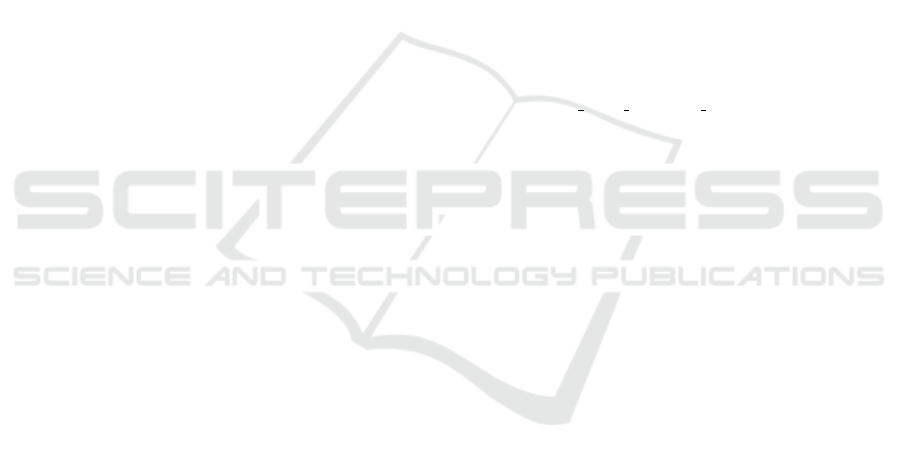
6 CONCLUSION
We present a system called Seg2Pose for converting
instance segmentation tracks into world coordinate
pose tracks for road users in static surveillance cam-
eras. The system uses our novel CNN, Seg2PoseNet,
which we show outperforms the baseline of only us-
ing normal positions on both synthetic data from
CARLA Simulator and a real world video, approx-
imately cutting the positioning errors in half. We
further show that stereo and trinocular cameras im-
prove accuracy on the CARLA dataset slightly, but
this trend is not clearly shown in our experiments with
real data.
ACKNOWLEDGEMENTS
This research was funded by VINNOVA project
2017-05510 “The Third Eye”.
REFERENCES
Ahrnbom, M., Nilsson, M., Ard
¨
o, H.,
˚
Astr
¨
om, K.,
Yastremska-Kravchenko, O., and Laureshyn, A.
(2021a). Calibration and absolute pose estimation of
trinocular linear camera array for smart city applica-
tions. In 2020 25th International Conference on Pat-
tern Recognition (ICPR), pages 103–110.
Ahrnbom, M., Nilsson, M., and Ard
¨
o, H. (2021b). Real-
time and online segmentation multi-target tracking
with track revival re-identification. In VISIGRAPP (5:
VISAPP), pages 777–784.
Bradler, H., Kretz, A., and Mester, R. (2021). Urban traf-
fic surveillance (uts): A fully probabilistic 3d tracking
approach based on 2d detections. In IEEE Intelligent
Vehicles Symposium, IV 2021, Nagoya, Japan, July 11
- July 17, 2021. IEEE.
Brazil, G., Pons-Moll, G., Liu, X., and Schiele, B. (2020).
Kinematic 3d object detection in monocular video. In
In Proceeding of European Conference on Computer
Vision, Virtual.
Dosovitskiy, A., Ros, G., Codevilla, F., Lopez, A., and
Koltun, V. (2017). CARLA: An open urban driving
simulator. In Proceedings of the 1st Annual Confer-
ence on Robot Learning, pages 1–16.
He, K., Gkioxari, G., Doll
´
ar, P., and Girshick, R. (2017).
Mask r-cnn. In Proceedings of the IEEE international
conference on computer vision, pages 2961–2969.
Hodan, T., Barath, D., and Matas, J. (2020). Epos: Estimat-
ing 6d pose of objects with symmetries. In Proceed-
ings of the IEEE/CVF Conference on Computer Vision
and Pattern Recognition (CVPR).
Jensen, M., Ahrnbom, M., Kruithof, M.,
˚
Astr
¨
om, K., Nils-
son, M., Ard
¨
o, H., Laureshyn, A., Johnsson, C.,
and Moeslund, T. (2019). A framework for auto-
mated analysis of surrogate measures of safety from
video using deep learning techniques. In Transporta-
tion Research Board. Annual Meeting Proceedings,
pages 281–306. Transportation Research Board Na-
tional Cooperative Highway Research Program. Con-
ference date: 13-01-2019 Through 17-01-2019.
Kumar, A., Brazil, G., and Liu, X. (2021). Groomed-
nms: Grouped mathematically differentiable nms for
monocular 3d object detection. In In Proceeding
of IEEE Computer Vision and Pattern Recognition,
Nashville, TN.
Lin, T.-Y., Maire, M., Belongie, S., Hays, J., Perona, P., Ra-
manan, D., Doll
´
ar, P., and Zitnick, C. L. (2014). Mi-
crosoft COCO: Common Objects in Context. In Com-
puter Vision – ECCV 2014, pages 740–755. Springer
International Publishing.
Muller, N., Wong, Y.-S., Mitra, N. J., Dai, A., and Nießner,
M. (2021). Seeing behind objects for 3d multi-object
tracking in rgb-d sequences. In Proceedings of the
IEEE/CVF Conference on Computer Vision and Pat-
tern Recognition, pages 6071–6080.
Leica Geosystems AG (2009). Leica S06 Specification.
https://w3.leica-geosystems.com/downloads123/
zz/tps/FlexLine%20TS06/brochures-datasheet/
FlexLine TS06 Datasheet en.pdf.
Lund University, Transport and Roads (2018). T-analyst.
https://bitbucket.org/TrafficAndRoads/tanalyst/wiki/
Manual.
Wu, Y., Kirillov, A., Massa, F., Lo, W.-Y., and Gir-
shick, R. (2019). Detectron2. https://github.com/
facebookresearch/detectron2.
Yang, F., Chang, X., Dang, C., Zheng, Z., Sakti, S.,
Nakamura, S., and Wu, Y. (2020). Remots: Self-
supervised refining multi-object tracking and segmen-
tation. arXiv preprint arXiv:2007.03200.
Yin, T., Zhou, X., and Krahenbuhl, P. (2021). Center-based
3d object detection and tracking. In Proceedings of
the IEEE/CVF Conference on Computer Vision and
Pattern Recognition, pages 11784–11793.
Zakharov, S., Shugurov, I., and Ilic, S. (2019). Dpod: 6d
pose object detector and refiner. In Proceedings of
the IEEE/CVF International Conference on Computer
Vision, pages 1941–1950.
Zhang, B. and Zhang, J. (2020). A traffic surveillance
system for obtaining comprehensive information of
the passing vehicles based on instance segmentation.
IEEE Transactions on Intelligent Transportation Sys-
tems, pages 1–16.
Zhang, S., Wang, C., He, Z., Li, Q., Lin, X., Li, X., Zhang,
J., Yang, C., and Li, J. (2020). Vehicle global 6-dof
pose estimation under traffic surveillance camera. IS-
PRS Journal of Photogrammetry and Remote Sensing,
159:114–128.
VISAPP 2022 - 17th International Conference on Computer Vision Theory and Applications
784