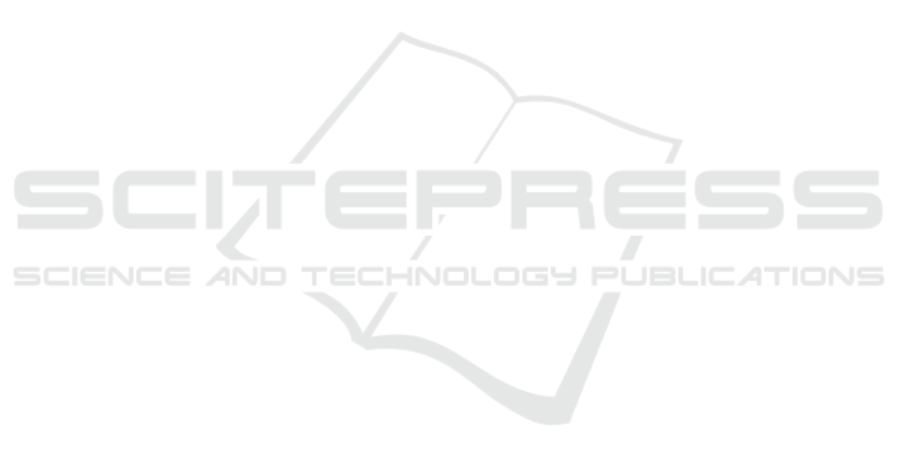
Cui, L., Zhu, W., Tao, S., Case, J., Bodenreider, O., and
Zhang, G.-Q. (2017). Mining non-lattice subgraphs
for detecting missing hierarchical relations and con-
cepts in snomed ct. Journal of the American Medical
Informatics Association : JAMIA, 24:788 – 798.
Damme, P., Quesada-Mart
´
ınez, M., Cornet, R., and
Fern
´
andez-breis, J. (2018). From lexical regulari-
ties to axiomatic patterns for the quality assurance of
biomedical terminologies and ontologies. Journal of
biomedical informatics, 84:59–74.
Dernoncourt, F., Lee, J. Y., and Szolovits, P. (2017).
Neuroner: an easy-to-use program for named-entity
recognition based on neural networks. In EMNLP.
Dogan, R., Leaman, R., and Lu, Z. (2014). Ncbi disease
corpus: A resource for disease name recognition and
concept normalization. Journal of biomedical infor-
matics, 47:1–10.
Friedman, C., Shagina, L., Lussier, Y., and Hripcsak, G.
(2004). Research paper: Automated encoding of clin-
ical documents based on natural language processing.
Journal of the American Medical Informatics Associ-
ation : JAMIA, 11 5:392–402.
Ghiasvand, O. and Kate, R. J. (2018). Learning for clinical
named entity recognition without manual annotations.
Informatics in Medicine Unlocked, 13:122–127.
Habibi, M., Weber, L., Neves, M., Wiegandt, D., and Leser,
U. (2017). Deep learning with word embeddings im-
proves biomedical named entity recognition. Bioin-
formatics, 33:i37 – i48.
Hina, S., Atwell, E., and Johnson, O. (2010). Secure in-
formation extraction from clinical documents using
snomed ct gazetteer and natural language processing.
2010 International Conference for Internet Technol-
ogy and Secured Transactions, pages 1–5.
IHTSDO (2021). SNOMED International. last accessed
24/08/2021.
Ina, S., Twell, E. R. A., and Ohnson, O. W. J. (2013).
Snomedtagger : A semantic tagger for medical nar-
ratives.
Jonquet, C., Shah, N., and Musen, M. (2009a). The open
biomedical annotator. Summit on Translational Bioin-
formatics, 2009:56 – 60.
Jonquet, C., Shah, N., Youn, C., Musen, M., Callendar, C.,
and Storey, M. (2009b). Ncbo annotator : Semantic
annotation of biomedical data.
Ju, Z., Wang, J., and Zhu, F. (2011). Named entity recogni-
tion from biomedical text using svm. 2011 5th Inter-
national Conference on Bioinformatics and Biomedi-
cal Engineering, pages 1–4.
Keretna, S., Lim, C., Creighton, D., and Shaban, K. (2015).
Enhancing medical named entity recognition with an
extended segment representation technique. Com-
puter methods and programs in biomedicine, 119
2:88–100.
Kocaman, V. and Talby, D. (2021). Spark nlp: Natu-
ral language understanding at scale. Softw. Impacts,
8:100058.
Kulick, S., Bies, A., Liberman, M., Mandel, M. A., McDon-
ald, R. T., Palmer, M., Schein, A., Ungar, L., Winters,
S., and White, P. S. (2004). Integrated annotation for
biomedical information extraction. In HLT-NAACL
2004.
Lample, G., Ballesteros, M., Subramanian, S., Kawakami,
K., and Dyer, C. (2016). Neural architectures for
named entity recognition. In NAACL.
Lee, J., Yoon, W., Kim, S., Kim, D., Kim, S., So, C. H.,
and Kang, J. (2020). Biobert: a pre-trained biomedi-
cal language representation model for biomedical text
mining. Bioinformatics, 36:1234 – 1240.
Lee, W., Kim, K., Lee, E. Y., and Choi, J. (2018). Condi-
tional random fields for clinical named entity recogni-
tion: A comparative study using korean clinical texts.
Computers in biology and medicine, 101:7–14.
Liu, X., Zhou, Y., and Wang, Z. (2019). Recognition and ex-
traction of named entities in online medical diagnosis
data based on a deep neural network. J. Vis. Commun.
Image Represent., 60:1–15.
Long, W. (2005). Extracting diagnoses from discharge sum-
maries. AMIA ... Annual Symposium proceedings.
AMIA Symposium, pages 470–4.
Luo, Y.-F., Sun, W., and Rumshisky, A. (2019). Mcn: A
comprehensive corpus for medical concept normaliza-
tion. Journal of biomedical informatics, 92:103132.
Mahi, M. (2019). scispaCy for Bio-medical Named Entity
Recognition. last accessed 30/08/2021.
Ogren, P. V., Savova, G., and Chute, C. (2008). Construct-
ing evaluation corpora for automated clinical named
entity recognition. In LREC.
Ohta, T., Tateisi, Y., and Kim, J.-D. (2002). The genia cor-
pus: an annotated research abstract corpus in molecu-
lar biology domain.
Pacheco, E., Stenzhorn, H., Nohama, P., Paetzold, J., and
Schulz, S. (2009). Detecting underspecification in
snomed ct concept definitions through natural lan-
guage processing. AMIA ... Annual Symposium pro-
ceedings. AMIA Symposium, 2009:492–6.
Pakhomov, S. V. S., Coden, A., and Chute, C. (2006). De-
veloping a corpus of clinical notes manually annotated
for part-of-speech. International journal of medical
informatics, 75 6:418–29.
P
´
erez, A., Weegar, R., Casillas, A., Gojenola, K., Oronoz,
M., and Dalianis, H. (2017). Semi-supervised medical
entity recognition: A study on spanish and swedish
clinical corpora. Journal of biomedical informatics,
71:16–30.
Pyysalo, S., Ginter, F., Moen, H., Salakoski, T., and Ana-
niadou, S. (2013). Distributional semantics resources
for biomedical text processing.
Rais, M., Lachkar, A., Lachkar, A., and Ouatik, S. A.
(2014). A comparative study of biomedical named en-
tity recognition methods based machine learning ap-
proach. 2014 Third IEEE International Colloquium
in Information Science and Technology (CIST), pages
329–334.
Rector, A. and Iannone, L. (2012). Lexically suggest, logi-
cally define: Quality assurance of the use of qualifiers
and expected results of post-coordination in snomed
ct. Journal of biomedical informatics, 45 2:199–209.
Rector, A. L., Iannone, L., and Stevens, R. (2011). Quality
assurance of the content of a large dl-based terminol-
A Novel Atomic Annotator for Quality Assurance of Biomedical Ontologies
43