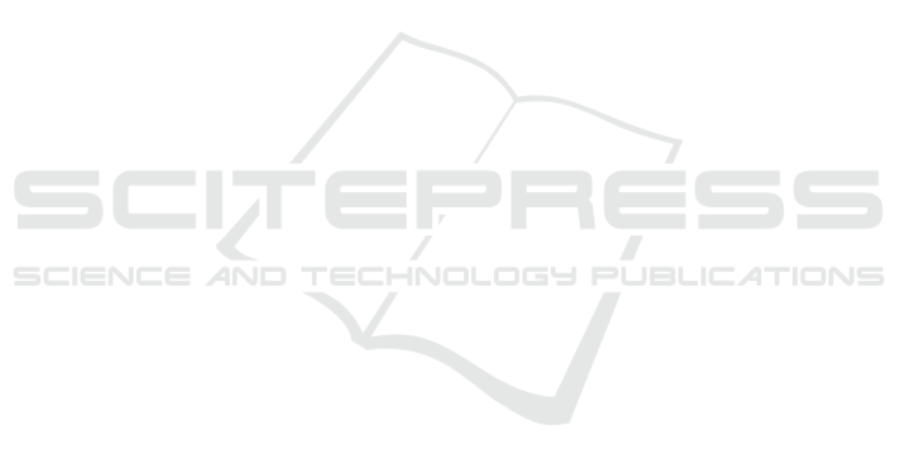
plementation only allows for a single source of truth
for data input which is a limitation for RDM systems
where the datasets are scattered in multiple locations.
The seen complex process model shown in figure 4b
results from actual user behavior in an environment
where users are free to interact with a system with-
out a specific order. Thus, there is a need to extend
DA4RDM with a pre-processing pipeline suggested
by the authors in (Yazdi et al., 2021) to abstract event
logs to achieve structured and simple process mod-
els. We have to acknowledge that Coscine is a de-
veloping RDM platform and is currently in its pilot
phase; hence the sample dataset available is limited
to its beta users, and the current preliminary findings
may not entirely reflect the actual user behavior in a
mature RDM system.
The future work includes adding additional in-
terfaces for conformance checking of the user pro-
cess, identifying unfinished user journeys and trig-
gering automatic actions, and extending the collected
dataset to produce a FAIR maturity dashboard for ev-
ery research project and suggest user actions to in-
crease research data FAIRness. Although the current
DA4RDM UI allows for a PI to interact with the sys-
tem, the post-processing of data models proved to be
too advanced; therefore, we may need to pre-scope
the available features of our web application for the
target audience.
5 CONCLUSION
In this paper, we discussed the benefits of DA4RDM
for an RDM system. We began with a technical re-
view of the developed web application, its charac-
teristics, and functionalities. Furthermore, we elab-
orated on an RDM platform (Coscine) as a candidate
system under study and the approach used to obtain
a real dataset for the goal of data modeling and anal-
ysis. Preliminary findings demonstrated the benefits
of such a system toward user behavior studies and
discovering non-functional requirements. Although
the extracted data from Coscine is entirely based on
a service layer, DA4RDM is showing to be adapt-
able to any log format according to the needs of a
data modeling algorithm. Therefore, the contributions
of DA4RDM are to allow for a scalable web appli-
cation that enables non-technical staff to reuse pre-
defined pre-and post-processing pipelines to execute
data-driven studies without technical or scientific ex-
pertise.
REFERENCES
Berti, A., van Zelst, S. J., and van der Aalst, W. (2019).
Process mining for python (pm4py): bridging the gap
between process-and data science. arXiv preprint
arXiv:1905.06169.
Celik, U. and Akçetin, E. (2018). Process mining tools
comparison. Online Academic Journal of Information
Technology, 9:97–104.
Gargiulo, P., Galimberti, P., Tammaro, A. M., and Zane, A.
(2021). Fair rdm (research data management): Italian
initiatives towards eosc implementation. In IRCDL,
pages 42–52.
Kebede, M. and Dumas, M. (2015). Comparative evaluation
of process mining tools. University of Tartu.
Kindler, E., Rubin, V., and Schäfer, W. (2006). Process
mining and petri net synthesis. In International Con-
ference on Business Process Management, pages 105–
116. Springer.
Malkawi, R., Saifan, A. A., Alhendawi, N., and Bani-
Ismaeel, A. (2020). Data mining tools evaluation
based on their quality attributes. International Journal
of Advanced Science and Technology, 29(3):13867–
13890.
Politze, M., Claus, F., Brenger, B., Yazdi, M. A., Heinrichs,
B., and Schwarz, A. (2020). How to manage it re-
sources in research projects? towards a collaborative
scientific integration environment. European Journal
of Higher Education IT, 2.
Rafiei, M., von Waldthausen, L., and van der Aalst, W. M.
(2018). Ensuring confidentiality in process min-
ing. Proceedings of the 8th International Sympo-
sium on Data-driven Process Discovery and Analysis-
SIMPDA, 18:3–17.
van der Aalst, W. (2016). Process mining: data science in
action. Springer.
Wilkinson, M. D., Dumontier, M., Aalbersberg, I. J., Apple-
ton, G., Axton, M., Baak, A., Blomberg, N., Boiten,
J.-W., da Silva Santos, L. B., Bourne, P. E., et al.
(2016). The fair guiding principles for scientific data
management and stewardship. Scientific data, 3(1):1–
9.
Yazdi, M. A. (2019). Enabling operational support in the
research data life cycle. In Proceedings of the First
International Conference on Process Mining, pages
1–10.
Yazdi, M. A., Farhadi, P., and Heinrichs, B. (2021). Event
log abstraction in client-server applications. In IC3K
2021: Proceedings of the 13th International Joint
Conference on Knowledge Discovery, Knowledge
Engineering and Knowledge Management: KDIR.
SciTePress.
Yazdi, M. A. and Politze, M. (2020). Reverse engineering:
The university distributed services. In Proceedings of
the Future Technologies Conference, pages 223–238.
Springer.
DA4RDM: Data Analysis for Research Data Management Systems
183