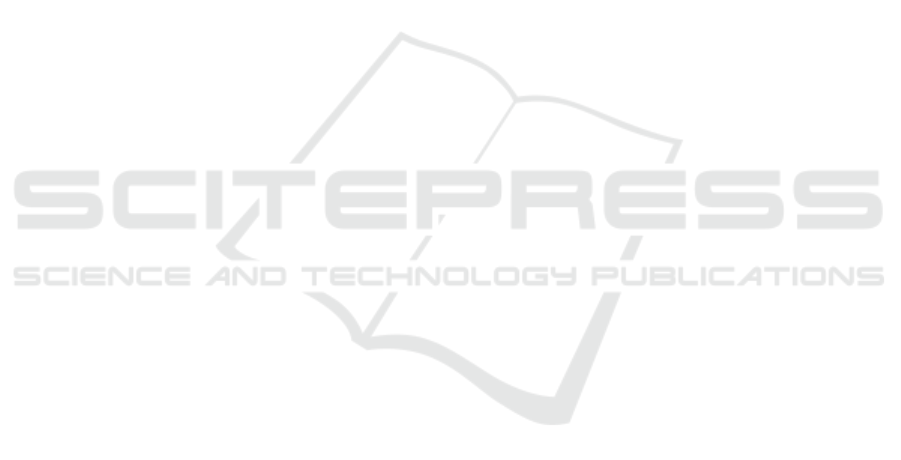
nificantly higher data quality assessment score values
than data points corresponding to lower missingness
percentage.
5 CONCLUSIONS - FUTURE
WORK
The work presented in this paper introduces a data
quality assessment approach that allows for decision
making regarding the need/efficiency of data comple-
tion in order to save system computational resources
and ensure quality of imputed data. To the best of
our knowledge, this is the first method deriving a pre-
dictive quantitative metric for data quality in the data
imputation paradigm, providing a yes or no answer
for the question: Would data completion about to per-
formed in a given batch of data is meaningful and re-
liable? The dearth of similar known works approach-
ing the data quality assessment in data imputation set-
tings in the same way deprives us of the potential to
perform extensive comparative evaluation.
Further experiments backing the validity of the
suggested score, while optimizing the score’s hyper-
parameters, in a variety of data missingness settings
will be addressed in our future work. Additionally,
we aim at expanding our validation experiments in the
multi-channel imputation setting, expecting for even
clearer evidence for our method’s utility and under-
stability. Moreover, we perform cross-validation to
demonstrate the proposed metric is insusceptible to
the selection of different data completion techniques.
Finally, although data quality has been assessed in
other setups, its application in the context of data im-
putation optimization has not been studied, thus not
being possible for us to perform more extensive com-
parative evaluation of the proposed approach.
The presented results seem to confirm the valid-
ity of the newly introduced data quality assessment
score since, as the data quality score assigned to a
given batch of input data is inversely proportional to
the value of the NRMSE yielded by the imputation
performed on that particular batch. Therefore, the ex-
ported results reinforce our initial hypothesis that the
suggested score is a suitable indicator regarding the
imputability of a given batch of data, allowing to as-
sess the potential outcome (e.g. errors introduced) of
the imputation processes, thus, saving us from unnec-
essary computational cost.
ACKNOWLEDGEMENTS
This work has been partially supported by the Smart-
Work project (GA 826343), EU H2020, SC1-DTH-
03-2018 - Adaptive smart working and living envi-
ronments supporting active and healthy ageing.
REFERENCES
Batini, C., Cappiello, C., Francalanci, C., and Maurino, A.
(2009). Methodologies for data quality assessment
and improvement. ACM computing surveys (CSUR),
41(3):1–52.
Cai, L. and Zhu, Y. (2015). The challenges of data quality
and data quality assessment in the big data era. Data
science journal, 14.
Cappiello, C., Francalanci, C., and Pernici, B. (2004). Data
quality assessment from the user’s perspective. In Pro-
ceedings of the 2004 international workshop on Infor-
mation quality in information systems, pages 68–73.
Fazakis, Nikos & Kocsis, Otilia & Dritsas, Elias & Alexiou,
Sotiris & Fakotakis, Nikos & Moustakas, Konstanti-
nos. (2021). Machine Learning Tools for Long-Term
Type 2 Diabetes Risk Prediction. IEEE Access. PP. 1-
1. 10.1109/ACCESS.2021.3098691.
George, G., Haas, M. R., and Pentland, A. (2014). Big data
and management.
Guan, Y. and Stephens, M. (2008). Practical issues in
imputation-based association mapping. PLoS genet-
ics, 4(12):e1000279.
Kocsis, O., Moustakas, K., Fakotakis, N., Hermens, H. J.,
Cabrita, M., Ziemke, T., and Kovordanyi, R. (2019a).
Conceptual architecture of a multi-dimensional mod-
eling framework for older office workers. In Pro-
ceedings of the 12th ACM International Conference
on PErvasive Technologies Related to Assistive Envi-
ronments, PETRA ’19, page 448–452, New York, NY,
USA. Association for Computing Machinery.
Kocsis, O., Moustakas, K., Fakotakis, N., Vassiliou, C.,
Toska, A., Vanderheiden, G. C., Stergiou, A., Amax-
ilatis, D., Pardal, A., Quintas, J. a., Hermens, H. J.,
Cabrita, M., Dantas, C., Hansen, S., Ziemke, T.,
Tageo, V., and Dougan, P. (2019b). Smartwork: De-
signing a smart age-friendly living and working envi-
ronment for office workers. In Proceedings of the 12th
ACM International Conference on PErvasive Tech-
nologies Related to Assistive Environments, PETRA
’19, page 435–441, New York, NY, USA. Association
for Computing Machinery.
Kocsis, O., Papoulias, G., Fakotakis, N., and Moustakas, K.
(2021). An approach to determine short- and long-
term work ability in smart work system. In Russo,
D., Ahram, T., Karwowski, W., Di Bucchianico, G.,
and Taiar, R., editors, Intelligent Human Systems Inte-
gration 2021, pages 388–394, Cham. Springer Inter-
national Publishing.
SmartWork 2021 - 2nd International Workshop on Smart, Personalized and Age-Friendly Working Environments
458