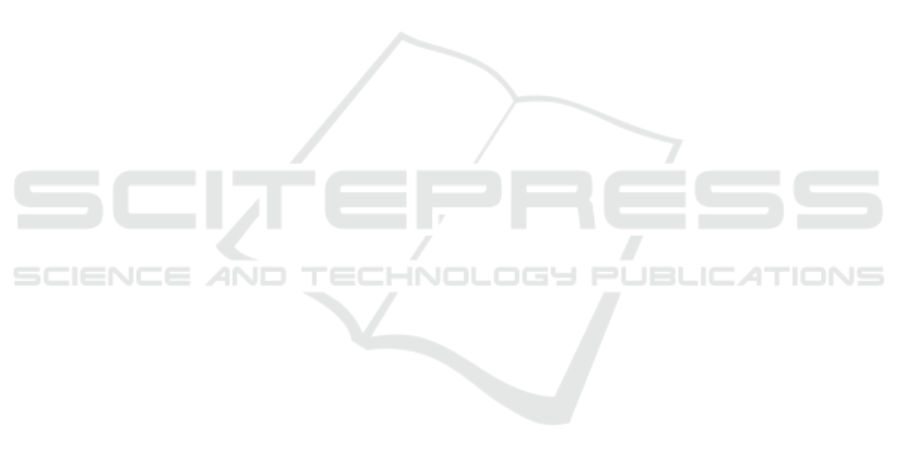
sults more applicable to daily word cloud usage. Fur-
thermore, we removed responses which we deemed
too drastically divergent from the expected interpre-
tation. One could argue that these responses indicate
actual misinterpretation of frequency series, however
we are convinced a misinterpretation of the study de-
sign is a more likely explanation, thus warranting re-
moval from the main analyses. Our sensitivity anal-
yses show that this decision did not overly influence
our findings. Finally, we limited the length of the sur-
vey in order to maximise completion, which led us to
exclude any dummy questions to check user participa-
tion. By randomising the order in which clouds were
presented (after the two initial anchoring questions)
we believe to have distributed any potential drift in
attention evenly across all clouds.
4.3 Comparison with Related Work
To our knowledge, our study is the first to quantify
the guesstimation error of word clouds in a large sam-
ple size of different types of participants. Though the
cutoff for an acceptable error is subjective, we pro-
vide clear insight into the consequence on the error
of different factors, thus enabling readers to make
their own judgement. Limitations include the non-
exhaustive selection of potential influencing variables
(e.g. word angle and colour) and their interactions.
Full assessment beyond word length and frequency
sequence would have made the survey (much) longer
and would most likely have lowered completion rates.
As mentioned in section 2.1 we have made our scripts
publicly available on GitHub for their use in future
studies. A great body of work indicates a steady in-
terest in the use of word clouds and their associated
graphs, making it very relevant to investigate their
validity in qualitative assessment. Extensive review
of the entire word cloud field is beyond the scope
of the current project and would contribute little to
the great work performed by others, such as Parejo
et al.(
´
Ursula Torres Parejo et al., 2021) Alternative
work on improving the quantitative interpretability of
word clouds or on finding alternative corpus visuali-
sations will be of value to the information visualisa-
tion field. Replication of our findings in a more stan-
dardised manner might shed further light on the way
word scaling influences our comparative assessment
of word frequency.
5 CONCLUSIONS
In conclusion, word clouds can be a misleading
method to depict relative differences in frequency.
Even in simplified form, participants vary widely
in their estimates of relative word frequency. Our
method that corrects for surface area failed to im-
prove the estimations. Word clouds are decorative in-
fographics, but unsuitable for serious scientific com-
munication.
REFERENCES
Alexander, E., Chang, C.-C., Shimabukuro, M., Franconeri,
S., Collins, C., and Gleicher, M. (2018). Percep-
tual biases in font size as a data encoding. IEEE
Transactions on Visualization and Computer Graph-
ics, 24:2397–2410.
Bayrak, S. B., Villwock, J. A., Villwock, M. R., Chiu, A. G.,
and Sykes, K. J. (2019). Using word clouds to re-
envision letters of recommendation for residency ap-
plicants. Laryngoscope., 129(9):2026–2030.
Chi, M. T., Lin, S. S., Chen, S. Y., Lin, C. H., and Lee, T. Y.
(2015). Morphable word clouds for time-varying text
data visualization. IEEE Trans. Vis. Comput. Graph,
21(12):1415–26.
Fellows, I. (2018). CRAN – Package wordcloud. CRAN.r-
project.org.
Hearst, M. A., Pedersen, E., Patil, L., Lee, E., Laskowski,
P., and Franconeri, S. (2020). An evaluation of seman-
tically grouped word cloud designs. IEEE Trans. Vis.
Comput. Graph, 26(9):2748–2761.
Medelyan, A. (2016). Why word clouds harm insights. Get-
TheMatic.com.
Mueller, A. (2015). wordcloud · PyPI. PyPI.org.
´
Ursula Torres Parejo, Campa
˜
na, J. R., Vila, M. A., and Del-
gado, M. (2021). A survey of tag clouds as tools for
information retrieval and content representation. In-
formation Visualization, 20(1):83–97.
Sellars, B. B., Sherrod, D. R., and Chappel-Aiken, L.
(2018). Using word clouds to analyze qualitative data
in clinical settings. Nurs. Manage, 49(10):51–53.
Stott, A., Zamoyski, S., and Alberti, H. (2018). Word
clouds: presenting student feedback to clinical teach-
ers. In Med, pages 1208–1209. uc, vol. 52, no. 11.
Temple, S. (2019). Word Clouds Are Lame. TowardsData-
science.com.
Vanstone, M., Toledo, F., Clarke, F., Boyle, A., Giaco-
mini, M., Swinton, M., Saunders, L., Shears, M., Zy-
taruk, N., Woods, A., Rose, T., Hand-Breckenridge,
T., Heels-Ansdell, D., Anderson-White, S., Sheppard,
R., and Cook, D. (2016). Narrative medicine and
death in the icu: word clouds as a visual legacy. BMJ
Support Palliat. Care, Nov, 2016:2016–00117.
Wang, Y., Chu, X., Bao, C., Zhu, L., Deussen, O., Chen,
B., and Seldmai, M. (2017). Edwordle: Consistency-
preserving word cloud editing. IEEE Trans. Vis. &
Comp. Graphics (Proc. IEEE Information Visualiza-
tion (Infovis) 2017).
Xiao, K., Luo, M. R., Li, C., Cui, G., and Park, D. (2011).
Investigation of colour size effect for colour appear-
ance assessment. Color Research & Application,
36(3):201–209.
IVAPP 2022 - 13th International Conference on Information Visualization Theory and Applications
128