart grids. In 2018 International Joint Conference
on Neural Networks (IJCNN), pages 1–8. IEEE.
De Santis, E., Rizzi, A., Sadeghiany, A., and Mascioli, F.
M. F. (2013). Genetic optimization of a fuzzy control
system for energy flow management in micro-grids. In
2013 Joint IFSA World Congress and NAFIPS Annual
Meeting (IFSA/NAFIPS), pages 418–423. IEEE.
De Santis, E., Sadeghian, A., and Rizzi, A. (2017). A
smoothing technique for the multifractal analysis of a
medium voltage feeders electric current. International
Journal of Bifurcation and Chaos, 27(14):1750211.
Duan, Y. and Xin-Hexu (2005). Fuzzy reinforcement learn-
ing and its application in robot navigation. In 2005
International Conference on Machine Learning and
Cybernetics, volume 2, pages 899–904 Vol. 2.
Elkins, L., Sellers, D., and Monach, W. R. (2010). The
autonomous maritime navigation (amn) project: Field
tests, autonomous and cooperative behaviors, data fu-
sion, sensors, and vehicles. Journal of Field Robotics,
27(6):790–818.
Ferrandino., E., Capillo., A., Mascioli., F., and Rizzi., A.
(2020). Nanogrids: A smart way to integrate public
transportation electric vehicles into smart grids. Pro-
ceedings of the 12th International Joint Conference
on Computational Intelligence - CI4EMS,, pages 512–
520.
Fossen, T. I. (2021). Handbook of Marine Craft Hydrody-
namics and Motion Control. Wiley.
Fujiwara, C., Onishi, N., Tateyama, T., Hiratsuka, S.,
Sakakibara, K., and Chen, Y.-W. (2012). Behav-
ior generation of fish school by the potential field
method in virtual aqua museum. In 2012 6th Interna-
tional Conference on New Trends in Information Sci-
ence, Service Science and Data Mining (ISSDM2012),
pages 495–498.
Glorennec, P. Y. (1996). Fuzzy logic-based navigation for
an autonomous robot. IFAC Proceedings Volumes,
29(4):45–49. IFAC Workshop on Intelligent compo-
nents for Autonomous and Semi-Autonomous Vehi-
cles, Toulouse, France, 25-26 October.
Grigorescu, S., Trasnea, B., Cocias, T., and Macesanu,
G. (2020). A survey of deep learning techniques
for autonomous driving. Journal of Field Robotics,
37(3):362–386.
He, K., Sun, H., and Cheng, W. (2008). Application of
fuzzy neural network based on t-s model for mobile
robot to avoid obstacles. 2008 7th World Congress
on Intelligent Control and Automation, pages 8282–
8285.
Hong, J., Tang, K., and Chen, C. (2017). Obstacle avoid-
ance of hexapod robots using fuzzy q-learning. In
2017 IEEE Symposium Series on Computational In-
telligence (SSCI), pages 1–6.
Kobayashi, E.-i., Yoneda, S., and Morita, A. (2014). Ad-
vanced route optimization in ship navigation. In
Proceedings of the 4th International Conference on
Simulation and Modeling Methodologies, Technolo-
gies and Applications, page 572–577, Setubal, PRT.
FCTA 2021 - 13th International Conference on Fuzzy Computation Theory and Applications
194