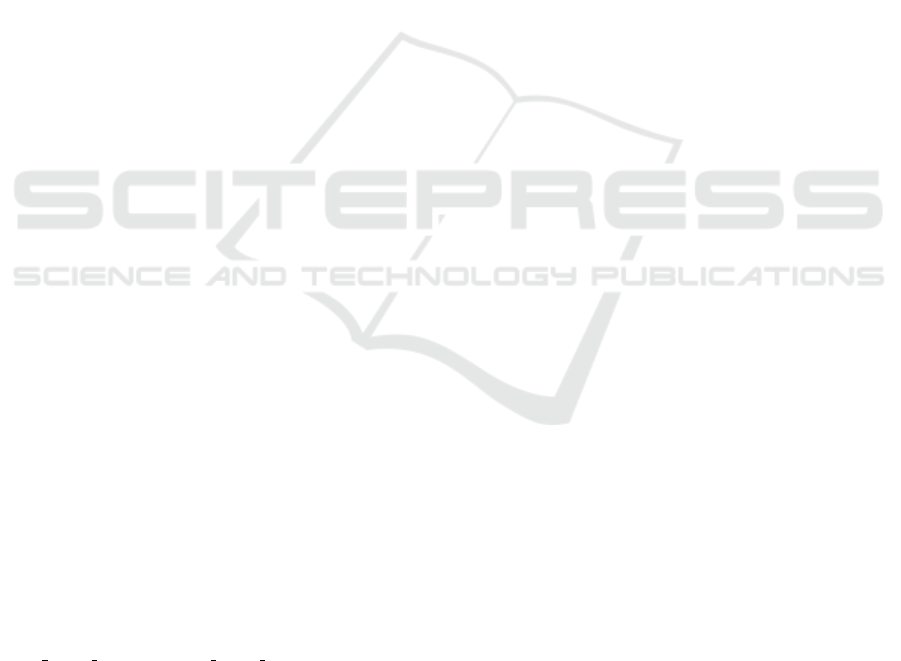
Gruber, T. R. (1993). A translation approach to portable
ontology specifications. Knowledge Acquisition.
Hargutt, V. and Kruger, H.-P. (2001). Eyelid movements
and their predictive value for fatigue stages.
Hartley, L., Horberry, T., Mabbott, N., and Krueger, G. P.
(2000). Review of fatigue detection and prediction
technologies. National Road Transport Commission.
Hina, M. D., Thierry, C., Soukane, A., and Ramdane-
Cherif, A. (2018). Cognition of Driving Context for
Driving Assistance. page 11.
Ji, Q., Zhu, Z., and Lan, P. (2004). Real-Time Nonintrusive
Monitoring and Prediction of Driver Fatigue. IEEE.
Jorion, P. (1989). Principes Des Systemes Intelligents.
Karray, F., Karray, F. O., and Silva, C. W. D. (2004). Soft
Computing and Intelligent Systems Design: Theory,
Tools, and Applications. Pearson Education.
Kasabov, N. and Filev, D. (2006). Evolving Intelligent Sys-
tems: Methods, Learning, & Applications. In Inter-
national Symposium on Evolving Fuzzy Systems, Am-
belside, UK. IEEE.
Krajewski, J., Sommer, D., Trutschel, U., Edwards, D., and
Golz, M. (2009). Steering Wheel Behavior Based Es-
timation of Fatigue. pages 118–124, Big Sky, Mon-
tana, USA. University of Iowa.
Kumar, D., Pah, N., and Bradley, A. (2003). Wavelet anal-
ysis of surface electromyography to determine muscle
fatigue. IEEE Trans. Neural Syst. Rehabil. Eng.
Li, Z., Chen, L., Peng, J., and Wu, Y. (2017a). Automatic
Detection of Driver Fatigue Using Driving Operation
Information for Transportation Safety. Sensors.
Li, Z., Li, S., Li, R., Cheng, B., and Shi, J. (2017b). On-
line Detection of Driver Fatigue Using Steering Wheel
Angles for Real Driving Conditions. Sensors.
Li, Z., Song, L., Yang, Q., Chen, S., and Chen, L. (2020).
Support vector machine filtering data aid on fatigue
driving detection.
Liu, C. C., Hosking, S. G., and Lenn
´
e, M. G. (2009). Pre-
dicting driver drowsiness using vehicle measures: Re-
cent insights and future challenges. Journal of Safety
Research, (4).
Mu, Z., Hu, J., and Min, J. (2017). Driver Fatigue Detection
System Using Electroencephalography Signals Based
on Combined Entropy Features. Applied Sciences.
Musen, M. A. (2015). The prot
´
eg
´
e project: a look back and
a look forward. AI Matters, 1(4):4–12.
Observatory, E. R. S. (2019). Annual Accident Report.
Technical report.
Organization., W. H. (2018). World Health Or-
ganization. (2018). Global Status Report on
Road Safety 2018. https://www.who.int/violence
injury prevention/road safety status/2018/en/.
Technical report.
Polychronopoulos, A., Amditis, A., and Bekiaris, E. (2004).
Information data flow in awake multi-sensor driver
monitoring system. In IEEE Intelligent Vehicles Sym-
posium, 2004, pages 902–906, Parma, Italy. IEEE.
Ramzan, M., Khan, H. U., Awan, S. M., Ismail, A., Ilyas,
M., and Mahmood, A. (2019). A Survey on State-of-
the-Art Drowsiness Detection Techniques. IEEE.
Reddy, B., Kim, Y.-H., Yun, S., Seo, C., and Jang, J. (2017).
Real-Time Driver Drowsiness Detection for Embed-
ded System Using Model Compression of Deep Neu-
ral Networks. Honolulu, HI, USA. IEEE.
Rigas, G., Katsis, C. D., Bougia, P., and Fotiadis, D. I.
(2008). A reasoning-based framework for car driver
and stress prediction. Ajaccio, France. IEEE.
Rondik, T. and Mautner, P. (2013). Cognitive event-related
potential waveform latency determination: Based on
result of matching pursuit algorithm and Hilbert-
Huang transform. Hangzhou, China. IEEE.
Roussey, C., Soulignac, V., Champomier, J. C., Abt, V., and
Chanet, J. P. (2010). Ontologies in Agriculture.
Salmon, P. M., Read, G. J., Beanland, V., Thompson, J.,
Filtness, A. J., Hulme, A., McClure, R., and John-
ston, I. (2019). Bad behaviour or societal failure? Per-
ceptions of the factors contributing to drivers’ engage-
ment in the fatal five driving behaviours.
Schleicher, R., Galley, N., Briest, S., and Galley, L. (2008).
Blinks and saccades as indicators of fatigue in sleepi-
ness warnings: looking tired? Ergonomics, 51.
Shinar, Z., Akselrod, S., Dagan, Y., and Baharav, A. (2006).
Autonomic changes during wake–sleep transition: A
heart rate variability based approach.
Siegmund, G. P., King, D. J., and Mumford, D. K. (1996).
Correlation of Steering Behavior with Heavy-Truck
Driver Fatigue.
Studer, R., Benjamins, V., and Fensel, D. (1998). Knowl-
edge engineering: Principles and methods. Data &
Knowledge Engineering.
Sun, Y., Yu, X., Berilla, J., and Liu, Z. (2011). An in-vehicle
physiological signal monitoring system for driver fa-
tigue detection. page 16.
Svensson, U. (2004). Blink behaviour based drowsiness de-
tection – method development and validation.
Thiffault, P. and Bergeron, J. (2003). Monotony of road en-
vironment and driver fatigue: a simulator study. Acci-
dent Analysis & Prevention, (3).
Thorslund, B. (2003). Electrooculogram analysis and de-
velopment of a system for defining stages of drowsi-
ness. PhD thesis.
Ting, P.-H., Hwang, J.-R., Doong, J.-L., and Jeng, M.-C.
(2008). Driver fatigue and highway driving: A simu-
lator study. Physiology & Behavior, 94(3).
Vicente, J., Laguna, P., Bartra, A., and Bail
´
on, R. (2016).
Drowsiness detection using heart rate variability. Med
Biol Eng Comput, (6).
Weiwei Liu, Haixin Sun, and Weijie Shen (2010). Driver
fatigue detection through pupil detection and yawing
analysis. Chengdu, China. IEEE.
Yue, C. (2011). EOG Signals in Drowsiness Research.
Zhao, C., Zhao, M., Liu, J., and Zheng, C. (2012). Elec-
troencephalogram and electrocardiograph assessment
of mental fatigue in a driving simulator.
Zhenhai, G., DinhDat, L., Hongyu, H., Ziwen, Y., and
Xinyu, W. (2017). Driver Drowsiness Detection Based
on Time Series Analysis of Steering Wheel Angular
Velocity. In (ICMTMA), Changsha, China. IEEE.
Modelling and Detection of Driver’s Fatigue using Ontology
95