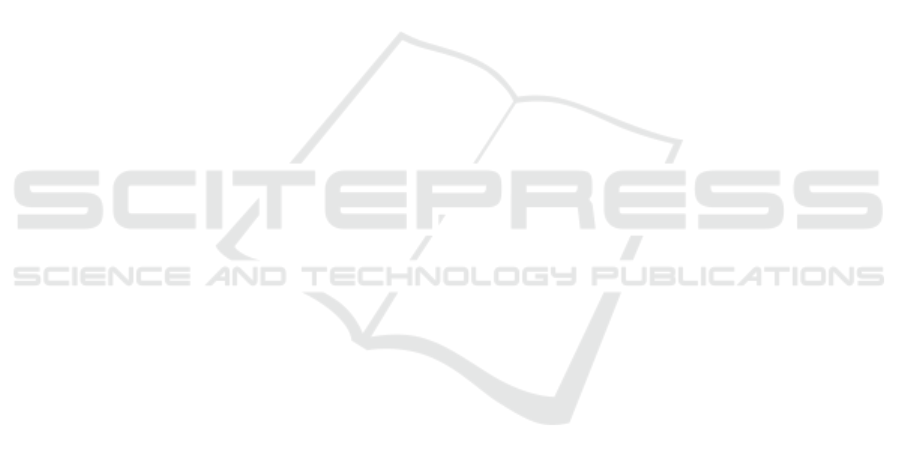
Gastaldi, X. (2017). Shake-shake regularization. arXiv
preprint arXiv:1705.07485.
Harris, E., Marcu, A., Painter, M., Niranjan, M., Pr
¨
ugel-
Bennett, A., and Hare, J. (2020). Understanding and
enhancing mixed sample data augmentation. arXiv
preprint arXiv:2002.12047.
Laine, S. and Aila, T. (2017). Temporal ensembling for
semi-supervised learning. In International Conference
on Learning Representations.
Li, J., Xiong, C., and Hoi, S. C. (2020). Semi-supervised
learning with contrastive graph regularization. arXiv
preprint arXiv:2011.11183.
Lundh, F., Clark, A., et al. Pillow.
Luo, Y., Zhu, J., Li, M., Ren, Y., and Zhang, B.
(2018). Smooth neighbors on teacher graphs for semi-
supervised learning. In IEEE Conference on Computer
Vision and Pattern Recognition, pages 8896–8905.
Oliver, A., Odena, A., Raffel, C., Cubuk, E. D., and Good-
fellow, I. J. (2018). Realistic evaluation of semi-
supervised learning algorithms. In International Con-
ference on Learning Representations.
Pham, H., Dai, Z., Xie, Q., and Le, Q. V. (2021). Meta
pseudo labels. In Proceedings of the IEEE/CVF Con-
ference on Computer Vision and Pattern Recognition,
pages 11557–11568.
Rasmus, A., Berglund, M., Honkala, M., Valpola, H., and
Raiko, T. (2015). Semi-supervised learning with ladder
networks. In Advances in neural information process-
ing systems, pages 3546–3554.
Sajjadi, M., Javanmardi, M., and Tasdizen, T. (2016a). Mu-
tual exclusivity loss for semi-supervised deep learning.
In 23rd IEEE International Conference on Image Pro-
cessing, ICIP 2016.
Sajjadi, M., Javanmardi, M., and Tasdizen, T. (2016b). Regu-
larization with stochastic transformations and perturba-
tions for deep semi-supervised learning. In Advances
in Neural Information Processing Systems, pages 1163–
1171.
Salimans, T., Goodfellow, I., Zaremba, W., Cheung, V., Rad-
ford, A., and Chen, X. (2016). Improved techniques
for training gans. In Advances in neural information
processing systems, pages 2234–2242.
Shu, R., Bui, H., Narui, H., and Ermon, S. (2018). A DIRT-T
approach to unsupervised domain adaptation. In Inter-
national Conference on Learning Representations.
Sohn, K., Berthelot, D., Li, C.-L., Zhang, Z., Carlini, N.,
Cubuk, E. D., Kurakin, A., Zhang, H., and Raffel, C.
(2020). Fixmatch: Simplifying semi-supervised learn-
ing with consistency and confidence. arXiv preprint
arXiv:2001.07685.
Sutskever, I., Martens, J., Dahl, G., and Hinton, G. (2013).
On the importance of initialization and momentum in
deep learning. In International conference on machine
learning, pages 1139–1147.
Tarvainen, A. and Valpola, H. (2017). Mean teachers are
better role models: Weight-averaged consistency tar-
gets improve semi-supervised deep learning results. In
Advances in Neural Information Processing Systems,
pages 1195–1204.
Verma, V., Lamb, A., Kannala, J., Bengio, Y., and Lopez-
Paz, D. (2019). Interpolation consistency training for
semi-supervised learning. CoRR, abs/1903.03825.
Wang, F., Kong, T., Zhang, R., Liu, H., and Li, H. (2021).
Self-supervised learning by estimating twin class dis-
tributions. arXiv preprint arXiv:2110.07402.
Wang, X., Kihara, D., Luo, J., and Qi, G.-J. (2019).
EnAET: Self-trained ensemble autoencoding transfor-
mations for semi-supervised learning. arXiv preprint
arXiv:1911.09265.
Xie, Q., Dai, Z., Hovy, E., Luong, M.-T., and Le, Q. V.
(2019). Unsupervised data augmentation. arXiv
preprint arXiv:1904.12848.
Yun, S., Han, D., Oh, S. J., Chun, S., Choe, J., and Yoo, Y.
(2019). Cutmix: Regularization strategy to train strong
classifiers with localizable features. In Proceedings
of the IEEE International Conference on Computer
Vision, pages 6023–6032.
Zagoruyko, S. and Komodakis, N. (2016). Wide residual
networks. In Richard C. Wilson, E. R. H. and Smith,
W. A. P., editors, Proceedings of the British Machine
Vision Conference (BMVC), pages 87.1–87.12. BMVA
Press.
Zhai, X., Oliver, A., Kolesnikov, A., and Beyer, L. (2019).
S4l: Self-supervised semi-supervised learning. In Pro-
ceedings of the IEEE international conference on com-
puter vision, pages 1476–1485.
Zhang, H., Cisse, M., Dauphin, Y. N., and Lopez-Paz, D.
(2018). mixup: Beyond empirical risk minimization.
In International Conference on Learning Representa-
tions.
Zhong, Z., Zheng, L., Kang, G., Li, S., and Yang, Y. (2020).
Random erasing data augmentation.
VISAPP 2022 - 17th International Conference on Computer Vision Theory and Applications
84