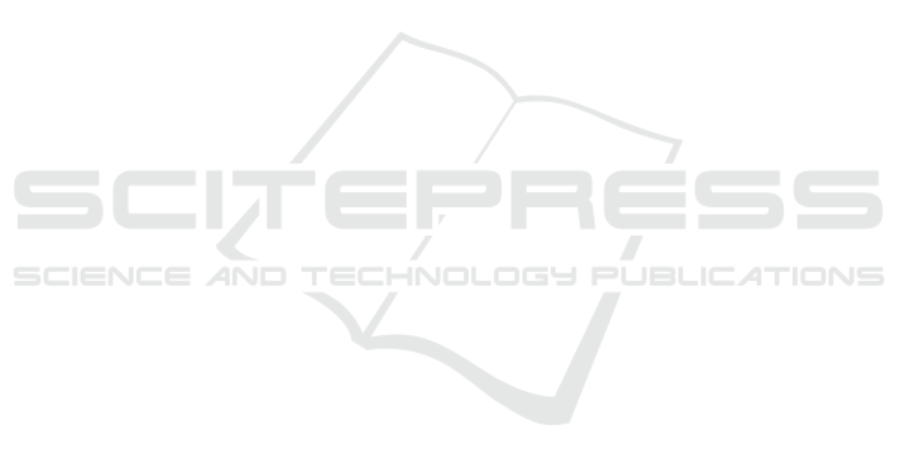
Jeetu, G. and Anusha, G. (2010). Pharmacovigilance:
a worldwide master key for drug safety monitoring.
Journal of Young Pharmacists, 2(3):315–320.
Jian-Xiang, W., Yun-Xia, Z., et al. (2015). ADRVis: an
information visualization platform for adverse drug
reactions. International Journal of u-and e-Service,
Science and Technology, 8(10):139–150.
Kakar, T., Qin, X., et al. (2019a). Mev: Visual analytics
for medication error detection. In International
Conference on Information Visualization Theory and
Applications (IVAPP). SciTePress.
Kakar, T., Qin, X., Rundensteiner, E., Harrison, L.,
Sahoo, S., and De, S. (2019b). Diva: Exploration
and validation of hypothesized drug-drug interactions.
In Computer Graphics Forum, volume 38, pages
95–106. Wiley Online Library.
Karimi, S., Wang, C., Metke-Jimenez, A., Gaire, R., and
Paris, C. (2015). Text and data mining techniques
in adverse drug reaction detection. ACM Computing
Surveys (CSUR), 47(4):56.
Kay, M., Kola, T., et al. (2016). When (ish) is my
bus? user-centered visualizations of uncertainty in
everyday, mobile predictive systems. In Proceedings
of the 2016 CHI Conference on Human Factors in
Computing Systems, pages 5092–5103.
Koch, S., John, M., W
¨
orner, M., M
¨
uller, A., and
Ertl, T. (2014). Varifocalreader—in-depth
visual analysis of large text documents. IEEE
Transactions on Visualization and Computer
Graphics, 20(12):1723–1732.
Lam, H., Bertini, E., Isenberg, P., Plaisant, C.,
and Carpendale, S. (2011). Empirical studies
in information visualization: Seven scenarios.
IEEE Transactions on Visualization and Computer
Graphics, 18(9).
Lazarou, J., Pomeranz, B. H., and Corey, P. N. (1998).
Incidence of adverse drug reactions in hospitalized
patients: a meta-analysis of prospective studies.
The Journal of American Medical Association,
279(15):1200–1205.
Liu, S., Wang, X., Collins, C., Dou, W., Ouyang, F.,
El-Assady, M., Jiang, L., and Keim, D. (2018).
Bridging text visualization and mining: A task-driven
survey. IEEE Transactions on Visualization and
Computer Graphics.
Liu, Z., G
¨
org, C., Kihm, J., Lee, H., Choo, J.,
Park, H., and Stasko, J. (2010). Data ingestion
and evidence marshalling in jigsaw vast 2010 mini
challenge 1 award: Good support for data ingest.
In IEEE Conference on Visual Analytics Science and
Technology (VAST), pages 271–272. IEEE.
Marais, K. B. and Robichaud, M. R. (2012). Analysis
of trends in aviation maintenance risk: An empirical
approach. Reliability Engineering & System Safety,
106:104–118.
Mayr, E., Hynek, N., Salisu, S., and Windhager, F.
(2019). Trust in information visualization. In EuroVis
Workshop on Trustworthy Visualization (TrustVis),
volume 20191187.
North, C. (2006). Toward measuring visualization insight.
IEEE Computer Graphics and Applications, 26(3).
North, C., Chang, R., Endert, A., Dou, W., May, R.,
Pike, B., and Fink, G. (2011). Analytic provenance:
process+ interaction+ insight. In CHI’11 Extended
Abstracts on Human Factors in Computing Systems,
pages 33–36.
Robertson, S., Zaragoza, H., et al. (2009). The
probabilistic relevance framework: Bm25 and
beyond. Foundations and Trends® in Information
Retrieval, 3(4).
SDR (2021). http://av-info.faa.gov/sdrx/ [Accessed:
2021-11-30].
Sedlmair, M., Meyer, M., and Munzner, T. (2012). Design
study methodology: Reflections from the trenches and
the stacks. IEEE Transactions on Visualization and
Computer Graphics, 18(12):2431–2440.
Serrano, N. and Ciordia, I. (2005). Bugzilla, itracker, and
other bug trackers. IEEE Software, 22(2):11–13.
Shneiderman, B., Plaisant, C., and Hesse, B. W. (2013).
Improving healthcare with interactive visualization.
Computer, 46(5):58–66.
Shrinivasan, Y. B., Gotzy, D., and Lu, J. (2009). Connecting
the dots in visual analysis. In IEEE Symposium
on Visual Analytics Science and Technology, pages
123–130. IEEE.
Stasko, J., G
¨
org, C., and Liu, Z. (2008). Sensemaking
across text documents: human-centered, visual
exploration with jigsaw. In Sensemaking Workshop@
CHI 2008.
Strobelt, H., Oelke, D., Kwon, B. C., Schreck,
T., and Pfister, H. (2015). Guidelines for
effective usage of text highlighting techniques.
IEEE Transactions on Visualization and Computer
Graphics, 22(1):489–498.
Sultanum, N., Singh, D., Brudno, M., and Chevalier, F.
(2018). Doccurate: A curation-based approach for
clinical text visualization. IEEE Transactions
on Visualization and Computer Graphics,
25(1):142–151.
Wright, W., Schroh, D., Proulx, P., Skaburskis, A.,
and Cort, B. (2006). The sandbox for analysis:
concepts and methods. In Proceedings of the SIGCHI
Conference on Human Factors in Computing Systems,
pages 801–810. ACM.
Wunnava, S., Qin, X., et al. (2017). Towards transforming
fda adverse event narratives into actionable structured
data for improved pharmacovigilance. In Proceedings
of the Symposium on Applied Computing, pages
777–782. ACM.
Wunnava, S., Qin, X., Kakar, T., Kong, X., and
Rundensteiner, E. (2020). A dual-attention network
for joint named entity recognition and sentence
classification of adverse drug events. In Proceedings
of the 2020 Conference on Empirical Methods in
Natural Language Processing: Findings, pages
3414–3423.
IVAPP 2022 - 13th International Conference on Information Visualization Theory and Applications
50