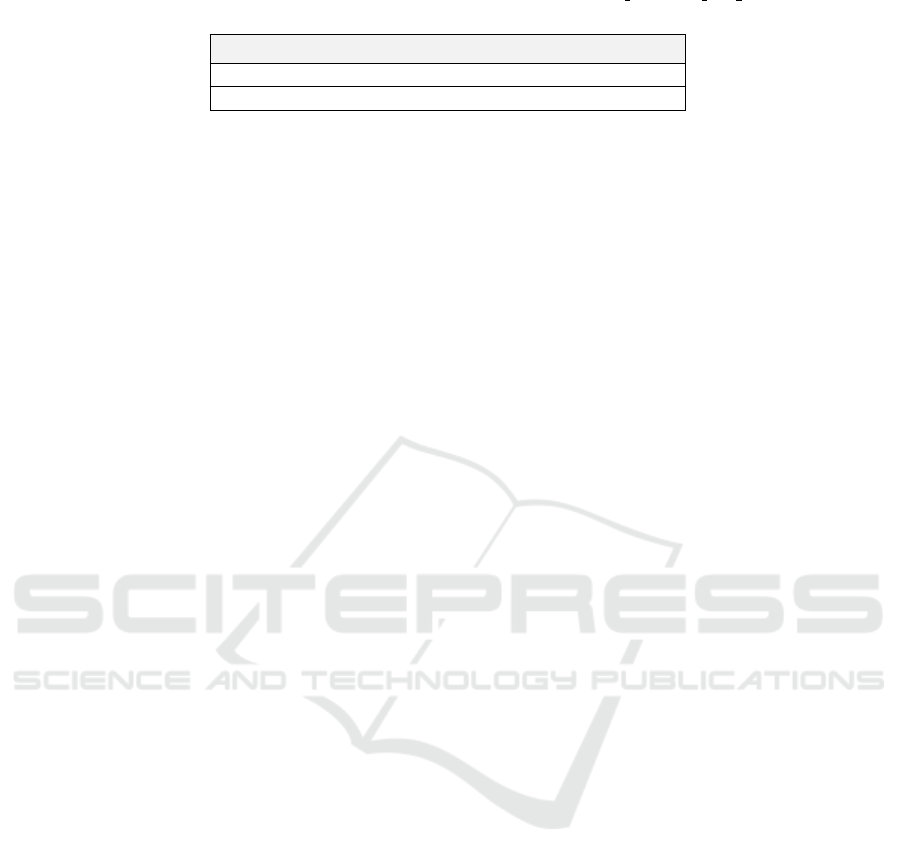
Table 4: Comparison of the metrics obtained for drug repositioning through the is substance that treats relation on the
OREGANO KG with the results obtained on Hetionet.
Data MRR Hits@1 Hits@3 Hits@10
OREGANO 0.4980 0.3898 0.5551 0.7300
HETIONET 0.4300 0.3370 0.4700 0.6410
5 CONCLUSION
In this paper, we have described a neuro-symbolic
XAI solution applied to the DR task using a KG. The
study has been implemented and evaluated in the con-
text of the OREGANO project using a state-of-the-art
algorithm which enables the explainability. Results
equivalent to those of the state of the art were obtained,
as well as several ways to provide an explanation for
each prediction, i.e. intrinsic to the model’s opera-
tion, but also post-hoc in a mechanistic way using fea-
tures of a graph oriented database. The challenges sur-
rounding this work are numerous. First, it is important
to better understand how the organization of data in a
KG affects the prediction task. This will be partic-
ularly important for the application of these methods
to DR for rare diseases, where data is by definition
less abundant. Also, more flexible methods must be
thought of, allowing biochemists and other clinicians
to easily participate in the learning process, bringing
their knowledge to the sum of data available for DR.
REFERENCES
Ashburn, T. and Thor, K. (2004). Drug repositioning: Identi-
fying and developing new uses for existing drugs. Na-
ture reviews Drug discovery, 3:673–83.
Bordes, A., Usunier, N., Garc
´
ıa-Dur
´
an, A., Weston, J., and
Yakhnenko, O. (2013). Translating embeddings for
modeling multi-relational data. In Advances in Neu-
ral Information Processing Systems 26.
Boudin, M. (2020). Computational approaches for drug
repositioning: Towards a holistic perspective based on
knowledge graphs. Proceedings of the 29th ACM In-
ternational Conference on Information & Knowledge
Management, page 3225–3228.
Das, R., Dhuliawala, S., Zaheer, M., Vilnis, L., Durugkar,
I., Krishnamurthy, A., Smola, A., and McCallum, A.
(2018). Go for a walk and arrive at the answer: Rea-
soning over paths in knowledge bases using reinforce-
ment learning. In International Conference on Learn-
ing Representations.
DiMasi, J. A., Grabowski, H. G., and Hansen, R. W. (2016).
Innovation in the pharmaceutical industry: New es-
timates of r&d costs. Journal of Health Economics,
47:20–33.
Himmelstein, D. S., Lizee, A., Hessler, C., Brueggeman, L.,
Chen, S. L., Hadley, D., Green, A., Khankhanian, P.,
and Baranzini, S. E. (2017). Systematic integration of
biomedical knowledge prioritizes drugs for repurpos-
ing. eLife, 6:e26726.
Hochreiter, S. and Schmidhuber, J. (1997). Long Short-
Term Memory. Neural Computation, 9(8):1735–1780.
Liu, Y., Hildebrandt, M., Joblin, M., Ringsquandl, M., Rais-
souni, R., and Tresp, V. (2021). Neural multi-hop
reasoning with logical rules on biomedical knowledge
graphs. In Verborgh, R., Hose, K., Paulheim, H.,
Champin, P.-A., Maleshkova, M., Corcho, O., Ris-
toski, P., and Alam, M., editors, The Semantic Web,
pages 375–391, Cham. Springer International Publish-
ing.
Meilicke, C., Chekol, M. W., Ruffinelli, D., and Stucken-
schmidt, H. (2019). Anytime bottom-up rule learning
for knowledge graph completion. In IJCAI.
Paulheim, H. (2017). Knowledge graph refinement: A sur-
vey of approaches and evaluation methods. Semantic
Web, 8:489–508.
Stokes, J. M., Yang, K., Swanson, K., Jin, W., Cubillos-
Ruiz, A., Donghia, N. M., MacNair, C. R., French, S.,
Carfrae, L. A., Bloom-Ackermann, Z., Tran, V. M.,
Chiappino-Pepe, A., Badran, A. H., Andrews, I. W.,
Chory, E. J., Church, G. M., Brown, E. D., Jaakkola,
T. S., Barzilay, R., and Collins, J. J. (2020). A
deep learning approach to antibiotic discovery. Cell,
180(4):688–702.e13.
Zhang, R., Hristovski, D., Schutte, D., Kastrin, A., Fiszman,
M., and Kilicoglu, H. (2021). Drug repurposing for
covid-19 via knowledge graph completion. Journal of
Biomedical Informatics, 115:103696.
Neuro-symbolic XAI for Computational Drug Repurposing
225