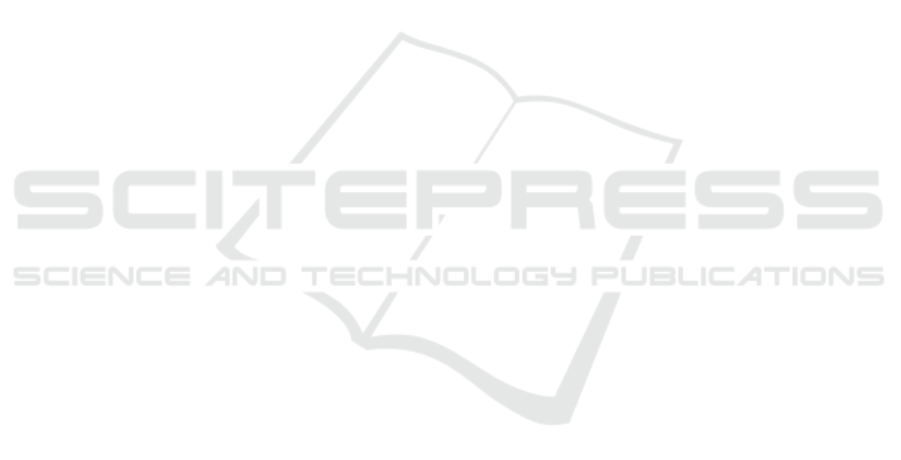
6 CONCLUSIONS
In this paper, we have presented data from experi-
ments on the k-beauty contest game. We provide ac-
cess to raw data files and files with data manipulation,
metrics calculation, and plot building. This will hope-
fully support reproducibility in this area of research.
Then we discuss the results of the experiments and
provide an analysis and explanation of patterns of be-
havior. It seems possible to confirm the existence of a
pattern in decision-making – every group behaves al-
most the same way when dealing with an unknown
strategic situation. We can formulate findings in a
few short notes. First, participants have chosen not
winning moves (> 66) partly because of a new situ-
ation and trouble understanding the rules. However,
a high percentage of such choices was present in the
second round, when players knew what was going on.
This effect was especially notable in the cases of high
school and adults and almost zero in the case of spe-
cial math schools and kids below 9th grade. We can
hypothesizeesis that high school is the age of experi-
mentation when children discover new things ared do
not afraid to do so. Second, the winning number as
the decision of a group is decreasing in all cases, so
we can see that group is learning fast and steady. Even
if some outliers choose 100, the mean still declines
with every round. There seems to be an unspoken
competition between players that leads to improve-
ment in the aggregated decision even if no prize is at
stake. It is a plausible scenario when all participants
choose higher numbers. However, this did not hap-
pen in any experiment. Third, a a stable percent of
people choose about 100, and it is not about learning
how to play the game. We think this is something like
a -1 level of reasoning when the player intentionally
plays a “bad move”, and this is an essential part of the
model. If we neglect such persons and their motiva-
tion, our model will not be correct.
In the second part of the paper, we presented an
agent-based model using conclusions about human
behavior in the game. Simplification is the key to
building good ABM, so in the model, agents have
only a few parameters: level and irrationality. Based
on these two parameters, agents choose a number
on each step. Depending on other players’ choices
model determine winners and losers: this state influ-
ences agents’ future level and irrationality. We per-
form about ten thousand games (each ten rounds long)
and apply the same analysis as before. This approach
shows that in some aspects, agents’ behavior is close
to humans. Future investigation of this model will
concentrate on the following possible modifications:
• deterministic choices of agents;
• different numbers of reasoning levels;
• rewards and punishments as elements of learning;
• implementation learning models from current re-
search;
• build testing environment to automatically com-
pare different learning strategies.
REFERENCES
Aumann, R. and Brandenburger, A. (1995). Epis-
temic Conditions for Nash Equilibrium. Economet-
rica, 63(5):1161–1180. http://www.jstor.org/stable/
2171725.
Aumann, R. J. (1995). Backward induction and com-
mon knowledge of rationality. Games and Eco-
nomic Behavior, 8(1):6–19. https://doi.org/10.1016/
S0899-8256(05)80015-6.
Camerer, C. F. (2003). Behavioral game theory: Experi-
ments in strategic interaction. The Roundtable Series
in Behavioral Economics. Princeton University Press.
Camerer, C. F., Ho, T.-H., and Chong, J.-K. (2002). Sophis-
ticated experience-weighted attraction learning and
strategic teaching in repeated games. Journal of
Economic theory, 104(1):137–188. https://doi.org/10.
1006/jeth.2002.2927.
Camerer, C. F., Ho, T.-H., and Chong, J.-K. (2004). A cog-
nitive hierarchy model of games. The Quarterly Jour-
nal of Economics, 119(3):861–898. https://doi.org/10.
1162/0033553041502225.
Costa-Gomes, M., Crawford, V. P., and Broseta, B. (2001).
Cognition and Behavior in Normal-Form Games: An
Experimental Study. Econometrica, 69(5):1193–
1235. https://doi.org/10.1111/1468-0262.00239.
Crawford, V. P., Costa-Gomes, M. A., and Iriberri, N.
(2013). Structural models of nonequilibrium strategic
thinking: Theory, evidence, and applications. Journal
of Economic Literature, 51(1):5–62. https://doi.org/
10.1257/jel.51.1.5.
Epstein, J. M. (1999). Agent-based computational models
and generative social science. Complexity, 4(5):41–
60. https://cutt.ly/hKtpQa9.
Fe, E., Gill, D., and Prowse, V. L. (2019). Cognitive skills,
strategic sophistication, and life outcomes. Working
Paper Series 448, The University of Warwick. https:
//tinyurl.com/2p8jmnjn.
Gill, D. and Prowse, V. (2016). Cognitive Ability, Character
Skills, and Learning to Play Equilibrium: A Level-k
Analysis. Journal of Political Economy, 124(6):1619–
1676. https://doi.org/10.1086/688849.
G
¨
uth, W., Kocher, M., and Sutter, M. (2002). Experi-
mental ‘beauty contests’ with homogeneous and het-
erogeneous players and with interior and boundary
equilibria. Economics Letters, 74(2):219–228. https:
//doi.org/10.1016/S0165-1765(01)00544-4.
Ho, T.-H., Camerer, C., and Weigelt, K. (1998). Iterated
Dominance and Iterated Best Response in Experimen-
tal “p-Beauty Contests”. The American Economic
How Concept Learning Changes Strategic Choice in Guessing Games?
719