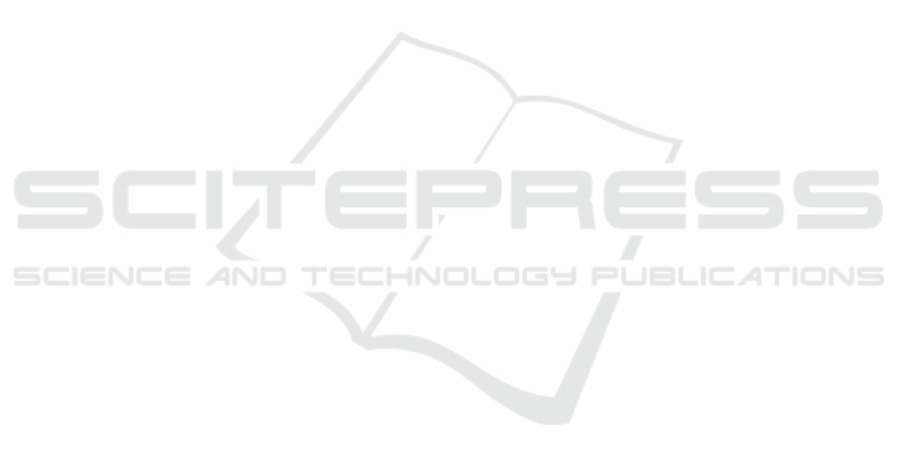
quential hBCI system as the least erroneous and the
mixed hBCI as the most erroneous. This correlates
with the quantitative results discussed earlier.
Users perceived the flickering stimuli of the hy-
brid systems to be less than that of the SSVEP-based
BCI. This may be attributed to the fact that the hybrid
systems makes use of four flickering stimuli instead
of eight. In fact, users found the hybrid systems to be
less tiring than the SSVEP-based BCI. Overall users
agreed that the time taken to make a selection was ap-
propriate for all systems. With regards to smart home
system features, all subjects stated that the menu in-
terface was easy to get used to and that it was adequate
for a smart home system.
5 CONCLUSIONS
This work concluded that eye movements can be clas-
sified reliably into horizontal or vertical eye move-
ments, using frontal EEG channels, with an accu-
racy of 98.47%. For the second tier in the proposed
hierarchical structure, classification into left, right,
up or down eye movements reached an accuracy of
74.38%. Finally, the eye movements could be clas-
sified into the eight considered saccadic gaze angle,
with an accuracy of 58.31%. These results compare
well with the limited literature in this field. Specifi-
cally (Dietriech M. P., 2017) had obtained a classifi-
cation of 96.6% when classifying between 4 extreme
eye movements (up, down, left, right) and the central
location using a kNN classifier, and 58.4% when us-
ing a linear SVM classifier. This however was only
based on a single subject as opposed to our work
which was validated on 5 subjects.
The results presented in this paper also show that
reliable eye-movement information may also be ex-
tracted using only the occipital EEG channels, though
with lower accuracies. Specifically classification into
horizontal or vertical eye movements reached an ac-
curacy of 71.11% while classification in the second
and third tiers of the proposed hierarchical structure
dropped to 43.85% and 28.02% respectively. How-
ever, if three frontal channels are added to the occipi-
tal channels vertical and horizontal eye movements at
large visual angles may be distinguished with an ac-
curacy close to 80% using the proposed hierarchical
classifier.
These results were then used for the develop-
ment of a smart home automation system where eye
movement information occurring prior to the visu-
ally evoked potential was exploited to improve clas-
sification performance of a hybrid BCI. An offline
study showed that a sequential hBCI gave an aver-
age accuracy over 5 subjects of 82.5% and an ITR
of 19.06bpm, while the mixed hBCI and the SSVEP-
based BCI gave an accuracy of 64.16% and 69.16%
respectively, and an ITR of 11.32bpm and 16.17bpm
respectively. The results of the online automation sys-
tem also confirmed that users performed better with
the sequential hBCI and users found this more intu-
itive to control. These results show that eye move-
ment information extracted from standard EEG chan-
nels typically used in an SSVEP based BCI can pro-
vide relevant information which improves the classi-
fication performance, especially for subjects whose
SSVEP response is not very strong.
ACKNOWLEDGEMENTS
This work was partially supported through the Project
R&I-2015-032-T ‘BrainApp’ financed by the Malta
Council for Science & Technology, for and on be-
half of the Foundation for Science and Technology,
through the FUSION: R&I Technology Development
Programme.
REFERENCES
Belkacem, A., Hirose, H., Yoshimura, N., Shin, D., and
Koike, Y. (2013). Classification of four eye directions
from eeg signals for eye-move-based communication
system. J Med Biol Eng, 34:581–588.
Belkacem, A., Saetia, S., Zintus-art, K., Shin, D., Kam-
bara, H., Yoshimura, N., Berrached, N., and Koike,
Y. (2015). Real-time control of a video game using
eye-movements and two temporal eeg sensors. Com-
putational Intelligence and Neuroscience.
Bishop, C. (2006). Pattern Recognition and Machine
Learning. Springer.
Chen, X., Wang, Y., Gao, S., Jung, T., and Gao, X. (2015).
Filter bank canonical correlation analysis for imple-
menting a high-speed ssvep-based brain-computer in-
terface. Journal of Neural Engineering, 12(4).
Dietriech M. P., Winterfeldt G., V. M. S. (2017). Towards
eeg-based eye-tracking for interaction design in head-
mounted devices. 7th International Conference on
Consumer Electronics (ICCE).
Gupta, S., Soman, S., Raj, P., Prakash, R., Sailaja, S., and
Borgohain, R. (2012). Detecting of eye movements in
eeg for controlling devices. IEEE Int. Conf. on Com-
putational Intelligence & Cybernetics.
Hsieh, C., Chu, H., and Huang, Y. (2014). An hmm-based
eye movement detection system using eeg brain-
computer interface. IEEE International Symposium
on Circuits and System.
Johannes M. G., Pfurtscheller G., F. H. (1999). Design-
ing optimal spatial filters for single-trial eeg classifi-
BIOSIGNALS 2022 - 15th International Conference on Bio-inspired Systems and Signal Processing
126