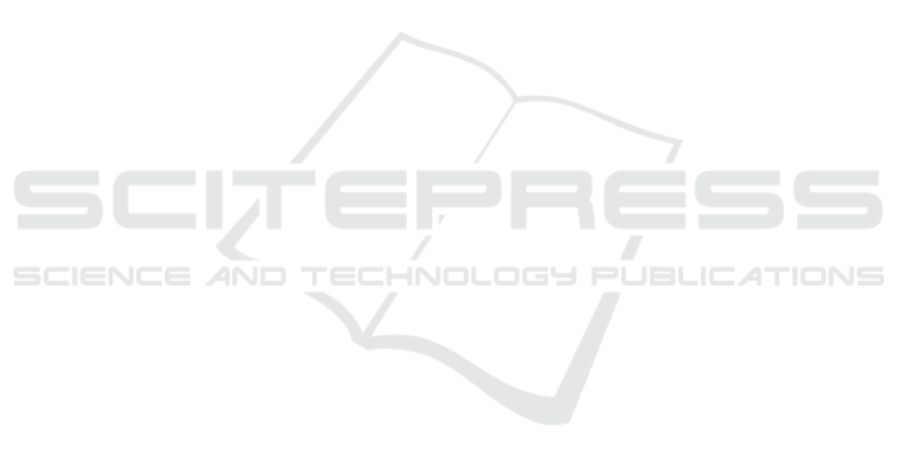
ACKNOWLEDGEMENTS
This work has received funding from Basque Govern-
ment under project AUTOLIB of the program ELKA-
RTEK 2019.
REFERENCES
Aranjuelo, N., Engels, G., Unzueta, L., Arganda-Carreras,
I., Nieto, M., and Otaegui, O. (2020). Robust 3d ob-
ject detection from lidar point cloud data with spatial
information aggregation. In International Workshop
on Soft Computing Models in Industrial and Environ-
mental Applications, pages 813–823. Springer.
Chen, X., Ma, H., Wan, J., Li, B., and Xia, T. (2017). Multi-
view 3d object detection network for autonomous
driving. In Proceedings of the IEEE Conference
on Computer Vision and Pattern Recognition, pages
1907–1915.
Du, X., Lin, T.-Y., Jin, P., Ghiasi, G., Tan, M., Cui, Y., Le,
Q. V., and Song, X. (2020). SpineNet: Learning scale-
permuted backbone for recognition and localization.
In Proceedings of the IEEE/CVF conference on com-
puter vision and pattern recognition, pages 11592–
11601.
Engels, G., Aranjuelo, N., Arganda-Carreras, I., Nieto, M.,
and Otaegui, O. (2020). 3d object detection from li-
dar data using distance dependent feature extraction.
arXiv preprint arXiv:2003.00888.
Geiger, A., Lenz, P., and Urtasun, R. (2012). Are we ready
for autonomous driving? the KITTI vision benchmark
suite. In 2012 IEEE conference on computer vision
and pattern recognition, pages 3354–3361. IEEE.
He, K., Gkioxari, G., Doll
´
ar, P., and Girshick, R. (2017).
Mask r-cnn. In Proceedings of the IEEE international
conference on computer vision, pages 2961–2969.
He, K., Zhang, X., Ren, S., and Sun, J. (2016). Deep resid-
ual learning for image recognition. In Proceedings of
the IEEE conference on computer vision and pattern
recognition, pages 770–778.
Lang, A. H., Vora, S., Caesar, H., Zhou, L., Yang, J., and
Beijbom, O. (2019). Pointpillars: Fast encoders for
object detection from point clouds. In Proceedings
of the IEEE/CVF Conference on Computer Vision and
Pattern Recognition, pages 12697–12705.
Lin, T.-Y., Doll
´
ar, P., Girshick, R., He, K., Hariharan, B.,
and Belongie, S. (2017). Feature pyramid networks
for object detection. In Proceedings of the IEEE con-
ference on computer vision and pattern recognition,
pages 2117–2125.
Luo, W., Li, Y., Urtasun, R., and Zemel, R. (2016). Under-
standing the effective receptive field in deep convo-
lutional neural networks. In Proceedings of the 30th
International Conference on Neural Information Pro-
cessing Systems, pages 4905–4913.
NVIDIA (2021). TensorRT: A platform for high-
performance deep learning inference. https://
developer.nvidia.com/tensorrt. Accessed: 07.01.2021.
Qi, C. R., Liu, W., Wu, C., Su, H., and Guibas, L. J. (2018).
Frustum pointnets for 3d object detection from rgb-d
data. In Proceedings of the IEEE conference on com-
puter vision and pattern recognition, pages 918–927.
Qi, C. R., Su, H., Mo, K., and Guibas, L. J. (2017). Point-
net: Deep learning on point sets for 3d classification
and segmentation. In Proceedings of the IEEE con-
ference on computer vision and pattern recognition,
pages 652–660.
Qian, W., Yang, X., Peng, S., Guo, Y., and Yan, J. (2019).
Learning modulated loss for rotated object detection.
arXiv preprint arXiv:1911.08299.
Redmon, J. and Farhadi, A. (2018). Yolov3: An incremental
improvement. arXiv preprint arXiv:1804.02767.
Ren, S., He, K., Girshick, R., and Sun, J. (2015). Faster R-
CNN: Towards real-time object detection with region
proposal networks. Advances in neural information
processing systems, 28:91–99.
Shi, S., Wang, X., and Li, H. (2018). PointRCNN: 3d object
proposal generation and detection from point cloud.
CoRR, abs/1812.04244.
Simon, M., Amende, K., Kraus, A., Honer, J., Samann,
T., Kaulbersch, H., Milz, S., and Michael Gross, H.
(2019). Complexer-yolo: Real-time 3d object detec-
tion and tracking on semantic point clouds. In Pro-
ceedings of the IEEE/CVF Conference on Computer
Vision and Pattern Recognition Workshops, pages 0–
0.
Yan, Y., Mao, Y., and Li, B. (2018). Second:
Sparsely embedded convolutional detection. Sensors,
18(10):3337.
Yang, B., Luo, W., and Urtasun, R. (2018). Pixor: Real-time
3d object detection from point clouds. In Proceedings
of the IEEE conference on Computer Vision and Pat-
tern Recognition, pages 7652–7660.
Zhou, D., Fang, J., Song, X., Guan, C., Yin, J., Dai, Y., and
Yang, R. (2019a). Iou loss for 2d/3d object detection.
In 2019 International Conference on 3D Vision (3DV),
pages 85–94. IEEE.
Zhou, D., Fang, J., Song, X., Liu, L., Yin, J., Dai, Y., Li, H.,
and Yang, R. (2020). Joint 3d instance segmentation
and object detection for autonomous driving. In Pro-
ceedings of the IEEE/CVF Conference on Computer
Vision and Pattern Recognition, pages 1839–1849.
Zhou, J., Tan, X., Shao, Z., and Ma, L. (2019b). FVnet:
3d front-view proposal generation for real-time ob-
ject detection from point clouds. In 2019 12th In-
ternational Congress on Image and Signal Process-
ing, BioMedical Engineering and Informatics (CISP-
BMEI), pages 1–8. IEEE.
Zhou, Y. and Tuzel, O. (2018). Voxelnet: End-to-end learn-
ing for point cloud based 3d object detection. In Pro-
ceedings of the IEEE conference on computer vision
and pattern recognition, pages 4490–4499.
Accurate 3D Object Detection from Point Cloud Data using Bird’s Eye View Representations
253