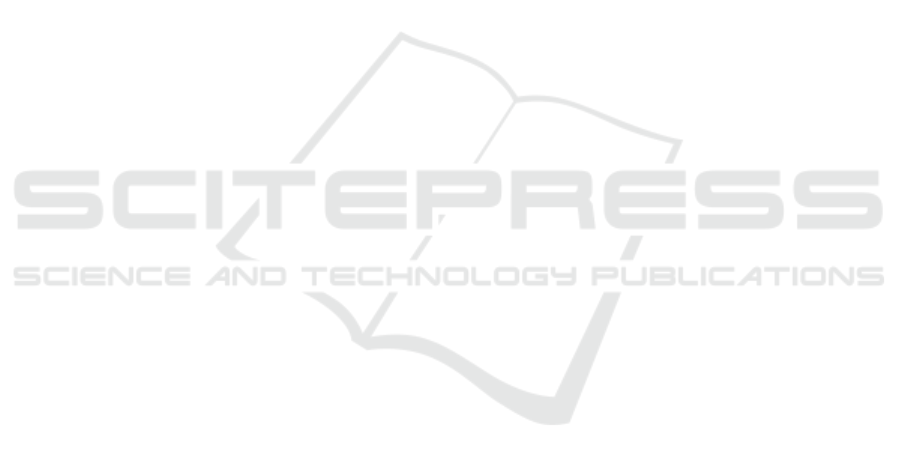
REFERENCES
Belgiu, M. and Dr
˘
agut¸, L. (2016). Random forest in remote
sensing: A review of applications and future direc-
tions. ISPRS journal of photogrammetry and remote
sensing, 114:24–31.
Chen, T., He, T., Benesty, M., Khotilovich, V., Tang, Y.,
Cho, H., et al. (2015). Xgboost: extreme gradient
boosting. R package version 0.4-2, 1(4).
Cillessen, J., Van Huffelen, A., Kappelle, L., Algra, A.,
and Van Gijn, J. (1994). Electroencephalography im-
proves the prediction of functional outcome in the
acute stage of cerebral ischemia. Stroke, 25(10):1968–
1972.
Cortes, C. and Vapnik, V. (1995). Support-vector networks.
Machine learning, 20(3):273–297.
Djamal, E. C., Ramadhan, R. I., Mandasari, M. I., and
Djajasasmita, D. (2020). Identification of post-stroke
eeg signal using wavelet and convolutional neural net-
works. Bulletin of Electrical Engineering and Infor-
matics, 9(5):1890–1898.
Gottlibe, M., Rosen, O., Weller, B., Mahagney, A., Omar,
N., Khuri, A., Srugo, I., and Genizi, J. (2020).
Stroke identification using a portable eeg device–a pi-
lot study. Neurophysiologie Clinique, 50(1):21–25.
Han, J., Kamber, M., and Pei, J. (2012). Data Mining: Con-
cepts and Techniques.
Ho, K. C., Speier, W., El-Saden, S., and Arnold, C. W.
(2017). Classifying acute ischemic stroke onset time
using deep imaging features. In AMIA Annual Sympo-
sium Proceedings, volume 2017, page 892. American
Medical Informatics Association.
Jahn, T. and Jin, B. (2020). On the discrepancy princi-
ple for stochastic gradient descent. Inverse Problems,
36(9):095009.
Jordan, K. G. (2004). Emergency eeg and continuous eeg
monitoring in acute ischemic stroke. Journal of clini-
cal neurophysiology, 21(5):341–352.
Liu, R., Xu, M., Zhang, Y., Peli, E., and Hwang, A. D.
(2020). A pilot study on electroencephalogram-based
evaluation of visually induced motion sickness. Jour-
nal of Imaging Science and Technology, 64(2):20501–
1.
Murri, L., Gori, S., Massetani, R., Bonanni, E., Marcella,
F., and Milani, S. (1998). Evaluation of acute is-
chemic stroke using quantitative eeg: a comparison
with conventional eeg and ct scan. Neurophysiologie
Clinique/Clinical Neurophysiology, 28(3):249–257.
Myles, A. J., Feudale, R. N., Liu, Y., Woody, N. A., and
Brown, S. D. (2004). An introduction to decision tree
modeling. Journal of Chemometrics: A Journal of the
Chemometrics Society, 18(6):275–285.
Narayanan, V., Arora, I., and Bhatia, A. (2013). Fast and
accurate sentiment classification using an enhanced
naive bayes model. In International Conference on In-
telligent Data Engineering and Automated Learning,
pages 194–201. Springer.
Persky, R. W., Turtzo, L. C., and McCullough, L. D. (2010).
Stroke in women: disparities and outcomes. Current
cardiology reports, 12(1):6–13.
Saadatfar, H., Khosravi, S., Joloudari, J. H., Mosavi, A., and
Shamshirband, S. (2020). A new k-nearest neighbors
classifier for big data based on efficient data pruning.
Mathematics, 8(2):286.
Soltanian-Zadeh, H. (2019). Multimodal analysis in
biomedicine. In Big Data in Multimodal Medical
Imaging, pages 193–203. Chapman and Hall/CRC.
Tolles, J. and Meurer, W. (2016). Logistic regression:
Relating patient characteristics to outcomes. JAMA,
316:533.
Wilkinson, C. M., Burrell, J. I., Kuziek, J. W.,
Thirunavukkarasu, S., Buck, B. H., and Mathewson,
K. E. (2020a). Application of the muse portable eeg
system to aid in rapid diagnosis of stroke. medRxiv.
Wilkinson, C. M., Burrell, J. I., Kuziek, J. W.,
Thirunavukkarasu, S., Buck, B. H., and Mathewson,
K. E. (2020b). Predicting stroke severity with a 3-min
recording from the muse portable eeg system for rapid
diagnosis of stroke. Scientific Reports, 10(1):1–11.
Machine Learning-based Approach for Stroke Classification using Electroencephalogram (EEG) Signals
117