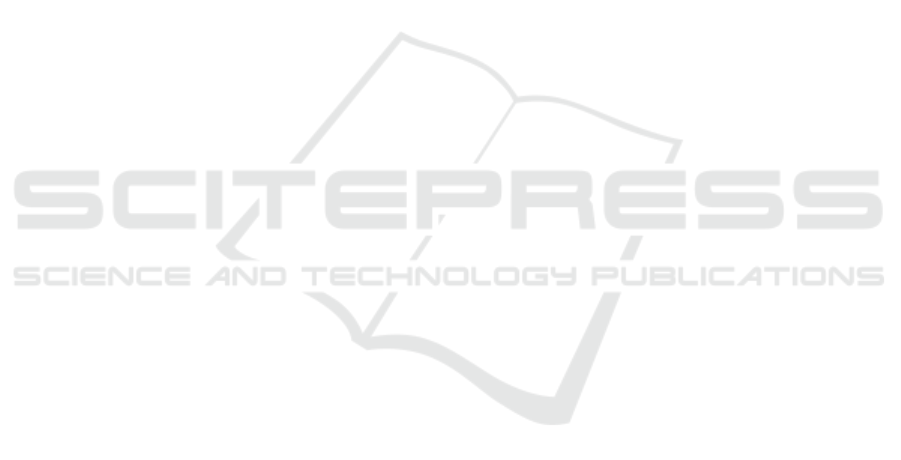
5 CONCLUSIONS
Knowledge Graph (KG) has a big impact on the
education field, practice, improvement of the quality
of teaching, and solving high school exercises.
Overall, we conclude that KGs are capable of
providing semantically organized data.
In this paper, We discussed how knowledge
graphs can be used in a variety of domains,
including Question Answering, Recommendation,
and Information Retrieval. Also, we presented a
background for the KG approach, which includes
KG definitions, two methods of knowledge graph
construction: top-down and bottom-up, and the
presentation of KG Embeddings models. A
comparison of different models of knowledge graphs
utilized in the field of education was offered.
We intend to expand this research in the
future by incorporating educational applications and
methodological extensions of KG-based algorithms
for multimodal extraction and analysis.
REFERENCES
Aliyu, I., Kana, A., and Aliyu, S. (2020). Development of
knowledge graph for university courses management.
International Journal of Education and Management
Engineering, 10(2):1.
Bellini, V., Anelli, V. W., Di Noia, T., and Di Sciascio,
E. (2017). Auto-encoding user ratings via knowledge
graphs in recommendation scenarios. In Proceedings
of the 2nd Workshop on Deep Learning for
Recommender Systems, pages 60–66.
Berant, J., Chou, A., Frostig, R., and Liang, P. (2013).
Semantic parsing on freebase from question-answer
pairs. In Proceedings of the 2013 conference on
empirical methods in natural language processing,
pages 1533–1544.
Bonatti, P. A., Decker, S., Polleres, A., and Presutti,
V. (2019). Knowledge graphs: New directions
for knowledge representation on the semantic web
(dagstuhl seminar 18371). In Dagstuhl Reports,
volume 8. Schloss Dagstuhl-Leibniz-Zentrum fuer
Informatik.
Bordes, A., Usunier, N., Garcia-Duran, A., Weston, J., and
Yakhnenko, O. (2013). Translating embeddings for
modeling multi-relational data. In Neural Information
Processing Systems (NIPS), pages 1–9.
Chen, P., Lu, Y., Zheng, V. W., Chen, X., and Li, X. (2018a).
An automatic knowledge graph construction system
for k-12 education. In Proceedings of the Fifth Annual
ACM Conference on Learning at Scale, pages 1–4.
Chen, P., Lu, Y., Zheng, V. W., Chen, X., and Yang, B.
(2018b). Knowedu: a system to construct knowledge
graph for education. Ieee Access, 6:31553–31563.
Dettmers, T., Minervini, P., Stenetorp, P., and Riedel,
S. (2018). Convolutional 2d knowledge graph
embeddings. In Proceedings of the AAAI Conference
on Artificial Intelligence, volume 32.
Fader, A., Zettlemoyer, L., and Etzioni, O. (2014).
Open question answering over curated and extracted
knowledge bases. In Proceedings of the 20th ACM
SIGKDD international conference on Knowledge
discovery and data mining, pages 1156–1165.
Fensel, D., S¸ims¸ek, U., Angele, K., Huaman, E., K
¨
arle, E.,
Panasiuk, O., Toma, I., Umbrich, J., and Wahler, A.
(2020). Knowledge Graphs. Springer.
Huang, Z., Xu, W., and Yu, K. (2015). Bidirectional
lstm-crf models for sequence tagging. arXiv preprint
arXiv:1508.01991.
Lin, Y., Liu, Z., Sun, M., Liu, Y., and Zhu, X.
(2015). Learning entity and relation embeddings
for knowledge graph completion. In Proceedings
of the AAAI Conference on Artificial Intelligence,
volume 29.
Liu, X. and Fang, H. (2015). Latent entity space: a
novel retrieval approach for entity-bearing queries.
Information Retrieval Journal, 18(6):473–503.
Liu, Z., Xiong, C., Sun, M., and Liu, Z. (2018). Entity-duet
neural ranking: Understanding the role of knowledge
graph semantics in neural information retrieval. arXiv
preprint arXiv:1805.07591.
Morwal, S., Jahan, N., and Chopra, D. (2012). Named
entity recognition using hidden markov model
(hmm). International Journal on Natural Language
Computing (IJNLC), 1(4):15–23.
Nguyen, D. Q., Nguyen, T. D., Nguyen, D. Q., and
Phung, D. (2017). A novel embedding model for
knowledge base completion based on convolutional
neural network. arXiv preprint arXiv:1712.02121.
Nickel, M., Rosasco, L., and Poggio, T. (2016).
Holographic embeddings of knowledge graphs. In
Proceedings of the AAAI Conference on Artificial
Intelligence, volume 30.
Qin, Y., Cao, H., and Xue, L. (2020). Research and
application of knowledge graph in teaching: Take the
database course as an example. In Journal of Physics:
Conference Series, volume 1607, page 012127. IOP
Publishing.
Su, K.-Y., Su, J., Wiebe, J., and Li, H. (2009). Proceedings
of the joint conference of the 47th annual meeting
of the acl and the 4th international joint conference
on natural language processing of the afnlp. In
Proceedings of the Joint Conference of the 47th
Annual Meeting of the ACL and the 4th International
Joint Conference on Natural Language Processing of
the AFNLP.
Tran, H. N. and Takasu, A. (2019). Analyzing
knowledge graph embedding methods from a multi-
embedding interaction perspective. arXiv preprint
arXiv:1903.11406.
Trouillon, T., Welbl, J., Riedel, S., Gaussier,
´
E., and
Bouchard, G. (2016). Complex embeddings for
simple link prediction. In International Conference
on Machine Learning, pages 2071–2080. PMLR.
Comparative Study of Knowledge Graph Models in Education Domain
343