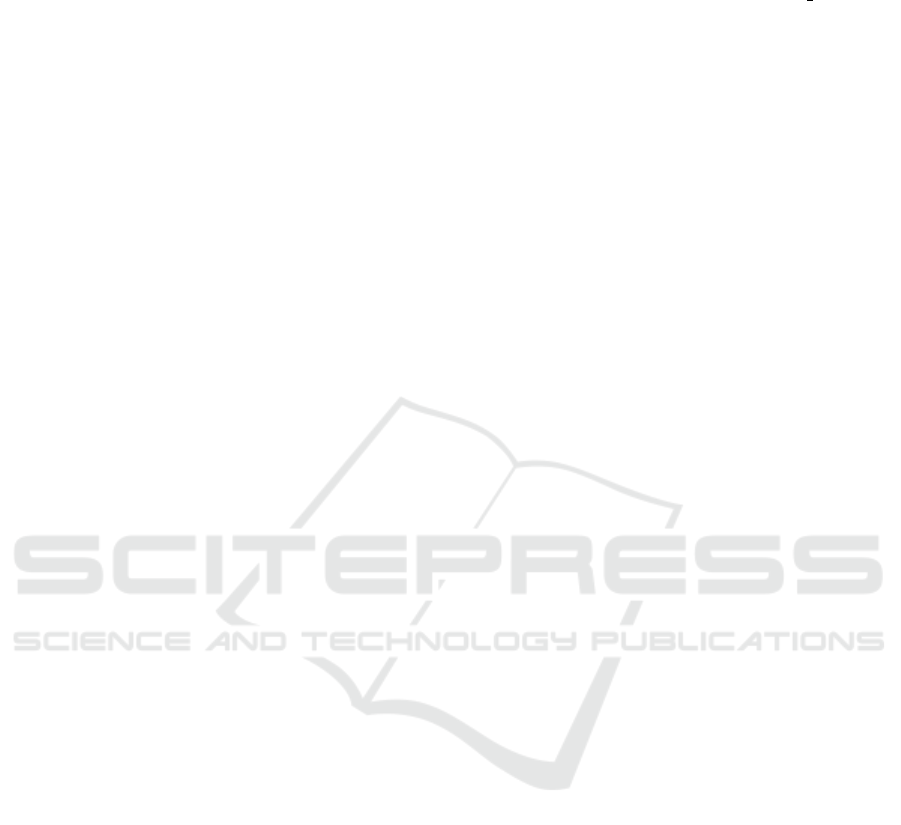
Fazakis, N., Kocsis, O., Dritsas, E., Alexiou, S., Fakotakis,
N., and Moustakas, K. (2021). Machine learning tools
for long-term type 2 diabetes risk prediction. IEEE
Access, 9:103737–103757.
Genuer, R., Poggi, J.-M., and Tuleau-Malot, C. (2010).
Variable selection using random forests. Pattern
recognition letters, 31(14):2225–2236.
Group, B. S. et al. (2000). Secondary prevention by raising
hdl cholesterol and reducing triglycerides in patients
with coronary artery disease. the bezafibrate infarction
prevention (bip) study. Circulation, 102:21–27.
Kocsis, O., Moustakas, K., Fakotakis, N., Vassiliou, C.,
Toska, A., Vanderheiden, G. C., Stergiou, A., Amaxi-
latis, D., Pardal, A., Quintas, J., et al. (2019). Smart-
work: designing a smart age-friendly living and work-
ing environment for office workers. In Proceedings
of the 12th ACM International Conference on PErva-
sive Technologies Related to Assistive Environments,
pages 435–441.
Landwehr, N., Hall, M., and Frank, E. (2005). Logistic
model trees. Machine learning, 59(1-2):161–205.
Lee, J. S., Cheong, H. S., and Shin, H. D. (2018). Prediction
of cholesterol ratios within a korean population. Royal
Society open science, 5(1):171204.
Liu, J., Sempos, C., Donahue, R. P., Dorn, J., Trevisan,
M., and Grundy, S. M. (2005). Joint distribution of
non-hdl and ldl cholesterol and coronary heart disease
risk prediction among individuals with and without di-
abetes. Diabetes care, 28(8):1916–1921.
Liu, W., Wang, Z., Liu, X., Zeng, N., Liu, Y., and Alsaadi,
F. E. (2017). A survey of deep neural network ar-
chitectures and their applications. Neurocomputing,
234:11–26.
Mahmood, S. S., Levy, D., Vasan, R. S., and Wang, T. J.
(2014). The framingham heart study and the epidemi-
ology of cardiovascular disease: a historical perspec-
tive. The lancet, 383(9921):999–1008.
Marmot, M., Oldfield, Z., Clemens, S., Blake, M., Phelps,
A., Nazroo, J., et al. (2018). English longitudinal
study of ageing: Waves 0–8, 1998–2017.
Muthukrishnan, R. and Rohini, R. (2016). Lasso: A fea-
ture selection technique in predictive modeling for
machine learning. In 2016 IEEE international confer-
ence on advances in computer applications (ICACA),
pages 18–20. IEEE.
Quinlan, J. R. (1986). Induction of decision trees. Machine
learning, 1(1):81–106.
Quinlan, J. R. (2014). C4. 5: programs for machine learn-
ing. Elsevier.
Reggiani, F., Carraro, M., Belligoli, A., Sanna, M., Dal Pr
`
a,
C., Favaretto, F., Ferrari, C., Vettor, R., and Tosatto,
S. C. (2020). In silico prediction of blood cholesterol
levels from genotype data. PloS one, 15(2):e0227191.
Rodr
´
ıguez, J., Kuncheva, L., and Alonso, C. (2006). Rota-
tion forest: A new classifier ensemble method. IEEE
transactions on pattern analysis and machine intelli-
gence, 28:1619–30.
Rodrigues, A. P., Kislaya, I., Antunes, L., Gaio, V., Bar-
reto, M., Santos, A., Gil, A., Namorado, S., Lyshol,
H., Nunes, B., et al. (2016). Prevalence of elevated
cholesterol in portugal: National health examination
survey results (2015) ana paula rodrigues. The Euro-
pean Journal of Public Health, 26(suppl 1):ckw174–
112.
Stone, M. (1978). Cross-validation: A review. Statis-
tics: A Journal of Theoretical and Applied Statistics,
9(1):127–139.
Sundhari, S. S. (2011). A knowledge discovery using de-
cision tree by gini coefficient. In 2011 International
Conference on Business, Engineering and Industrial
Applications, pages 232–235. IEEE.
Triguero, I., Garc
´
ıa, S., and Herrera, F. (2015). Self-labeled
techniques for semi-supervised learning: taxonomy,
software and empirical study. Knowledge and Infor-
mation systems, 42(2):245–284.
Wakabayashi, I. and Groschner, K. (2009). Modification
of the association between alcohol drinking and non-
hdl cholesterol by gender. Clinica Chimica Acta,
404(2):154–159.
SmartWork 2021 - 2nd International Workshop on Smart, Personalized and Age-Friendly Working Environments
450