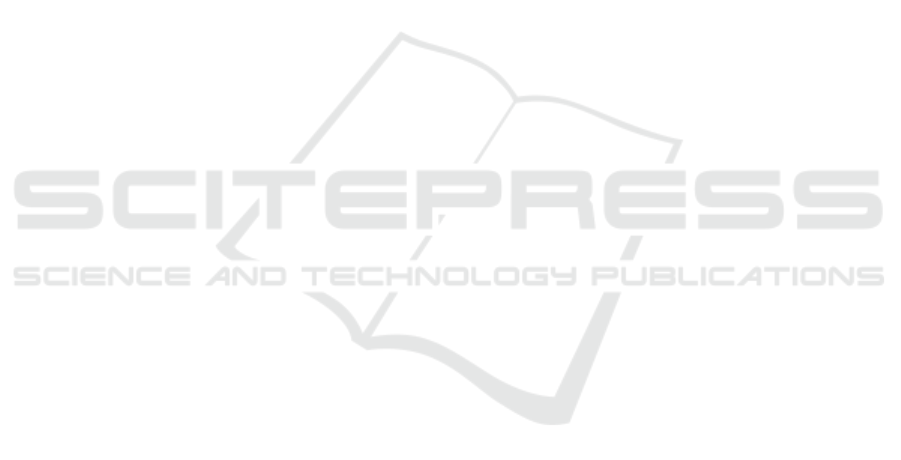
gested us “to try to increase the use cases and trials of
the app to better identify any room for improvement.”
6 CONCLUSION
In this work, we have developed a new probability
and envelope (layers) based visualization, called the
probabilistic envelope based (PE-) technique, for the
summary representation and visualization of differ-
ent drilling log data. Our PE-based representation is
easy to construct and support adjustable visualization
to provide quantitative information about the statis-
tics of the data. We have developed two visual anal-
ysis systems based on this representation to support
the anomaly detection from drilling log signals and
the casing wear analysis, both of which are impor-
tant tasks in oil and gas industries. We have applied
our systems to a number of drilling log data sets to
demonstrate their effectiveness.
Even though our results show that the PE-
technique performs much better than the standard
methods in the anomaly detection and provides a con-
cise and effective representation of various casing
wear, it is yet to assess how easily the proposed PE-
technique can be adapted to other more challenging
situations. In addition, the proposed PE-technique is
computed based on the probabilistic information. In
the future, other statistics information can be used to
aid the design of the visualization to address different
needs of specific applications.
REFERENCES
Aigner, W., Miksch, S., M
¨
uller, W., Schumann, H.,
and Tominski, C. (2007). Visualizing time-oriented
data—a systematic view. Computers & Graphics,
31(3):401 – 409.
Aigner, W., Miksch, S., M
¨
uller, W., Schumann, H., and
Tominski, C. (2008). Visual methods for analyzing
time-oriented data. IEEE transactions on visualiza-
tion and computer graphics, 14(1):47–60.
Andrienko, N., Andrienko, G., Camossi, E., Claramunt, C.,
Garcia, J. M. C., Fuchs, G., Hadzagic, M., Jousselme,
A.-L., Ray, C., Scarlatti, D., et al. (2017). Visual ex-
ploration of movement and event data with interactive
time masks. Visual Informatics, 1(1):25–39.
Chen, H., Zhang, S., Chen, W., Mei, H., Zhang, J., Mer-
cer, A., Liang, R., and Qu, H. (2015). Uncertainty-
aware multidimensional ensemble data visualization
and exploration. IEEE Transactions on Visualization
and Computer Graphics, 21(9):1072–1086.
Eren, T. (2018). Kick tolerance calculations for drilling op-
erations. Journal of Petroleum Science and Engineer-
ing, 171:558–569.
Fu, T.-c. (2011). A review on time series data min-
ing. Engineering Applications of Artificial Intelli-
gence, 24(1):164–181.
Hao, L., Healey, C. G., and Bass, S. A. (2016). Effective vi-
sualization of temporal ensembles. IEEE Transactions
on Visualization and Computer Graphics, 22(1):787–
796.
Hao, L., Healey, C. G., and Hutchinson, S. E. (2015). En-
semble visualization for cyber situation awareness of
network security data. In 2015 IEEE Symposium on
Visualization for Cyber Security (VizSec), pages 1–8.
Kincaid, R. (2010). Signallens: Focus+ context applied to
electronic time series. IEEE Transactions on Visual-
ization and Computer Graphics, 16(6):900–907.
Lejano, D., Colina, R., Yglopaz, D., Andrino, R., Malate,
R., and Ana, F. S. (2010). Casing inspection caliper
campaign in the leyte geothermal production field,
philippines. In Thirty-Fifth Workshop on Geothermal
Reservoir Engineering.
Mirzargar, M., Whitaker, R. T., and Kirby, R. M. (2014).
Curve boxplot: Generalization of boxplot for ensem-
bles of curves. IEEE transactions on visualization and
computer graphics, 20(12):2654–2663.
Potter, K., Wilson, A., Bremer, P., Williams, D., Dou-
triaux, C., Pascucci, V., and Johnson, C. R. (2009).
Ensemble-vis: A framework for the statistical visual-
ization of ensemble data. In 2009 IEEE International
Conference on Data Mining Workshops, pages 233–
240.
Raj, M., Mirzargar, M., Preston, J. S., Kirby, R. M., and
Whitaker, R. T. (2016). Evaluating shape alignment
via ensemble visualization. IEEE Computer Graphics
and Applications, 36(3):60–71.
Robertson, G., Fernandez, R., Fisher, D., Lee, B., and
Stasko, J. (2008). Effectiveness of animation in trend
visualization. IEEE transactions on visualization and
computer graphics, 14(6):1325–1332.
Van, G. A., Staals, F., L
¨
offler, M., Dykes, J., and Speck-
mann, B. (2017). Multi-granular trend detection for
time-series analysis. IEEE transactions on visualiza-
tion and computer graphics, 23(1):661–670.
Walker, J., Borgo, R., and Jones, M. W. (2015). Timenotes:
a study on effective chart visualization and interaction
techniques for time-series data. IEEE transactions on
visualization and computer graphics, 22(1):549–558.
Wang, J., Hazarika, S., Li, C., and Shen, H.-W. (2018). Vi-
sualization and visual analysis of ensemble data: A
survey. IEEE transactions on visualization and com-
puter graphics, 25(9):2853–2872.
Wilks, D. S. (2011). Statistical methods in the atmospheric
sciences, volume 100. Academic press.
Zhao, J., Chevalier, F., Pietriga, E., and Balakrishnan,
R. (2011). Exploratory analysis of time-series with
chronolenses. IEEE Transactions on Visualization and
Computer Graphics, 17(12):2422–2431.
IVAPP 2022 - 13th International Conference on Information Visualization Theory and Applications
62