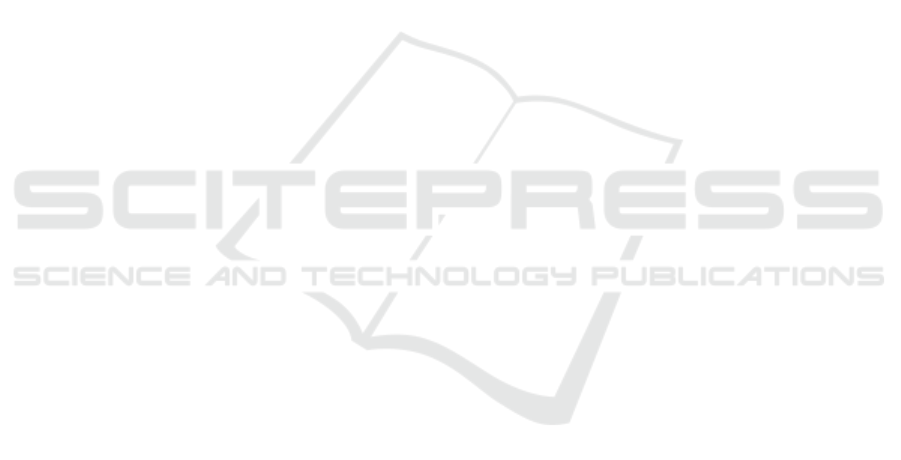
ACKNOWLEDGEMENTS
The work was supported by National Centre for
Research and Development, Poland (NCBiR); grant
BIOSTRATEG3/343547/8/NCBR/2017.
REFERENCES
Ashapure, A., Jung, J., Chang, A., Oh, S., Maeda, M., and
Landivar, J. (2019). A comparative study of rgb and
multispectral sensor-based cotton canopy cover mod-
elling using multi-temporal uas data. Remote Sensing,
11(23):2757.
Badrinarayanan, V., Kendall, A., and Cipolla, R. (2017).
Segnet: A deep convolutional encoder-decoder ar-
chitecture for image segmentation. IEEE transac-
tions on pattern analysis and machine intelligence,
39(12):2481–2495.
Bendig, J., Yu, K., Aasen, H., Bolten, A., Bennertz, S.,
Broscheit, J., Gnyp, M. L., and Bareth, G. (2015).
Combining uav-based plant height from crop surface
models, visible, and near infrared vegetation indices
for biomass monitoring in barley. International Jour-
nal of Applied Earth Observation and Geoinforma-
tion, 39:79–87.
Bosilj, P., Aptoula, E., Duckett, T., and Cielniak, G. (2020).
Transfer learning between crop types for semantic
segmentation of crops versus weeds in precision agri-
culture. Journal of Field Robotics, 37(1):7–19.
Cardim Ferreira Lima, M., Krus, A., Valero, C., Barri-
entos, A., Del Cerro, J., and Rold
´
an-G
´
omez, J. J.
(2020). Monitoring plant status and fertilization strat-
egy through multispectral images. Sensors, 20(2):435.
Castillo-Mart
´
ınez, M.
´
A., Gallegos-Funes, F. J., Carvajal-
G
´
amez, B. E., Urriolagoitia-Sosa, G., and Rosales-
Silva, A. J. (2020). Color index based thresholding
method for background and foreground segmentation
of plant images. Computers and Electronics in Agri-
culture, 178:105783.
Chadwick, A. J., Goodbody, T. R., Coops, N. C., Hervieux,
A., Bater, C. W., Martens, L. A., White, B., and
R
¨
oeser, D. (2020). Automatic delineation and height
measurement of regenerating conifer crowns under
leaf-off conditions using uav imagery. Remote Sens-
ing, 12(24):4104.
Chen, L.-C., Papandreou, G., Schroff, F., and Adam,
H. (2017). Rethinking atrous convolution for
semantic image segmentation. arXiv preprint
arXiv:1706.05587.
Deng, J., Dong, W., Socher, R., Li, L.-J., Li, K., and Fei-
Fei, L. (2009). ImageNet: A Large-Scale Hierarchical
Image Database. In CVPR09.
G Braga, J. R., Peripato, V., Dalagnol, R., P Ferreira, M.,
Tarabalka, Y., OC Arag
˜
ao, L. E., F de Campos Velho,
H., Shiguemori, E. H., and Wagner, F. H. (2020). Tree
crown delineation algorithm based on a convolutional
neural network. Remote Sensing, 12(8).
Hamuda, E., Glavin, M., and Jones, E. (2016). A survey of
image processing techniques for plant extraction and
segmentation in the field. Computers and Electronics
in Agriculture, 125:184–199.
He, K., Gkioxari, G., Doll
´
ar, P., and Girshick, R. (2017).
Mask r-cnn. In Proceedings of the IEEE international
conference on computer vision, pages 2961–2969.
He, K., Zhang, X., Ren, S., and Sun, J. (2016). Deep resid-
ual learning for image recognition. In Proceedings of
the IEEE conference on computer vision and pattern
recognition, pages 770–778.
Kapur, J. N., Sahoo, P. K., and Wong, A. K. (1985). A new
method for gray-level picture thresholding using the
entropy of the histogram. Computer vision, graphics,
and image processing, 29(3):273–285.
Lobo Torres, D., Queiroz Feitosa, R., Nigri Happ, P., Elena
Cu
´
e La Rosa, L., Marcato Junior, J., Martins, J.,
Ol
˜
a Bressan, P., Gonc¸alves, W. N., and Liesenberg,
V. (2020). Applying fully convolutional architectures
for semantic segmentation of a single tree species in
urban environment on high resolution uav optical im-
agery. Sensors, 20(2):563.
Long, J., Shelhamer, E., and Darrell, T. (2015). Fully con-
volutional networks for semantic segmentation. In
Proceedings of the IEEE conference on computer vi-
sion and pattern recognition, pages 3431–3440.
Machefer, M., Lemarchand, F., Bonnefond, V., Hitchins,
A., and Sidiropoulos, P. (2020). Mask r-cnn refitting
strategy for plant counting and sizing in uav imagery.
Remote Sensing, 12(18):3015.
Otsu, N. (1979). A threshold selection method from gray-
level histograms. IEEE transactions on systems, man,
and cybernetics, 9(1):62–66.
Patrignani, A. and Ochsner, T. E. (2015). Canopeo: A pow-
erful new tool for measuring fractional green canopy
cover. Agronomy Journal, 107(6):2312–2320.
Qiao, L., Gao, D., Zhang, J., Li, M., Sun, H., and Ma, J.
(2020). Dynamic influence elimination and chloro-
phyll content diagnosis of maize using uav spectral
imagery. Remote Sensing, 12(16):2650.
Ren, S., He, K., Girshick, R., and Sun, J. (2015). Faster
r-cnn: Towards real-time object detection with region
proposal networks. arXiv preprint arXiv:1506.01497.
Riehle, D., Reiser, D., and Griepentrog, H. W. (2020). Ro-
bust index-based semantic plant/background segmen-
tation for rgb-images. Computers and Electronics in
Agriculture, 169:105201.
Ronneberger, O., Fischer, P., and Brox, T. (2015). U-net:
Convolutional networks for biomedical image seg-
mentation. In International Conference on Medical
image computing and computer-assisted intervention,
pages 234–241. Springer.
Rosin, P. L. (2001). Unimodal thresholding. Pattern recog-
nition, 34(11):2083–2096.
Woebbecke, D. M., Meyer, G. E., Von Bargen, K., and
Mortensen, D. A. (1995). Color indices for weed iden-
tification under various soil, residue, and lighting con-
ditions. Transactions of the ASAE, 38(1):259–269.
Hybrid Method for Rapid Development of Efficient and Robust Models for In-row Crop Segmentation
281