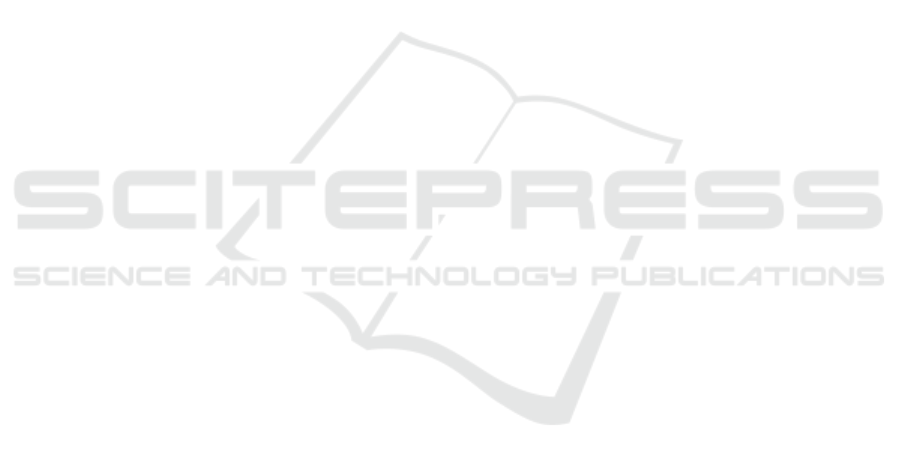
using a business simulator with machine learning ele-
ments include computers, smartphones, tablets with
Internet access, a business simulator with machine
learning elements; teaching materials.
The result of the proposed methodology: the skills
of managing personal money are formed at a high
level; acquired skills to successfully apply a business
simulator with elements of machine learning to per-
form practical work.
Within the framework of this methodology, we of-
fer different forms and methods of using a business
simulator with machine learning elements to develop
personal finance management skills: organization and
development of a business simulator with machine
learning elements to develop personal finance man-
agement skills; sessions of using a business simulator
with elements of machine learning to simulate socio-
economic situations that correspond to the topic of
the lesson; organization of thematic economic train-
ing using a business simulator with elements of ma-
chine learning; visualization of economic and behav-
ioral concepts; using a business simulator with ele-
ments of machine learning as a means of targeted in-
depth problem-based learning; using a business sim-
ulator with elements of machine learning as a means
of organizing an assessment.
At the same time, the organization and develop-
ment process of a business simulator with elements
of machine learning for the development of personal
finance management skills ensures the use of knowl-
edge needed to complete a project in the field of pro-
fessional activity of students of IT specialties as an
incentive for obtaining new knowledge in the field of
economics. In the process of developing a business
simulator with elements of machine learning, the ac-
quisition of skills and abilities is ensured, as well as
the formation of a personal attitude to the problematic
issues of the economic industry.
Sessions of using a business simulator with
elements of machine learning to simulate socio-
economic situations that correspond to the subject of
the lesson material provide students with interest, pro-
vide the necessary theoretical information in the field
of the lesson, organize the possibility of obtaining
skills in this topic, and initiate the formation of stu-
dents’ personal attitude to the socio-economic situa-
tion, considered by focusing on a specific economic
law, principle or concept, which reduces the complex-
ity of understanding.
The organization of thematic economic training
using a business simulator with elements of machine
learning contributes to motivation and increased at-
tention to the knowledge of the lesson material. Ob-
taining the skills and abilities of rational behavior in
the problematic area of training is an integral part of
the training, providing one’s own experience, close to
practice and the formation of a stable personal atti-
tude, provoked by the experience of mastering prob-
lematic material and the active generation of relevant
conclusions.
Visualization of economic and behavioral con-
cepts allows students to be motivated to perceive the
lesson material. When using a business simulator
with elements of machine learning with dynamic pa-
rameters or dynamic content, provides students with
the skills and abilities to manage an object of a socio-
economic nature. Visualization and selection of top-
ics relevant to the audience contribute to the formation
of one’s own attitude towards the object of knowl-
edge. The use of a business simulator with elements
of machine learning as a means of targeted in-depth
problem-based learning basically contains the need
for independent work on organizing such a lesson.
This approach allows for the development of per-
sonal finance management skills, initiating the inde-
pendent study of the lesson material. Supplementing
this method with an assessment in the form of an es-
say, including questions on the relevance of the sub-
ject of the simulation conducted for the student who
conducts it, and the group as a whole, contributes to
the formation of a personal attitude to the problems of
the studied material.
A separate object of study in the process of using
such a simulator can be the results of comparing the
optimality of decisions made by a person and a model
using machine learning algorithms. Such a compar-
ison complements the learning process with visual
data on the causes and consequences of applying var-
ious approaches to managing personal finances.
Consider the main features of this simulator,
which are presented in different sections of the simu-
lator (figure 2):
1. Current account management options.
2. Savings management options.
3. Deposit management options.
4. Credit management options.
5. Non-financial investment management options.
6. Information on changes in current accounts that
have occurred in the last week.
7. Analytical information on the dynamics of
changes in current account funds, savings, and in-
vestments.
8. Information on current exchange rates.
9. List of recent transactions that have been made on
current accounts.
The Methodology for Using a Business Simulator with Elements of Machine Learning to Develop Personal Finance Management Skills
95