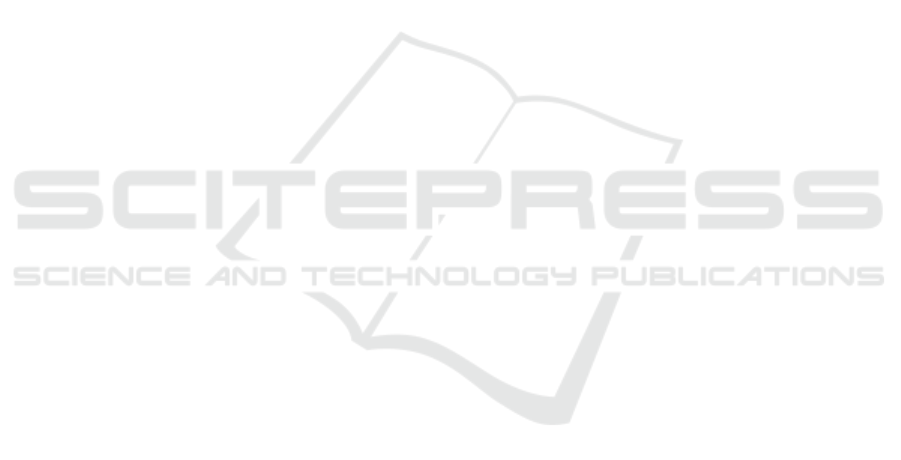
created. As a result, the network is more capable of
distinguishing normal from abnormal examples. The
application of SMOTE techniques boosted the CNN
model in overall by 0.71%.
7 CONCLUSIONS AND FUTURE
WORK
In this paper, the problematic concerning to the short-
age of abnormal heart sound examples is studied and
addressed. We proposed the usage of SMOTE tech-
niques to generate synthetic spectrogram images. The
best result was achieved by a SMOTE-CNN algo-
rithm, an overall of 88.43%, a Sensitivity of 84.51%
and a Specificity of 92.34%.
The current results, strongly indicate that the ap-
plication of oversampling techniques, such as the
SMOTE, can improve significantly the capability of
CNN model’s to discriminate between normal and ab-
normal heart beats. The proposed SMOTE approach
can serve as a basis for other unbalanced dataset prob-
lems besides heart sounds problems.
For future work we intend to extend out experi-
ments to pre-trained models. We also intend explore
other oversampling techniques.
ACKNOWLEDGEMENTS
This work is financed by National Funds through
the Portuguese funding agency, FCT-Fundac¸
˜
ao
para a Ci
ˆ
encia e a Tecnologia, within project
UIDB/50014/2020.
REFERENCES
Banerjee, M. and Majhi, S. (2020). Multi-class heart sounds
classification using 2d-convolutional neural network.
In 2020 5th International Conference on Computing,
Communication and Security (ICCCS), pages 1–6.
Baydoun, M., Safatly, L., Ghaziri, H., and El-Hajj, A.
(2020). Analysis of heart sound anomalies using en-
semble learning. Biomed. Signal Process. Control.,
62:102019.
Boulares, M., Alafif, T., and Barnawi, A. (2020). Transfer
learning benchmark for cardiovascular disease recog-
nition. IEEE Access, 8:109475–109491.
Dornbush S, T. A. (2021). Physiology, heart sounds.
Khan, K. N., Khan, F. A., Abid, A., Olmez, T., Dokur,
Z., Khandakar, A., Chowdhury, M. E. H., and Khan,
M. S. (2021). Deep learning based classification of un-
segmented phonocardiogram spectrograms leveraging
transfer learning.
Koike, T., Qian, K., Kong, Q., Plumbley, M. D., Schuller,
B. W., and Yamamoto, Y. (2020). Audio for audio is
better? an investigation on transfer learning models
for heart sound classification. In 2020 42nd Annual
International Conference of the IEEE Engineering in
Medicine Biology Society (EMBC), pages 74–77.
Latif, S., Usman, M., Rana, R., and Qadir, J. (2018).
Phonocardiographic sensing using deep learning for
abnormal heartbeat detection. IEEE Sensors Journal,
18(22):9393–9400.
Libby, P., Bonow, R., Mann, D., and Zipes, D. (2007).
Braunwald’s Heart Disease: A Textbook of Cardio-
vascular Medicine. 8th edition. Elsevier Science.
MA., C. (2008). Cardiac auscultation: rediscovering the
lost art. Curr Probl Cardiol., 7(33):326–408.
Mustafa, M. e. a. (2020). Detection of heartbeat sounds
arrhythmia using automatic spectral methods and
cardiac auscultatory. Journal of Supercomputing,
76(8):5899–5922.
Nogueira, D. M., Ferreira, C. A., Gomes, E. F., and Jorge,
A. M. (2019). Classifying heart sounds using images
of motifs, mfcc and temporal features. J. Med. Syst.,
43(6).
Potes, C., Parvaneh, S., Rahman, A., and Conroy, B. (2016).
Ensemble of feature-based and deep learning-based
classifiers for detection of abnormal heart sounds. In
2016 Computing in Cardiology Conference (CinC),
pages 621–624.
Prodoctor2019 (2020). Estetosc
´
opio eletr
ˆ
onico: tudo o que
voc
ˆ
e precisa saber.
Singh, M. and Cheema, A. (2013). Heart sounds classifi-
cation using feature extraction of phonocardiography
signal. International Journal of Computer Applica-
tions, 4(77):13–17.
WHO (2020). World health organization (2020) cardiovas-
cular diseases (cvds), cardiovascular diseases (cvds).
Zabihi, M., Rad, A. B., Kiranyaz, S., Gabbouj, M., and Kat-
saggelos, A. K. (2016). Heart sound anomaly and
quality detection using ensemble of neural networks
without segmentation. In 2016 Computing in Cardi-
ology Conference (CinC), pages 613–616.
BIOSIGNALS 2022 - 15th International Conference on Bio-inspired Systems and Signal Processing
132