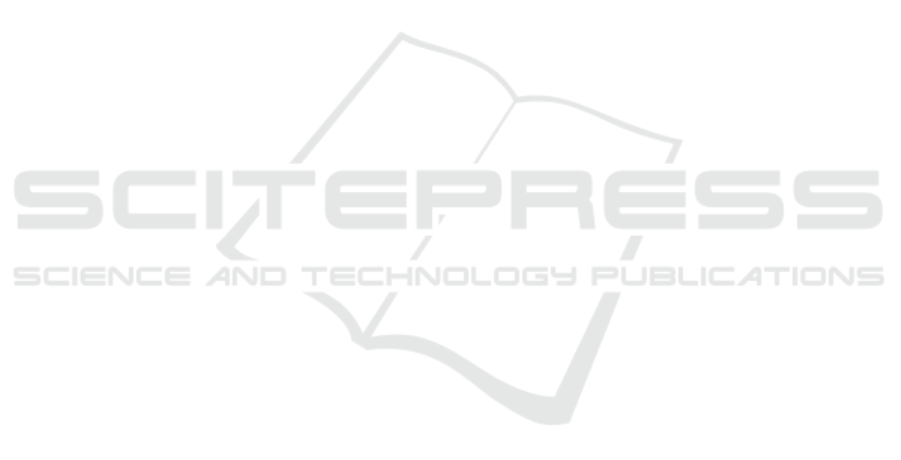
REFERENCES
Caggiano, A. (2018). Cloud-based manufacturing pro-
cess monitoring for smart diagnosis services. Inter-
national Journal of Computer Integrated Manufactur-
ing, 31(7):612–623.
Chen, J.-Y. and Lee, B.-Y. (2010). Development of a sim-
plified machine for measuring geometric parameters
of end mills.
Cupek, R., Erdogan, H., Huczala, L., Wozar, U., and
Ziebinski, A. (2015). Agent based quality manage-
ment in lean manufacturing. In Núñez, M., Nguyen,
N. T., Camacho, D., and Trawi
´
nski, B., editors, Com-
putational Collective Intelligence, volume 9329 of
Lecture Notes in Computer Science, pages 89–100.
Springer International Publishing, Cham.
Cupek, R., Zi˛ebi
´
nski, A., Drewniak, M., and Fojcik, M.
(2018). Improving kpi based performance analysis in
discrete, multi-variant production. In Nguyen, N. T.,
Hoang, D. H., Hong, T.-P., Pham, H., and Trawi
´
nski,
B., editors, Intelligent Information and Database Sys-
tems, volume 10752 of Lecture Notes in Computer
Science, pages 661–673. Springer International Pub-
lishing, Cham.
Erhan, D., Szegedy, C., Toshev, A., and Anguelov, D.
(2014). Scalable object detection using deep neural
networks. In Proceedings of the IEEE conference on
computer vision and pattern recognition, pages 2147–
2154.
Faster, R. (2015). Towards real-time object detection with
region proposal networks. Advances in neural infor-
mation processing systems, page 9199.
Girshick, R. (2015). Fast r-cnn. In 2015 IEEE International
Conference on Computer Vision (ICCV), pages 1440–
1448. IEEE.
Girshick, R., Donahue, J., Darrell, T., and Malik, J. (2014).
Rich feature hierarchies for accurate object detec-
tion and semantic segmentation. In Proceedings of
the IEEE conference on computer vision and pattern
recognition, pages 580–587.
Gonzalez, S., Arellano, C., and Tapia, J. E. (2019). Deep-
blueberry: Quantification of blueberries in the wild
using instance segmentation. IEEE Access, 7:105776–
105788.
Grzechca, D., Zi˛ebi
´
nski, A., and Rybka, P. (2017). En-
hanced reliability of adas sensors based on the obser-
vation of the power supply current and neural network
application. In Nguyen, N. T., Papadopoulos, G. A.,
J˛edrzejowicz, P., Trawi
´
nski, B., and Vossen, G., ed-
itors, Computational Collective Intelligence, volume
10449 of Lecture Notes in Computer Science, pages
215–226. Springer International Publishing, Cham.
Hariharan, B., Arbeláez, P., Girshick, R., and Malik, J.
(2014). Simultaneous detection and segmentation.
In European Conference on Computer Vision, pages
297–312.
Hariharan, B., Arbelaez, P., Girshick, R., and Malik,
J. (2017). Object instance segmentation and fine-
grained localization using hypercolumns. IEEE trans-
actions on pattern analysis and machine intelligence,
39(4):627–639.
He, K., Gkioxari, G., Dollár, P., and Girshick, R. (2017).
Mask r-cnn. In Proceedings of the IEEE international
conference on computer vision, pages 2961–2969.
He, K., Gkioxari, G., Dollar, P., and Girshick, R. (2020).
Mask r-cnn. IEEE transactions on pattern analysis
and machine intelligence, 42(2):386–397.
He, K., Zhang, X., Ren, S., and Sun, J. (2015). Spatial pyra-
mid pooling in deep convolutional networks for visual
recognition. IEEE transactions on pattern analysis
and machine intelligence, 37(9):1904–1916.
Kang, J. and Gwak, J. (2019). Ensemble of in-
stance segmentation models for polyp segmentation in
colonoscopy images. IEEE Access, 7:26440–26447.
Liang, S. Y., Hecker, R. L., and Landers, R. G. (2004). Ma-
chining process monitoring and control: the state-of-
the-art. J. Manuf. Sci. Eng., 126(2):297–310.
Liu, L., Zheng, F., Zhu, L., Li, Y., Huan, K., Shi, X.,
and Liu, G. (2015). Luminance uniformity of inte-
grating sphere light source. In 2015 International
Conference on Optoelectronics and Microelectronics
(ICOM), pages 265–268.
Liu, W., Anguelov, D., Erhan, D., Szegedy, C., Reed, S.,
Fu, C.-Y., and Berg, A. C. (2016). Ssd: Single shot
multibox detector. 9905:21–37.
Nur Ömero
˘
glu, A., Kumbasar, N., Argun Oral, E., and
Ozbek, I. Y. (2019). Mask r-cnn algoritması ile hangar
tespiti hangar detection with mask r-cnn algorithm.
27th Signal Processing and Communications Appli-
cations Conference (SIU), 31(7):1–4.
Pinheiro, P. O., Collobert, R., and Dollar, P. (2015). Learn-
ing to segment object candidates.
Pinheiro, P. O., Lin, T.-Y., Collobert, R., and Dollàr, P.
(2016). Learning to refine object segments.
Redmon, J., Divvala, S., Girshick, R., and Farhadi, A.
(2016). You only look once: Unified, real-time object
detection. In Proceedings of the IEEE conference on
computer vision and pattern recognition, pages 779–
788.
Ren, S., He, K., Girshick, R., and Sun, J. (2015). Faster
r-cnn: Towards real-time object detection with region
proposal networks. Advances in neural information
processing systems, 28:91–99.
Ren, S., He, K., Girshick, R., and Sun, J. (2017). Faster
r-cnn: Towards real-time object detection with re-
gion proposal networks. IEEE transactions on pattern
analysis and machine intelligence, 39(6):1137–1149.
Rumelhart, D. E., Hinton, G. E., and Williams, R. J. (1986).
Learning representations by back-propagating errors.
nature, 323(6088):533–536.
Schulz, H. and Moriwaki, T. (1992). High-speed machin-
ing. CIRP annals, 41(2):637–643.
Sermanet, P., Eigen, D., Zhang, X., Mathieu, M., Fergus,
R., and LeCun, Y. (2014). Overfeat: Integrated recog-
nition, localization and detection using convolutional
networks. 2nd international conference on learning
representations, iclr 2014. In 2nd International Con-
ference on Learning Representations, ICLR 2014.
Short, M. and Twiddle, J. (2019). An industrial digitaliza-
tion platform for condition monitoring and predictive
High Resolution Mask R-CNN-based Damage Detection on Titanium Nitride Coated Milling Tools for Condition Monitoring by using a
New Illumination Technique
313