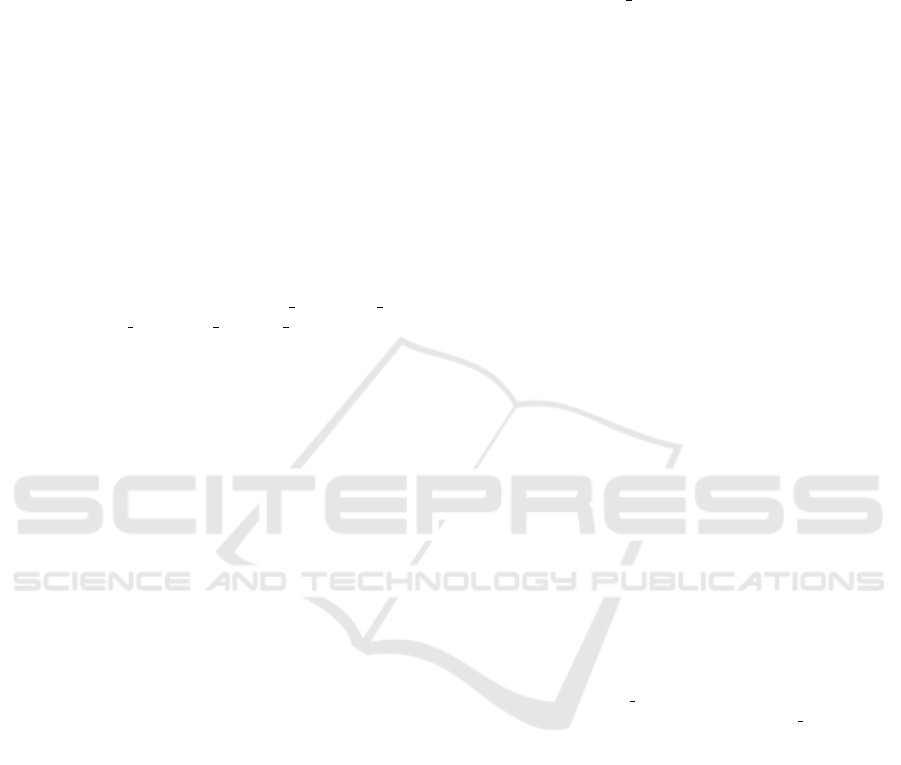
workforce, volume 27 of International Perspectives
on Education and Society, pages 239–277. Emerald
Group Publishing Limited. https://doi.org/10.1108/
S1479-367920140000027013.
Horal, L., Khvostina, I., Reznik, N., Shyiko, V., Yashcher-
itsyna, N., Korol, S., and Zaselskiy, V. (2020).
Predicting the economic efficiency of the business
model of an industrial enterprise using machine learn-
ing methods. In Kiv, A., editor, Proceedings of
the Selected Papers of the Special Edition of In-
ternational Conference on Monitoring, Modeling &
Management of Emergent Economy (M3E2-MLPEED
2020), Odessa, Ukraine, July 13-18, 2020, volume
2713 of CEUR Workshop Proceedings, pages 334–
351. CEUR-WS.org. https://ceur-ws.org/Vol-2713/
paper37.pdf.
Isac, C., Nita, D., and Dura, C. (2010). Optimizing
Franchising Investment Decision Using Elec-
tre and Rompedet Methods. The IUP Jour-
nal of Managerial Economics, 8(1/2):7–32.
https://www.iupindia.in/510/IJME
Optimizing
Franchising Investment Decision 7.html.
Kardan, A. A., Sadeghi, H., Ghidary, S. S., and Sani,
M. R. F. (2013). Prediction of student course selec-
tion in online higher education institutes using neural
network. Computers & Education, 65:1–11. https:
//doi.org/10.1016/j.compedu.2013.01.015.
Kirichek, G., Harkusha, V., Timenko, A., and Kulykovska,
N. (2019). System for detecting network anoma-
lies using a hybrid of an uncontrolled and con-
trolled neural network. CEUR Workshop Proceed-
ings, 2546:138–148. https://ceur-ws.org/Vol-2546/
paper09.pdf.
Kondruk, N. E. and Maliar, M. M. (2019). Bagatokry-
terialna optymizatsiia liniinykh system [Multicriteria
optimization of linear systems]. Autdor-Shark, Uzh-
gorod, Ukraine. https://dspace.uzhnu.edu.ua/jspui/
handle/lib/24042.
Lesinski, G., Corns, S., and Dagli, C. (2016). Application
of an Artificial Neural Network to Predict Graduation
Success at the United States Military Academy. Pro-
cedia Computer Science, 95:375–382. https://doi.org/
10.1016/j.procs.2016.09.348.
Liu, C., Feng, Y., and Yuling, W. (2022). An innova-
tive evaluation method for undergraduate education:
an approach based on bp neural network and stress
testing. Studies in Higher Education, 47(1):212–228.
https://doi.org/10.1080/03075079.2020.1739013.
Mahapatra, S. S. and Khan, M. S. (2007). A neural net-
work approach for assessing quality in technical edu-
cation: an empirical study. International Journal of
Productivity and Quality Management, 2(3):287–306.
https://doi.org/10.1504/IJPQM.2007.012451.
Markova, O. M., Semerikov, S., and Popel, M. (2018).
Cocalc as a learning tool for neural network simula-
tion in the special course ”foundations of mathematic
informatics”. In Ermolayev, V., Su
´
arez-Figueroa,
M. C., Yakovyna, V., Kharchenko, V. S., Kobets,
V., Kravtsov, H., Peschanenko, V. S., Prytula, Y.,
Nikitchenko, M. S., and Spivakovsky, A., editors, Pro-
ceedings of the 14th International Conference on ICT
in Education, Research and Industrial Applications.
Integration, Harmonization and Knowledge Transfer.
Volume II: Workshops, Kyiv, Ukraine, May 14-17,
2018, volume 2104 of CEUR Workshop Proceedings,
pages 388–403. CEUR-WS.org. https://ceur-ws.org/
Vol-2104/paper 204.pdf.
M
¨
uller, B., Reinhardt, J., and Strickland, M. T. (1995). Neu-
ral Networks: An Introduction. Physics of Neural Net-
works. Springer-Verlag Berlin Heidelberg, 2 edition.
https://doi.org/10.1007/978-3-642-57760-4.
Okubo, F., Yamashita, T., Shimada, A., and Konomi, S.
(2017a). Students’ performance prediction using data
of multiple courses by recurrent neural network. In
Mohd Ayub, A. F., Mitrovic, A., Yang, J.-C., Wong,
S. L., and Chen, W., editors, Proceedings of the 25th
International Conference on Computers in Education,
ICCE 2017 - Main Conference Proceedings, page 439
– 444. Asia-Pacific Society for Computers in Educa-
tion. https://www.apsce.net/icce/icce2017/140.115.
135.84/icce/icce2017/sites/default/files/proceedings/
main/C3/Students%20Performance%20Prediction%
20Using%20Data%20of%20Multiple%20Courses%
20by%20Recurrent%20Neural%20Network.pdf.
Okubo, F., Yamashita, T., Shimada, A., and Ogata, H.
(2017b). A neural network approach for students’
performance prediction. In Proceedings of the Sev-
enth International Learning Analytics & Knowledge
Conference, LAK ’17, page 598–599, New York, NY,
USA. Association for Computing Machinery. https:
//doi.org/10.1145/3027385.3029479.
Osadchyi, V., Kruglyk, V., and Bukreyev, D. (2018). De-
velopment of a software product for forecasting the
entrance of applicants to higher educational institu-
tions. Ukrainian Journal of Educational Studies and
Information Technology, 6(3):55–69. https://doi.org/
10.32919/uesit.2018.03.06.
Parvu, I. and Ipate, D. M. (2007). Mathematical model of
measuring the quality of services of the higher ed-
ucation institutions. Journal of Applied Economic
Sciences, 2(1(2)
Fall2007). https://EconPapers.repec.
org/RePEc:ush:jaessh:v:2:y:2007:i:1(2) fall2007:5.
Pererva, V. V., Lavrentieva, O. O., Lakomova, O. I., Zaval-
niuk, O. S., and Tolmachev, S. T. (2020). The tech-
nique of the use of virtual learning environment in the
process of organizing the future teachers’ terminolog-
ical work by specialty. CTE Workshop Proceedings,
7:321–346. https://doi.org/10.55056/cte.363.
Porokhnya, V. and Ostapenko, O. (2019). Neural network
and index forecasting of the strategies of development
of the armed forces of ukraine depending on their own
economic opportunities and encroachments of the ag-
gressor states. In Kiv, A., Semerikov, S., Soloviev,
V. N., Kibalnyk, L., Danylchuk, H., and Matviy-
chuk, A., editors, Proceedings of the Selected Pa-
pers of the 8th International Conference on Monitor-
ing, Modeling & Management of Emergent Economy,
M3E2-EEMLPEED 2019, Odessa, Ukraine, May 22-
24, 2019, volume 2422 of CEUR Workshop Proceed-
ings, pages 111–120. CEUR-WS.org. https://ceur-ws.
org/Vol-2422/paper09.pdf.
Rivas, A., Gonz
´
alez-Briones, A., Hern
´
andez, G., Prieto,
Methodology for Assessing the Quality of an Educational Program and Educational Activities of a Higher Education Institution Using a
Neural Network
197