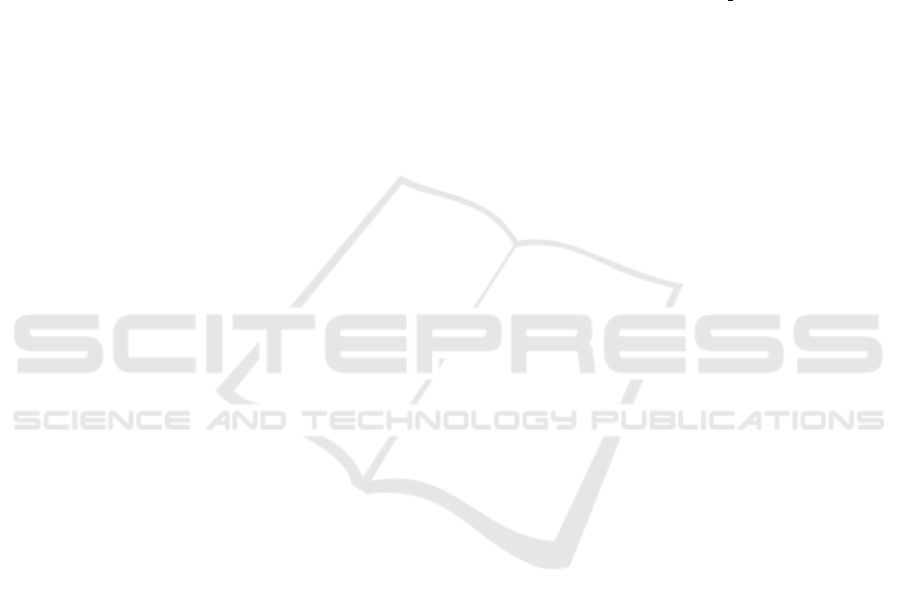
Cook, D. A. and Triola, M. M. (2009). Virtual patients:
a critical literature review and proposed next steps.
Medical Education, 43(4):303–311.
Crichton, G., Pyysalo, S., Chiu, B., and Korhonen, A.
(2017). A neural network multi-task learning ap-
proach to biomedical named entity recognition. BMC
bioinformatics, 18(1):1–14.
Dafli, E., Antoniou, P., Ioannidis, L., Dombros, N., Topps,
D., and Bamidis, P. D. (2015). Virtual patients on the
semantic web: a proof-of-application study. Journal
of medical Internet research, 17(1):e3933.
de Araujo Guerra Grangeia, T., de Jorge, B., Franci,
D., Martins Santos, T., Vellutini Setubal, M. S.,
Schweller, M., and de Carvalho-Filho, M. A. (2016).
Cognitive load and self-determination theories applied
to e-learning: impact on students’ participation and
academic performance. PloS one, 11(3):e0152462.
de Menezes Mota, M. F., Pantoja, F. L., Mota, M. S.,
Grangeia, T. d. A. G., de Carvalho Filho, M. A., and
Santanch
`
e, A. (2019). Analytical design of clinical
cases for educational games. In Joint International
Conference on Entertainment Computing and Serious
Games, pages 353–365. Springer.
Devlin, J., Chang, M.-W., Lee, K., and Toutanova, K.
(2018). Bert: Pre-training of deep bidirectional trans-
formers for language understanding. arXiv preprint
arXiv:1810.04805.
Do
˘
gan, R. I. and Lu, Z. (2012). An improved corpus of
disease mentions in PubMed citations. In Proc. 2012
Workshop on Biomedical Natural Language Process-
ing, pages 91–99.
Do
˘
gan, R. I., Leaman, R., and Lu, Z. (2014). Ncbi disease
corapus: a resource for disease name recognition and
concept normalization. Journal of biomedical infor-
matics, 47:1–10.
Hege, I., Kononowicz, A. A., and Adler, M. (2017). A clin-
ical reasoning tool for virtual patients: design-based
research study. JMIR medical education, 3(2):e8100.
Jackson, C. B., Østerlund, C., Crowston, K., Harandi, M.,
and Trouille, L. (2020). Shifting forms of engage-
ment: Volunteer learning in online citizen science.
Proceedings of the ACM on Human-Computer Inter-
action, 4(CSCW1):1–19.
Kim, D., Lee, J., So, C. H., Jeon, H., Jeong, M., Choi, Y.,
Yoon, W., Sung, M., and Kang, J. (2019). A neural
named entity recognition and multi-type normaliza-
tion tool for biomedical text mining. IEEE Access,
7:73729–73740.
Lee, J., Yoon, W., Kim, S., Kim, D., Kim, S., So, C. H.,
and Kang, J. (2020). Biobert: a pre-trained biomedi-
cal language representation model for biomedical text
mining. Bioinformatics, 36(4):1234–1240.
Li, J., Sun, Y., Johnson, R. J., Sciaky, D., Wei, C.-H.,
Leaman, R., Davis, A. P., Mattingly, C. J., Wiegers,
T. C., and Lu, Z. (2016). Biocreative v cdr task cor-
pus: a resource for chemical disease relation extrac-
tion. Database, 2016.
Lyu, Y. and Zhong, J. (2021). Dsmer: A deep semantic
matching based framework for named entity recog-
nition. In European Conference on Information Re-
trieval, pages 419–432. Springer.
Miftahutdinov, Z., Kadurin, A., Kudrin, R., and Tutubalina,
E. (2021). Drug and disease interpretation learn-
ing with biomedical entity representation transformer.
arXiv preprint arXiv:2101.09311.
Miles, A. and Bechhofer, S. (2009). SKOS Simple Knowl-
edge Organization System Reference. Technical re-
port.
Mosbach, M., Andriushchenko, M., and Klakow, D. (2020).
On the stability of fine-tuning bert: Misconceptions,
explanations, and strong baselines. arXiv preprint
arXiv:2006.04884.
Noy, N. F., Shah, N. H., Whetzel, P. L., Dai, B., Dorf, M.,
Griffith, N., Jonquet, C., Rubin, D. L., Storey, M.-A.,
Chute, C. G., et al. (2009). Bioportal: ontologies and
integrated data resources at the click of a mouse. Nu-
cleic acids research, 37(suppl 2):W170–W173.
Pyysalo, S. and Ananiadou, S. (2014). Anatomical entity
mention recognition at literature scale. Bioinformat-
ics, 30(6):868–875.
Schreiber, G. and Raimond, Y. (2014). RDF 1.1 Primer.
Technical report.
Vaswani, A., Shazeer, N., Parmar, N., Uszkoreit, J., Jones,
L., Gomez, A. N., Kaiser, L., and Polosukhin, I.
(2017). Attention is all you need. arXiv preprint
arXiv:1706.03762.
Velupillai, S., Suominen, H., Liakata, M., Roberts, A.,
Shah, A. D., Morley, K., Osborn, D., Hayes, J., Stew-
art, R., Downs, J., et al. (2018). Using clinical natu-
ral language processing for health outcomes research:
overview and actionable suggestions for future ad-
vances. Journal of biomedical informatics, 88:11–19.
Harena Semantics: A Framework to Support Semantic Annotation in Citizen Science Systems
343