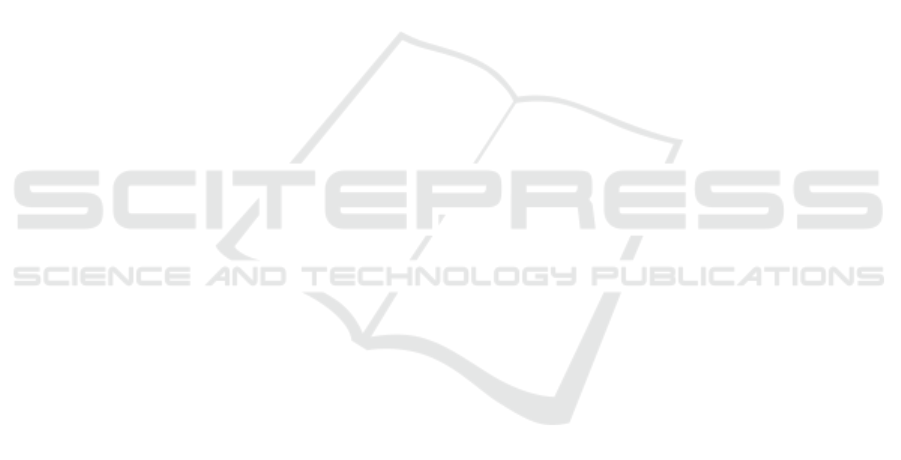
REFERENCES
Arjovsky, M. and Bottou, L. (2017). Towards principled
methods for training generative adversarial networks.
arXiv preprint arXiv:1701.04862.
Auer, S., Bizer, C., Kobilarov, G., Lehmann, J., Cyganiak,
R., and Ives, Z. (2007). Dbpedia: A nucleus for a web
of open data. In The semantic web, pages 722–735.
Springer.
Bond, F. and Foster, R. (2013). Linking and extending an
open multilingual wordnet. In Proceedings of the 51st
Annual Meeting of the Association for Computational
Linguistics (Volume 1: Long Papers), pages 1352–
1362.
Carreira, J. and Zisserman, A. (2017). Quo vadis, action
recognition? a new model and the kinetics dataset.
In proceedings of the IEEE Conference on Computer
Vision and Pattern Recognition, pages 6299–6308.
Devlin, J., Chang, M.-W., Lee, K., and Toutanova, K.
(2018). Bert: Pre-training of deep bidirectional trans-
formers for language understanding. arXiv preprint
arXiv:1810.04805.
Felix, R., Reid, I., Carneiro, G., et al. (2018). Multi-modal
cycle-consistent generalized zero-shot learning. In
Proceedings of the European Conference on Com-
puter Vision (ECCV), pages 21–37.
Fu, Y., Hospedales, T. M., Xiang, T., and Gong, S. (2015).
Transductive multi-view zero-shot learning. IEEE
transactions on pattern analysis and machine intelli-
gence, 37(11):2332–2345.
Gao, J., Zhang, T., and Xu, C. (2019). I know the relation-
ships: Zero-shot action recognition via two-stream
graph convolutional networks and knowledge graphs.
In Proceedings of the AAAI conference on artificial
intelligence, volume 33, pages 8303–8311.
Huang, H., Wang, C., Yu, P. S., and Wang, C.-D. (2019a).
Generative dual adversarial network for generalized
zero-shot learning. In Proceedings of the IEEE/CVF
Conference on Computer Vision and Pattern Recogni-
tion, pages 801–810.
Huang, K., Delany, S.-J., and McKeever, S. (2019b). Hu-
man action recognition in videos using transfer learn-
ing. In Proceedings of IMVIP 2019: Irish Machine
Vision & Image Processing, Technological University
Dublin, Dublin, Ireland.
Huang, K., Delany, S. J., and McKeever, S. (2021). Fairer
evaluation of zero shot action recognition in videos.
In VISIGRAPP (5: VISAPP), pages 206–215.
Jain, M., Van Gemert, J. C., Mensink, T., and Snoek, C. G.
(2015). Objects2action: Classifying and localizing
actions without any video example. In Proceedings
of the IEEE international conference on computer vi-
sion, pages 4588–4596.
Kataoka, H., Wakamiya, T., Hara, K., and Satoh, Y. (2020).
Would mega-scale datasets further enhance spatiotem-
poral 3d cnns? arXiv preprint arXiv:2004.04968.
Kingma, D. P. and Welling, M. (2013). Auto-encoding vari-
ational bayes. arXiv preprint arXiv:1312.6114.
Li, Y., Hu, S.-h., and Li, B. (2016). Recognizing unseen ac-
tions in a domain-adapted embedding space. In 2016
IEEE International Conference on Image Processing
(ICIP), pages 4195–4199. IEEE.
Liu, J., Kuipers, B., and Savarese, S. (2011). Recognizing
human actions by attributes. In CVPR 2011, pages
3337–3344. IEEE.
Liu, K., Liu, W., Ma, H., Huang, W., and Dong, X. (2019).
Generalized zero-shot learning for action recognition
with web-scale video data. WWW, 22(2):807–824.
Mandal, D., Narayan, S., Dwivedi, S. K., Gupta, V.,
Ahmed, S., Khan, F. S., and Shao, L. (2019). Out-of-
distribution detection for generalized zero-shot action
recognition. In Proceedings of CVPR, pages 9985–
9993.
Mettes, P. and Snoek, C. G. (2017). Spatial-aware object
embeddings for zero-shot localization and classifica-
tion of actions. In Proceedings of the IEEE inter-
national conference on computer vision, pages 4443–
4452.
Mikolov, T., Sutskever, I., Chen, K., Corrado, G. S., and
Dean, J. (2013). Distributed representations of words
and phrases and their compositionality. In Advances in
neural information processing systems, pages 3111–
3119.
Mishra, A., Pandey, A., and Murthy, H. A. (2020). Zero-
shot learning for action recognition using synthesized
features. Neurocomputing, 390:117–130.
Mishra, A., Verma, V. K., Reddy, M. S. K., Arulkumar, S.,
Rai, P., and Mittal, A. (2018). A generative approach
to zero-shot and few-shot action recognition. In 2018
IEEE Winter Conference on WACV, pages 372–380.
IEEE.
Narayan, S., Gupta, A., Khan, F. S., Snoek, C. G., and
Shao, L. (2020). Latent embedding feedback and dis-
criminative features for zero-shot classification. In
Computer Vision–ECCV 2020: 16th European Con-
ference, Glasgow, UK, August 23–28, 2020, Proceed-
ings, Part XXII 16, pages 479–495. Springer.
Soomro, K., Zamir, A. R., and Shah, M. (2012). Ucf101:
A dataset of 101 human actions classes from videos in
the wild. arXiv preprint arXiv:1212.0402.
Speer, R., Chin, J., and Havasi, C. (2017). Conceptnet 5.5:
An open multilingual graph of general knowledge. In
Thirty-first AAAI conference on artificial intelligence.
Verma, V. K., Arora, G., Mishra, A., and Rai, P. (2018).
Generalized zero-shot learning via synthesized exam-
ples. In Proceedings of the IEEE conference on com-
puter vision and pattern recognition, pages 4281–
4289.
Wang, Q. and Chen, K. (2017). Zero-shot visual recognition
via bidirectional latent embedding. IJCV, 124(3):356–
383.
Wang, X. (2013). Intelligent multi-camera video surveil-
lance: A review. Pattern recognition letters, 34(1):3–
19.
Xian, Y., Akata, Z., Sharma, G., Nguyen, Q., Hein, M., and
Schiele, B. (2016). Latent embeddings for zero-shot
classification. In Proceedings of CVPR, pages 69–77.
Xian, Y., Lorenz, T., Schiele, B., and Akata, Z. (2018). Fea-
ture generating networks for zero-shot learning. In
Zero-Shot Action Recognition with Knowledge Enhanced Generative Adversarial Networks
263