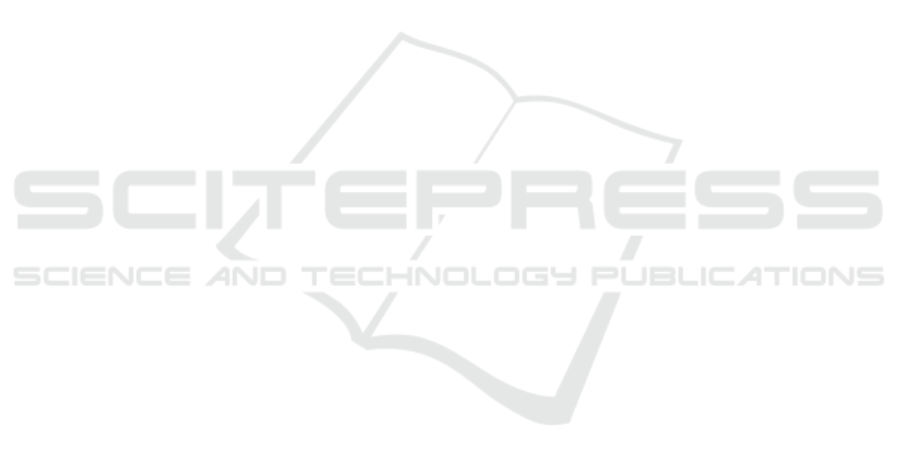
4 CONCLUSIONS
The present work proposed a new hybrid method of
detecting drivers’ drowsiness based on time-
frequency analysis of EEG signals from a single
channel (FP1).
We extracted a total of eight features from the
three domains, the time, Fourier and PSD. After that,
we trained eight machine learning models, support
vector machine (with its four kernels), Gaussian
process (GP), K-Nearest-Neighbors (KNN), Multi-
layer Perceptron (MLP), and Decision Tree (DT).
We compared our work to previous ones based on
the same dataset available at the Physionet database,
and the use of a single channel of EEG records. The
added value of our model is the improvement of the
detection’s performance in the term of accuracy,
which achieved 95.7% and the processing time 0.062
seconds.
REFERENCES
B, V. P., & Chinara, S. (2021). Automatic
classification methods for detecting drowsiness
using wavelet packet transform extracted time-
domain features from single-channel EEG
signal. Journal of Neuroscience Methods, 347,
108927. doi: 10.1016/j.jneumeth.2020.108927
Bajaj, V., Taran, S., Khare, S. K., & Sengur, A.
(2020). Feature extraction method for
classification of alertness and drowsiness states
EEG signals. Applied Acoustics, 163, 107224.
doi: 10.1016/j.apacoust.2020.107224
Belakhdar, I., Kaaniche, W., Djemal, R., & Ouni, B.
(2018). Single-channel-based automatic
drowsiness detection architecture with a
reduced number of EEG features.
Microprocessors and Microsystems, 58, 13–23.
doi: 10.1016/j.micpro.2018.02.004
Budak, U., Bajaj, V., Akbulut, Y., Atilla, O., &
Sengur, A. (2019). An Effective Hybrid Model
for EEG-Based. 19(17), 7624–7631.
Chang, W., Chen, L., Member, S., & Chiou, Y.
(2018). Design and Implementation of a
Drowsiness -Fatigue-Detection System Based
on Wearable Smart Glasses to Increase Road
Safety. IEEE Transactions on Consumer
Electronics, PP(c), 1. doi:
10.1109/TCE.2018.2872162
Des, R., & Des, S. (2016). Recueil des statistiques des
accidents corporels de la circulation routière
2016.
Dhanalakshmi, S., Rosepet, J. J., Rosy, G. L., &
Philominal, M. (2016). Drowsy Driver
Identification Using MATLAB. 4(Iv), 198–205.
Kiashari, S. E. H., Nahvi, A., Bakhoda, H.,
Homayounfard, A., & Tashakori, M. (2020).
Evaluation of driver drowsiness using
respiration analysis by thermal imaging on a
driving simulator. Multimedia Tools and
Applications. doi: 10.1007/s11042-020-08696-
x
Ouabida, E., Essadike, A., & Bouzid, A. (2020).
Optik Optical correlator based algorithm for
driver drowsiness detection. Optik -
International Journal for Light and Electron
Optics, 204(December 2019), 164102. doi:
10.1016/j.ijleo.2019.164102
Statistiques, R. D. E. S. (2017). Recueil des
statistiques des accidents corporels de la
circulation routière 2017. 1–129.
Strijkstra, A. M., Beersma, D. G. M., Drayer, B.,
Halbesma, N., & Daan, S. (2003). Subjective
sleepiness correlates negatively with global
alpha ( 8 – 12 Hz ) and positively with central
frontal theta ( 4 – 8 Hz ) frequencies in the
human resting awake electroencephalogram.
340, 17–20. doi: 10.1016/S0304-
3940(03)00033-8
Automatic Drowsiness Detection based on a Single Channel of EEG Signals using a Hybrid Analysis and Decision Tree Classification
Method under Python
305