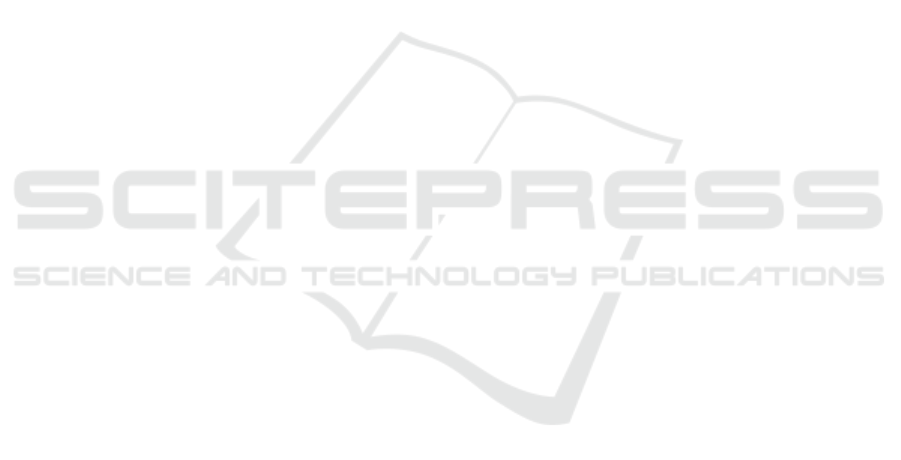
ACKNOWLEDGEMENTS
The authors would like to thank Axel Davy and
Thibaud Ehret for providing the implementation of a
contrario approach.
REFERENCES
Bay, H., Ess, A., Tuytelaars, T., and Gool, L. V. (2008).
Speeded-up robust features (SURF). Comput. Vis. Im-
age Underst., 110(3):346–359.
Bloisi, D. and Iocchi, L. (2012). Independent multimodal
background subtraction. In Computational Modelling
of Objects Represented in Images - Fundamentals,
Methods and Applications III, Third International
Symposium, CompIMAGE 2012, Rome, Italy, Septem-
ber 5-7, 2012, pages 39–44. CRC Press.
Bloisi, D. D., Pennisi, A., and Iocchi, L. (2014). Back-
ground modeling in the maritime domain. Mach. Vis.
Appl., 25(5):1257–1269.
Bovcon, B. and Kristan, M. (2020). A water-obstacle sep-
aration and refinement network for unmanned surface
vehicles. In ICRA, pages 9470–9476. IEEE.
Cane, T. and Ferryman, J. (2018). Evaluating deep seman-
tic segmentation networks for object detection in mar-
itime surveillance. pages 1–6.
Davy, A., Ehret, T., Morel, J., and Delbracio, M. (2018).
Reducing anomaly detection in images to detection in
noise. In IEEE,ICIP, pages 1058–1062. IEEE.
Desolneux, A., Moisan, L., and Morel, J.-M. (2008). From
Gestalt Theory to Image Analysis: A Probabilistic Ap-
proach, volume 34.
Elad, M. and Aharon, M. (2007). Image denoising via
sparse and redundant representations over learned dic-
tionaries. IEEE TIP, 15:3736–45.
Elgammal, A. M., Harwood, D., and Davis, L. S. (2000).
Non-parametric model for background subtraction. In
Computer Vision - ECCV 2000, 6th European Con-
ference on Computer Vision, Dublin, Ireland, June 26
- July 1, 2000, Proceedings, Part II, volume 1843 of
Lecture Notes in Computer Science, pages 751–767.
Springer.
Grosjean, B. and Moisan, L. (2009). A-contrario detectabil-
ity of spots in textured backgrounds. J. Math. Imaging
Vis., 33(3):313–337.
Heidarsson, H. K. and Sukhatme, G. S. (2011). Obstacle de-
tection from overhead imagery using self-supervised
learning for autonomous surface vehicles. In IROS,
pages 3160–3165. IEEE.
Hou, X. and Zhang, L. (2007). Saliency detection: A spec-
tral residual approach. In IEEE CVPR.
Itti, L., Koch, C., and Niebur, E. (1998). A model
of saliency-based visual attention for rapid scene
analysis. IEEE Trans. Pattern Anal. Mach. Intell.,
20(11):1254–1259.
Karnowski, J., Hutchins, E., and Johnson, C. (2015). Dol-
phin detection and tracking. IEEE, WACVW 2015,
pages 51–56.
Kristan, M., Kenk, V., Kova
ˇ
ci
ˇ
c, S., and Pers, J. (2015). Fast
image-based obstacle detection from unmanned sur-
face vehicles. IEEE transactions on cybernetics, 46.
Lebrun, M. and Leclaire, A. (2012). An implementation
and detailed analysis of the K-SVD image denoising
algorithm. Image Processing Online, 2:96–133.
Lee, S.-J., Roh, M.-I., Lee, H., Ha, J.-S., and Woo, I.-G.
(2018). Image-based ship detection and classification
for unmanned surface vehicle using real-time object
detection neural networks.
Lezama, J., Randall, G., and von Gioi, R. G. (2017). Vanish-
ing point detection in urban scenes using point align-
ments. Image Process. Line, 7:131–164.
Lowe, D. (2004). Distinctive image features from scale-
invariant keypoints. 60:91–110.
Moosbauer, S., K
¨
onig, D., J
¨
akel, J., and Teutsch, M. (2019).
A benchmark for deep learning based object detection
in maritime environments. In CVPR Workshops, pages
916–925. Computer Vision Foundation / IEEE.
Mus
´
e, P., Sur, F., Cao, F., and Gousseau, Y. (2003). Un-
supervised thresholds for shape matching. In ICIP,
pages 647–650. IEEE.
Oliver, N., Rosario, B., and Pentland, A. (2000). A bayesian
computer vision system for modeling human inter-
actions. IEEE Trans. Pattern Anal. Mach. Intell.,
22(8):831–843.
Onunka, C. and Bright, G. (2010). Autonomous marine
craft navigation: On the study of radar obstacle detec-
tion. In ICARCV, pages 567–572. IEEE.
Prasad, D. K., Prasath, C. K., Rajan, D., Rachmawati, L.,
Rajabally, E., and Quek, C. (2019). Object detection
in a maritime environment: Performance evaluation of
background subtraction methods. IEEE Trans. Intell.
Transp. Syst., 20(5):1787–1802.
Prasad, D. K., Rajan, D., Rachmawati, L., Rajabally, E.,
and Quek, C. (2017). Video processing from electro-
optical sensors for object detection and tracking in a
maritime environment: A survey. IEEE Trans. Intell.
Transp. Syst., 18(8):1993–2016.
Shin, B., Tao, J., and Klette, R. (2015). A superparticle filter
for lane detection. Pattern Recognit., 48(11):3333–
3345.
Shocher, A., Cohen, N., and Irani, M. (2018). ”zero-shot”
super-resolution using deep internal learning. In 2018
IEEE Conference on Computer Vision and Pattern
Recognition, CVPR 2018, Salt Lake City, UT, USA,
June 18-22, 2018, pages 3118–3126. IEEE Computer
Society.
Sobral, A. (2013). BGSLibrary: An opencv c++ back-
ground subtraction library. In IX Workshop de Vis
˜
ao
Computacional (WVC’2013), Rio de Janeiro, Brazil.
Sobral, A., Bouwmans, T., and Zahzah, E. Double-
constrained RPCA based on saliency maps for fore-
ground detection in automated maritime surveillance.
In AVSS, 2015, pages 1–6.
Sobral, A. and Vacavant, A. (2014). A comprehensive re-
view of background subtraction algorithms evaluated
with synthetic and real videos. Comput. Vis. Image
Underst., 122:4–21.
Unidentified Floating Object Detection in Maritime Environment
73