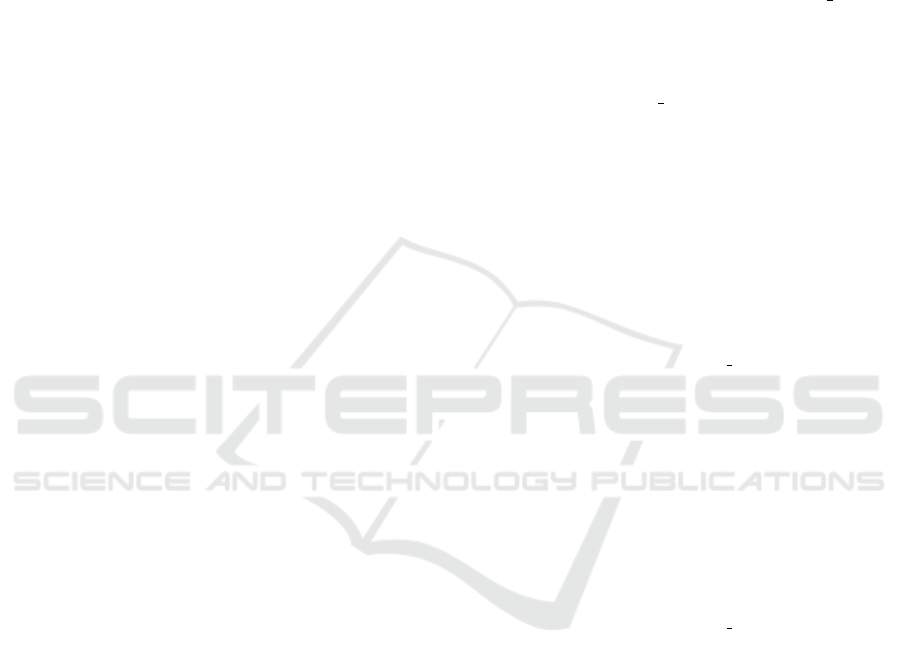
Nicolis, G. and Prigogine, I. (1989). Exploring complexity:
An Introduction. W.H. Freeman New York.
Nobel Foundation (2021). Press release: The nobel prize
in physics 2021. https://www.nobelprize.org/prizes/
physics/2021/press-release/.
Noth, M., Borning, A., and Waddell, P. (2003). An
extensible, modular architecture for simulating ur-
ban development, transportation, and environmental
impacts. Computers, Environment and Urban Sys-
tems, 27(2):181–203. https://www.sciencedirect.com/
science/article/pii/S0198971501000308.
Ovens, A., Hopper, T., and Butler, J. (2013). Complexity
Thinking in Physical Education: Reframing Curricu-
lum, Pedagogy and Research. Routledge, 1 edition.
https://doi.org/10.4324/9780203126455.
Pagels, H. (1988). The Dreams of Reason: The Computer
and the Rise of the Sciences of Complexity. Simon &
Schuster.
Pareto, V. (1896). Cours d’
´
Economie Politique, volume 1.
Poincar
´
e, H. (1890). Sur le probl
`
eme des trois corps et les
´
equations de la dynamique. Acta Math, 13:1–270.
Posadas, A., Morales, J., and Posadas-Garzon, A. (2021).
Earthquakes and entropy: Characterization of occur-
rence of earthquakes in Southern Spain and Alboran
Sea. Chaos: An Interdisciplinary Journal of Nonlin-
ear Science, 31(4):043124. https://doi.org/10.1063/5.
0031844.
Prigogine, I. (1980). From Being to Becoming Time and
Complexity in the Physical Sciences. W.H. Freeman.
Prigogine, I. (1989). The philosophy of instability.
Futures, 21(4):396–400. https://doi.org/10.1016/
S0016-3287(89)80009-6.
Prigogine, I. and Stengers, I. (1984). Order Out of Chaos
Man’s New Dialogue with Nature. Bantam Books.
Prigogine, I. and Stengers, I. (1997). The End of Certainty.
Free Press.
Ram
´
ırez-Montoya, M. S., Castillo-Mart
´
ınez, I. M.,
Sanabria-Z, J., and Miranda, J. (2022). Com-
plex Thinking in the Framework of Education 4.0
and Open Innovation – A Systematic Literature Re-
view. Journal of Open Innovation: Technology, Mar-
ket, and Complexity, 8(1):4. https://doi.org/10.3390/
joitmc8010004.
Saravia, L. A., Doyle, S. R., and Bond-Lamberty, B.
(2018). Power laws and critical fragmentation in
global forests. Scientific Reports, 8(1):17766. https:
//doi.org/10.1038/s41598-018-36120-w.
Shannon, C. E. (1948). A Mathematical Theory of
Communication. Bell System Technical Journal,
27(3):379–423. https://doi.org/10.1002/j.1538-7305.
1948.tb01338.x.
Shumway, R. H. and Stoffer, D. S. (2016). Time Se-
ries Analysis and Its Applications. With R Examples.
Springer Texts in Statistics. Springer, 4 edition.
Sigahi, T. F. A. C. and Sznelwar, L. I. (2022). Explor-
ing applications of complexity theory in engineering
education research: A systematic literature review.
Journal of Engineering Education, 111(1):232–260.
https://doi.org/10.1002/jee.20438.
Soloviev, V. N. and Belinskiy, A. (2018). Complex Systems
Theory and Crashes of Cryptocurrency Market. In Er-
molayev, V., Su
´
arez-Figueroa, M. C., Yakovyna, V.,
Mayr, H. C., Nikitchenko, M. S., and Spivakovsky, A.,
editors, Information and Communication Technolo-
gies in Education, Research, and Industrial Applica-
tions - 14th International Conference, ICTERI 2018,
Kyiv, Ukraine, May 14-17, 2018, Revised Selected Pa-
pers, volume 1007 of Communications in Computer
and Information Science, pages 276–297. Springer.
https://doi.org/10.1007/978-3-030-13929-2 14.
Soloviev, V. N. and Belinskiy, A. (2019). Complex Sys-
tems Theory and Crashes of Cryptocurrency Mar-
ket. Communications in Computer and Information
Science, 1007:276 – 297. https://doi.org/10.1007/
978-3-030-13929-2 14.
Soloviev, V. N. and Belinskyi, A. (2018a). Methods of Non-
linear Dynamics and the Construction of Cryptocur-
rency Crisis Phenomena Precursors. In Ermolayev, V.,
Su
´
arez-Figueroa, M. C., Yakovyna, V., Kharchenko,
V. S., Kobets, V., Kravtsov, H., Peschanenko, V. S.,
Prytula, Y., Nikitchenko, M. S., and Spivakovsky, A.,
editors, Proceedings of the 14th International Confer-
ence on ICT in Education, Research and Industrial
Applications. Integration, Harmonization and Knowl-
edge Transfer. Volume II: Workshops, Kyiv, Ukraine,
May 14-17, 2018, volume 2104 of CEUR Workshop
Proceedings, pages 116–127. CEUR-WS.org. https:
//ceur-ws.org/Vol-2104/paper 175.pdf.
Soloviev, V. N. and Belinskyi, A. (2018b). Methods of Non-
linear Dynamics and the Construction of Cryptocur-
rency Crisis Phenomena Precursors. In Ermolayev, V.,
Su
´
arez-Figueroa, M. C., Yakovyna, V., Kharchenko,
V. S., Kobets, V., Kravtsov, H., Peschanenko, V. S.,
Prytula, Y., Nikitchenko, M. S., and Spivakovsky, A.,
editors, Proceedings of the 14th International Confer-
ence on ICT in Education, Research and Industrial
Applications. Integration, Harmonization and Knowl-
edge Transfer. Volume II: Workshops, Kyiv, Ukraine,
May 14-17, 2018, volume 2104 of CEUR Workshop
Proceedings, pages 116–127. CEUR-WS.org. http:
//ceur-ws.org/Vol-2104/paper 175.pdf.
Soloviev, V. N., Bielinskyi, A., Serdyuk, O., Solovieva,
V., and Semerikov, S. (2020a). Lyapunov Expo-
nents as Indicators of the Stock Market Crashes. In
Sokolov, O., Zholtkevych, G., Yakovyna, V., Tara-
sich, Y., Kharchenko, V., Kobets, V., Burov, O., Se-
merikov, S., and Kravtsov, H., editors, Proceedings
of the 16th International Conference on ICT in Ed-
ucation, Research and Industrial Applications. Inte-
gration, Harmonization and Knowledge Transfer. Vol-
ume II: Workshops, Kharkiv, Ukraine, October 06-10,
2020, volume 2732 of CEUR Workshop Proceedings,
pages 455–470. CEUR-WS.org. http://ceur-ws.org/
Vol-2732/20200455.pdf.
Soloviev, V. N., Bielinskyi, A., and Solovieva, V. (2019).
Entropy Analysis of Crisis Phenomena for DJIA In-
dex. In Ermolayev, V., Mallet, F., Yakovyna, V.,
Kharchenko, V. S., Kobets, V., Kornilowicz, A.,
Kravtsov, H., Nikitchenko, M. S., Semerikov, S., and
Spivakovsky, A., editors, Proceedings of the 15th
International Conference on ICT in Education, Re-
AET 2021 - Myroslav I. Zhaldak Symposium on Advances in Educational Technology
218