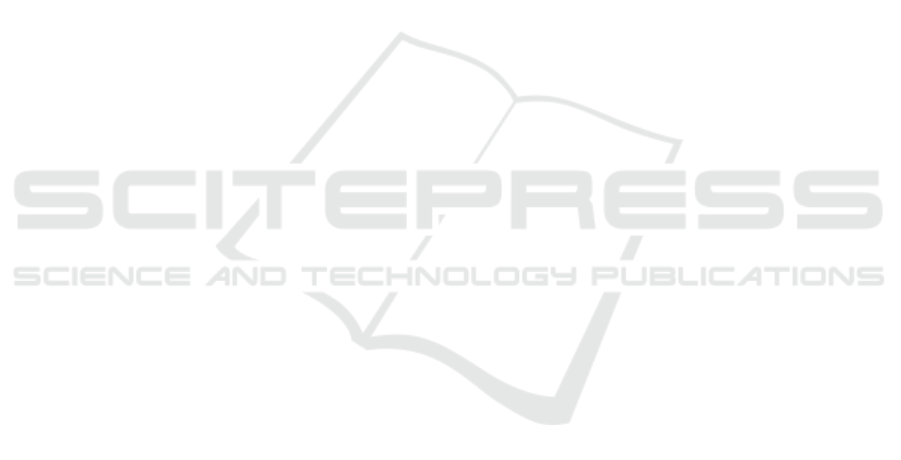
Neural Information Processing Systems, pages 7167–
7177.
Liang, S., Li, Y., and Srikant, R. (2018). Enhancing the reli-
ability of out-of-distribution image detection in neural
networks. In International Conference on Learning
Representations.
Liu, W., Wang, X., Owens, J., and Li, S. Y. (2020). Energy-
based out-of-distribution detection. Advances in Neu-
ral Information Processing Systems, 33.
McGregor, B. A. (2018). 4 - physical, chemical, and tensile
properties of cashmere, mohair, alpaca, and other rare
animal fibers. In Bunsell, A. R., editor, Handbook
of Properties of Textile and Technical Fibres (Second
Edition), The Textile Institute Book Series, pages 105
– 136. Woodhead Publishing, second edition edition.
Mendes J
´
unior, P. R., de Souza, R. M., Werneck, R. d. O.,
Stein, B. V., Pazinato, D. V., de Almeida, W. R., Pe-
natti, O. A. B., Torres, R. d. S., and Rocha, A. (2017).
Nearest neighbors distance ratio open-set classifier.
Machine Learning, 106(3):359–386.
Natekar, P. and Sharma, M. (2020). Representation based
complexity measures for predicting generalization in
deep learning. arXiv preprint arXiv:2012.02775.
Neal, L., Olson, M., Fern, X., Wong, W.-K., and Li, F.
(2018). Open set learning with counterfactual images.
In Proceedings of the European Conference on Com-
puter Vision (ECCV).
Nguyen, A., Yosinski, J., and Clune, J. (2015). Deep neural
networks are easily fooled: High confidence predic-
tions for unrecognizable images. In Proceedings of
the IEEE conference on computer vision and pattern
recognition, pages 427–436.
Oza, P. and Patel, V. M. (2019). C2ae: Class conditioned
auto-encoder for open-set recognition. In Proceedings
of the IEEE/CVF Conference on Computer Vision and
Pattern Recognition (CVPR).
Phan, K.-H. and Wortmann, F. (2001). Quality assessment
of goat hair for textile use. In Silk, Mohair, Cashmere
and Other Luxury Fibres, pages 227–233. Elsevier.
Pizer, S. M., Amburn, E. P., Austin, J. D., Cromartie, R.,
Geselowitz, A., Greer, T., ter Haar Romeny, B., Zim-
merman, J. B., and Zuiderveld, K. (1987). Adaptive
histogram equalization and its variations. Computer
vision, graphics, and image processing, 39(3):355–
368.
Rane, P. P. and Barve, S. (2011). Standardization and op-
timization of mtdna isolation and molecular genetic
analysis of d-loop region in animal natural fibres. In-
ternational Journal of Zoological Research, 7(2):190.
Rippel, O., Bilitewski, N., Rahimi, K., Kurniadi, J., Her-
rmann, A., and Merhof, D. (2021a). Identifying pris-
tine and processed animal fibers using machine learn-
ing. In 2021 IEEE International Instrumentation and
Measurement Technology Conference (I2MTC), pages
1–6.
Rippel, O., Mertens, P., and Merhof, D. (2021b). Model-
ing the distribution of normal data in pre-trained deep
features for anomaly detection. In 2020 25th Inter-
national Conference on Pattern Recognition (ICPR),
pages 6726–6733.
Robson, D. (1997). Animal fiber analysis using imaging
techniques: Part i: Scale pattern data. Textile Research
Journal, 67(10):747–752.
Robson, D. (2000). Animal fiber analysis using imaging
techniques: Part ii: Addition of scale height data. Tex-
tile Research Journal, 70(2):116–120.
Saito, K. and Saenko, K. (2021). Ovanet: One-vs-all net-
work for universal domain adaptation. arXiv preprint
arXiv:2104.03344.
Sun, X., Yang, Z., Zhang, C., Ling, K.-V., and Peng, G.
(2020). Conditional gaussian distribution learning for
open set recognition. In Proceedings of the IEEE/CVF
Conference on Computer Vision and Pattern Recogni-
tion (CVPR).
Tan, M. and Le, Q. V. (2019). EfficientNet: Rethinking
model scaling for convolutional neural networks. In
Chaudhuri, K. and Salakhutdinov, R., editors, Pro-
ceedings of the 36th International Conference on Ma-
chine Learning, ICML 2019, 9-15 June 2019, Long
Beach, California, USA, volume 97 of Proceedings
of Machine Learning Research, pages 6105–6114.
PMLR.
Waldron, S., Brown, C., and Komarek, A. M. (2014). The
chinese cashmere industry: a global value chain anal-
ysis. Development Policy Review, 32(5):589–610.
Wortmann, F.-J. (1991). Quantitative fiber mixture analy-
sis by scanning electron microscopy: Part iii: Round
trial results on mohair / wool blends. Textile Research
Journal, 61(7):371–374.
Wortmann, F.-J. and Wortmann, G. (1992). Quantita-
tive fiber mixture analysis by scanning electron mi-
croscopy: Part iv: Assessment of light microscopy as
an alternative tool for analyzing wool/specialty fiber
blends. Textile Research Journal, 62(7):423–431.
Xing, W., Liu, Y., Deng, N., Xin, B., Wang, W., and Chen,
Y. (2020a). Automatic identification of cashmere and
wool fibers based on the morphological features anal-
ysis. Micron, 128:102768.
Xing, W., Liu, Y., Xin, B., Zang, L., and Deng, N. (2020b).
The application of deep and transfer learning for iden-
tifying cashmere and wool fibers. Journal of Natural
Fibers, 0(0):1–17.
Ye, Z., Chen, Y., and Zheng, H. (2021). Understanding
the effect of bias in deep anomaly detection. arXiv
preprint arXiv:2105.07346.
Yildiz, K. (2020). Identification of wool and mohair fibres
with texture feature extraction and deep learning. IET
Image Processing, 14(2):348–353.
Zhang, H., Li, A., Guo, J., and Guo, Y. (2020). Hybrid mod-
els for open set recognition. In Vedaldi, A., Bischof,
H., Brox, T., and Frahm, J.-M., editors, Computer Vi-
sion - ECCV 2020, pages 102–117, Cham. Springer
International Publishing.
Zhang, L. and Ainsworth, W. (2005). Microscope analysis
of animal fiber blends - training of operators. Techni-
cal report.
Zisselman, E. and Tamar, A. (2020). Deep residual flow
for out of distribution detection. In Proceedings of
the IEEE/CVF Conference on Computer Vision and
Pattern Recognition (CVPR).
Zoccola, M., Lu, N., Mossotti, R., Innocenti, R., and Mon-
tarsolo, A. (2013). Identification of wool, cashmere,
yak, and angora rabbit fibers and quantitative deter-
mination of wool and cashmere in blend: a near
infrared spectroscopy study. Fibers and Polymers,
14(8):1283–1289.
Animal Fiber Identification under the Open Set Condition
47