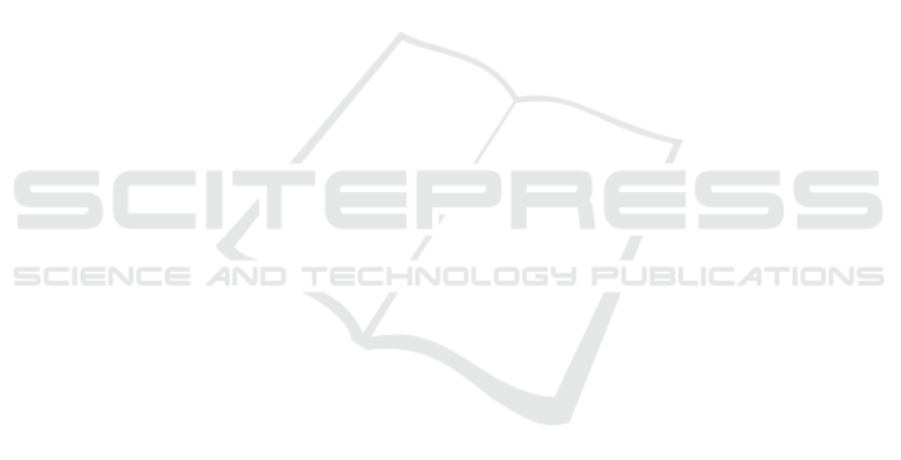
Ellis, K., Kerr, J., Godbole, S., Staudenmayer, J., and
Lanckriet, G. (2016). Hip and wrist accelerom-
eter algorithms for free-living behavior classifica-
tion. Medicine and science in sports and exercise,
48(5):933.
Freedson, P., Melanson, E., and Sirard, J. (1998). Calibra-
tion of the computer science and applications, inc. ac-
celerometer. Medicine & science in sports & exercise,
30(5):777–781.
Howcroft, J., Kofman, J., and Lemaire, E. D. (2017). Fea-
ture selection for elderly faller classification based on
wearable sensors. Journal of neuroengineering and
rehabilitation, 14(1):1–11.
Izenman, A. J. (2013). Linear discriminant analysis.
In Modern multivariate statistical techniques, pages
237–280. Springer.
Kate, R. J. (2016). Using dynamic time warping distances
as features for improved time series classification.
Data Mining and Knowledge Discovery, 30(2):283–
312.
Kreyszig, E. (2009). Advanced engineering mathematics,
10th eddition. Accessed: 26-04-2021.
Lee, K. and Kwan, M.-P. (2018). Physical activity classifi-
cation in free-living conditions using smartphone ac-
celerometer data and exploration of predicted results.
Computers, Environment and Urban Systems, 67:124–
131.
Li, H., Shrestha, A., Fioranelli, F., Le Kernec, J., Heidari,
H., Pepa, M., Cippitelli, E., Gambi, E., and Spinsante,
S. (2017). Multisensor data fusion for human activ-
ities classification and fall detection. In 2017 IEEE
SENSORS, pages 1–3. IEEE.
Ling, C. X. and Li, C. (1998). Data mining for direct mar-
keting: Problems and solutions. In Kdd, volume 98,
pages 73–79.
Mannini, A. and Intille, S. S. (2018). Classifier personal-
ization for activity recognition using wrist accelerom-
eters. IEEE journal of biomedical and health infor-
matics, 23(4):1585–1594.
Mohamed, R., Zainudin, M. N. S., Sulaiman, M. N., Peru-
mal, T., and Mustapha, N. (2018). Multi-label classi-
fication for physical activity recognition from various
accelerometer sensor positions. Journal of Informa-
tion and Communication Technology, 17(2):209–231.
Montesinos, V., Dell’Agnola, F., Arza, A., Aminifar, A.,
and Atienza, D. (2019). Multi-modal acute stress
recognition using off-the-shelf wearable devices. In
2019 41st Annual International Conference of the
IEEE Engineering in Medicine and Biology Society
(EMBC), pages 2196–2201. IEEE.
Moskovitch, R. and Shahar, Y. (2015). Classification-driven
temporal discretization of multivariate time series.
Data Mining and Knowledge Discovery, 29(4):871–
913.
Pavey, T. G., Gilson, N. D., Gomersall, S. R., Clark, B.,
and Trost, S. G. (2017). Field evaluation of a random
forest activity classifier for wrist-worn accelerome-
ter data. Journal of science and medicine in sport,
20(1):75–80.
Rastegari, E., Azizian, S., and Ali, H. (2019). Machine
learning and similarity network approaches to support
automatic classification of parkinson’s diseases using
accelerometer-based gait analysis. In Proceedings of
the 52nd Hawaii International Conference on System
Sciences.
Ravish, D., Shanthi, K., Shenoy, N. R., and Nisargh, S.
(2014). Heart function monitoring, prediction and pre-
vention of heart attacks: Using artificial neural net-
works. In 2014 International Conference on Contem-
porary Computing and Informatics (IC3I), pages 1–6.
IEEE.
Sanchez, J. A. U. and Mu
˜
noz, D. M. (2019). Fall detec-
tion using accelerometer on the user’s wrist and artifi-
cial neural networks. In XXVI Brazilian Congress on
Biomedical Engineering, pages 641–647. Springer.
Sasaki, J. E., Hickey, A., Staudenmayer, J., John, D., Kent,
J. A., and Freedson, P. S. (2016). Performance of
activity classification algorithms in free-living older
adults. Medicine and science in sports and exercise,
48(5):941.
Shensa, M. J. (1992). The discrete wavelet transform: wed-
ding the a trous and mallat algorithms. IEEE Transac-
tions on signal processing, 40(10):2464–2482.
Shoeb, A. H. and Guttag, J. V. (2010). Application of ma-
chine learning to epileptic seizure detection. In Pro-
ceedings of the 27th International Conference on Ma-
chine Learning (ICML-10), pages 975–982.
Tian, Y., Zhang, J., Wang, J., Geng, Y., and Wang, X.
(2020). Robust human activity recognition using sin-
gle accelerometer via wavelet energy spectrum fea-
tures and ensemble feature selection. Systems Science
& Control Engineering, 8(1):83–96.
Wang, X.-W., Nie, D., and Lu, B.-L. (2014). Emotional
state classification from eeg data using machine learn-
ing approach. Neurocomputing, 129:94–106.
Wang, Z., Wu, D., Chen, J., Ghoneim, A., and Hossain,
M. A. (2016). A triaxial accelerometer-based hu-
man activity recognition via eemd-based features and
game-theory-based feature selection. IEEE Sensors
Journal, 16(9):3198–3207.
Wold, S., Esbensen, K., and Geladi, P. (1987). Principal
component analysis. Chemometrics and intelligent
laboratory systems, 2(1-3):37–52.
Zhou, P.-Y. and Chan, K. C. (2015). A feature extraction
method for multivariate time series classification us-
ing temporal patterns. In Pacific-Asia Conference on
Knowledge Discovery and Data Mining, pages 409–
421. Springer.
Zubair, M., Song, K., and Yoon, C. (2016). Human activ-
ity recognition using wearable accelerometer sensors.
In 2016 IEEE International Conference on Consumer
Electronics-Asia (ICCE-Asia), pages 1–5. IEEE.
BIOSIGNALS 2022 - 15th International Conference on Bio-inspired Systems and Signal Processing
110