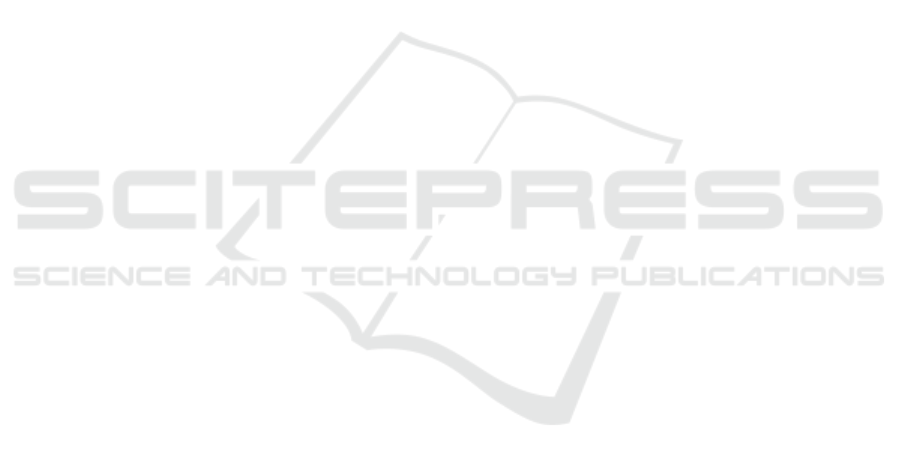
equalization for image contrast enhancement. IEEE
Transactions on Consumer Electronics, 53(2):593–
600.
Akcay, S. and Breckon, T. (2021). Towards automatic
threat detection: A survey of advances of deep learn-
ing within x-ray security imaging. Pattern Recogni-
tion, 122:108245.
C¸ allı, E., Sogancioglu, E., van Ginneken, B., van Leeuwen,
K. G., and Murphy, K. (2021). Deep learning for chest
x-ray analysis: A survey. Medical Image Analysis,
72:102125.
Alloqmani, A., B., Y., Khan, A., and Alsolami, F. (2021).
Deep learning based anomaly detection in images:
Insights, challenges and recommendations. Interna-
tional Journal of Advanced Computer Science and Ap-
plications, 12.
Cohen, J. P., Morrison, P., Dao, L., Roth, K., Duong, T. Q.,
and Ghassemi, M. (2020). Covid-19 image data col-
lection: Prospective predictions are the future. arXiv
2006.11988.
Elgendi, M., Nasir, M. U., Tang, Q., Smith, D., Grenier, J.-
P., Batte, C., Spieler, B., Leslie, W. D., Menon, C.,
Fletcher, R. R., Howard, N., Ward, R., Parker, W.,
and Nicolaou, S. (2021). The effectiveness of image
augmentation in deep learning networks for detect-
ing covid-19: A geometric transformation perspec-
tive. Frontiers in Medicine, 8:153.
Everingham, M., Gool, L. V., Williams, C. K. I., Winn, J.,
and Zisserman, A. (2010). The pascal visual object
classes (voc) challenge.
He, K., Zhang, X., Ren, S., and Sun, J. (2015). Deep
residual learning for image recognition. CoRR,
abs/1512.03385.
Ho, Y. and Wookey, S. (2020). The real-world-weight cross-
entropy loss function: Modeling the costs of mislabel-
ing. CoRR, abs/2001.00570.
Hussain, M., Bird, J. J., and Faria, D. R. (2019). A study on
cnn transfer learning for image classification. In Lotfi,
A., Bouchachia, H., Gegov, A., Langensiepen, C., and
McGinnity, M., editors, Advances in Computational
Intelligence Systems, pages 191–202, Cham. Springer
International Publishing.
Iandola, F. N., Moskewicz, M. W., Ashraf, K., Han, S.,
Dally, W. J., and Keutzer, K. (2016). Squeezenet:
Alexnet-level accuracy with 50x fewer parameters and
<1mb model size. CoRR, abs/1602.07360.
Itseez (2015). Open source computer vision library.
Jiang, Z. (2020). Chest x-ray pneumonia detection based
on convolutional neural networks. In 2020 Interna-
tional Conference on Big Data, Artificial Intelligence
and Internet of Things Engineering (ICBAIE), pages
341–344.
Krizhevsky, A., Sutskever, I., and Hinton, G. E. (2012).
Imagenet classification with deep convolutional neu-
ral networks. In Pereira, F., Burges, C. J. C., Bottou,
L., and Weinberger, K. Q., editors, Advances in Neu-
ral Information Processing Systems 25, pages 1097–
1105. Curran Associates, Inc.
Lee, S., il Oh, S., and Jo, J. (2021). Deep learning for early
dental caries detection in bitewing radiographs. Na-
ture Scientific Reports.
Mooney, P. (2018). Chest x-ray images (pneumonia).
Paszke, A., Gross, S., Massa, F., Lerer, A., Bradbury, J.,
Chanan, G., Killeen, T., Lin, Z., Gimelshein, N.,
Antiga, L., Desmaison, A., K
¨
opf, A., Yang, E., De-
Vito, Z., Raison, M., Tejani, A., Chilamkurthy, S.,
Steiner, B., Fang, L., Bai, J., and Chintala, S. (2019).
Pytorch: An imperative style, high-performance deep
learning library. CoRR, abs/1912.01703.
Rahman, T., Chowdhury, M. E. H., Khandakar, A., Islam,
K. R., Islam, K. F., Mahbub, Z. B., Kadir, M. A., and
Kashem, S. (2020). Transfer learning with deep con-
volutional neural network (cnn) for pneumonia detec-
tion using chest x-ray. Applied Sciences, 10(9).
Russakovsky, O., Deng, J., Su, H., Krause, J., Satheesh, S.,
Ma, S., Huang, Z., Karpathy, A., Khosla, A., Bern-
stein, M. S., Berg, A. C., and Li, F. (2014). Ima-
genet large scale visual recognition challenge. CoRR,
abs/1409.0575.
Szegedy, C., Vanhoucke, V., Ioffe, S., Shlens, J., and Wojna,
Z. (2015). Rethinking the inception architecture for
computer vision. CoRR, abs/1512.00567.
Wang, K., Gao, X., Zhao, Y., Li, X., Dou, D., and Xu, C.-Z.
(2019). Pay attention to features - transfer learn faster.
Wang, S. and Zhang, Y.-D. (2020). Densenet-201-based
deep neural network with composite learning factor
and precomputation for multiple sclerosis classifica-
tion. ACM Transactions on Multimedia Computing,
Communications, and Applications, 16:1–19.
Wang, X., Peng, Y., Lu, L., Lu, Z., Bagheri, M., and Sum-
mers, R. M. (2017). Chestx-ray8: Hospital-scale chest
x-ray database and benchmarks on weakly-supervised
classification and localization of common thorax dis-
eases. CoRR, abs/1705.02315.
Younesian, T., Hong, C., Ghiassi, A., Birke, R., and Chen,
L. Y. (2020). End-to-end learning from noisy crowd
to supervised machine learning models.
VISAPP 2022 - 17th International Conference on Computer Vision Theory and Applications
330