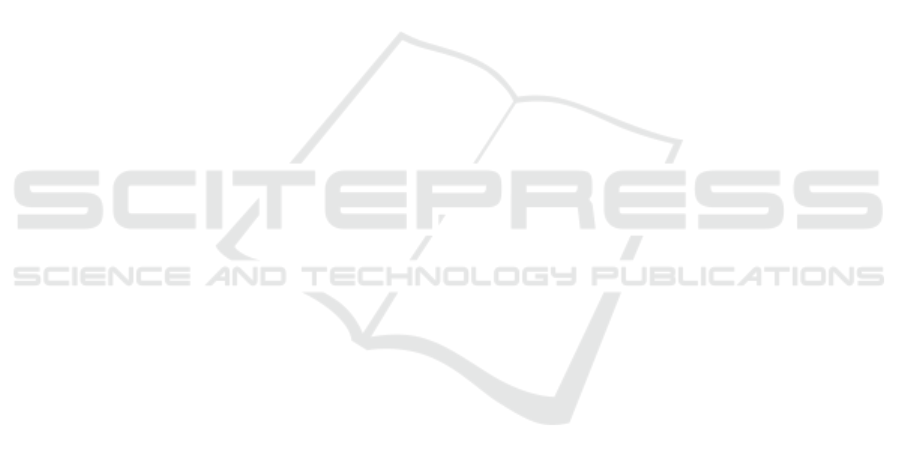
0.13 rad. Finally, the observed instance is translated
in all three axes from 0.02 to 0.1 m. Examples of the
altered observed instances with the noise, outliers, ro-
tation and translation together with their correspond-
ing holistic and hol+part registrations are presented
in Figure 6. Note how local geometries such as the
lens of the camera, the handle of the mug and the trig-
ger of the spray bottles are better captured with the
hol+part registration.
Numerical results of the drill and spray bottle cat-
egories with fully observed instances are presented in
Figure 5. Figure 7 reports the average registration er-
rors of partial views. Only two categories are pre-
sented but the remaining three behave similarly. The
hol+part variant continues outperforming the other
two methods with noise and outliers on fully observed
instances. However, for rotation and translation, the
partwise variant is more robust, which implies that
misalignments on a global level of the object are more
difficult to refine. Moreover, on partial views the part-
wise variant continues outperforming the other two
methods and is more robust against noise, outliers ro-
tation and translation.
5 CONCLUSION
In this paper, we have presented a novel part-based
non-rigid registration method that improves the accu-
racy of local structures by registering individually de-
composed parts of the object. The method is based
on learned shape spaces of each of the decomposed
parts. We have shown in the experimental section that
a holistic registration followed by a partwise registra-
tion (hol+part) is very effective for registering fully
observed objects, while a partwise registration per-
forms better with partial views. We demonstrated the
robustness of our approach against noise, outliers and
misalignments in rotation and translation. In the fu-
ture, we plan to investigate hierarchies and dependen-
cies between parts of more complex objects. More-
over, we will evaluate the strengths and limitations of
the part-based registration when applied to grasping
skill transfer methods.
REFERENCES
Adeshina, S. A. and Cootes, T. F. (2010). Evaluation of
performance of part-based models for groupwise reg-
istration. Medical Image Understanding and Analysis
Conf. (MIUA), pages 221–251.
Aleotti, J. and Caselli, S. (2011). Part-based robot grasp
planning from human demonstration. In IEEE Int.
Conf. on Robotics and Automation (ICRA), pages
4554–4560.
Aleotti, J., Rizzini, D. L., and Caselli, S. (2014). Percep-
tion and grasping of object parts from active robot ex-
ploration. Journal of Intelligent & Robotic Systems
(JINT), 76(3):401–425.
Allen, B., Curless, B., and Popovi
´
c, Z. (2003). The space
of human body shapes: Reconstruction and parame-
terization from range scans. ACM Transactions on
Graphics (TOG).
Araslanov, N., Koo, S., Gall, J., and Behnke, S. (2016).
Efficient single-view 3D co-segmentation using shape
similarity and spatial part relations. In German Conf.
on Pattern Recognition (GCPR), pages 297–308.
Blanz, V. and Vetter, T. (1999). A morphable model for the
synthesis of 3D faces. In Proc. of the 26th Conf. on
Computer Graphics and Interactive Techniques (SIG-
GRAPH), pages 187–194.
Burghard, O., Berner, A., Wand, M., Mitra, N., Seidel, H.-
P., and Klein, R. (2013). Compact part-based shape
spaces for dense correspondences. arXiv preprint
arXiv:1311.7535.
Chui, H. and Rangarajan, A. (2003). A new point matching
algorithm for non-rigid registration. Computer Vision
and Image Understanding (CVIU), pages 114–141.
Engelmann, F., St
¨
uckler, J., and Leibe, B. (2016). Joint ob-
ject pose estimation and shape reconstruction in urban
street scenes using 3D shape priors. In German Conf.
on Pattern Recognition (GCPR).
Haehnel, D., Thrun, S., and Burgard, W. (2003). An ex-
tension of the ICP algorithm for modeling nonrigid
objects with mobile robots. In Int. Joint Conf. on Ar-
tificial Intelligence (IJCAI).
Hasler, N., Stoll, C., Sunkel, M., Rosenhahn, B., and Sei-
del, H.-P. (2009). A statistical model of human pose
and body shape. In Computer Graphics Forum (Euro-
graphics).
Horaud, R., Forbes, F., Yguel, M., Dewaele, G., and Zhang,
J. (2010). Rigid and articulated point registration with
expectation conditional maximization. IEEE Trans-
actions on Pattern Analysis and Machine Intelligence
(TPAMI), pages 587–602.
Huang, Q.-X., Zhang, G.-X., Gao, L., Hu, S.-M., Butscher,
A., and Guibas, L. (2012). An optimization approach
for extracting and encoding consistent maps in a shape
collection. ACM Transactions on Graphics (TOG),
page 167.
Kim, V. G., Lipman, Y., and Funkhouser, T. (2011).
Blended intrinsic maps. In ACM Transactions on
Graphics (TOG).
Krebs, J., Mansi, T., Delingette, H., Zhang, L., Ghesu, F. C.,
Miao, S., Maier, A. K., Ayache, N., Liao, R., and Ka-
men, A. (2017). Robust non-rigid registration through
agent-based action learning. In Int. Conf. on Medi-
cal Image Computing and Computer-Assisted Inter-
vention (MICCAI), pages 344–352.
Ma, J., Zhao, J., and Yuille, A. L. (2016). Non-rigid point
set registration by preserving global and structures.
IEEE Transactions on Image Processing, pages 53–
64.
Category-level Part-based 3D Object Non-rigid Registration
801