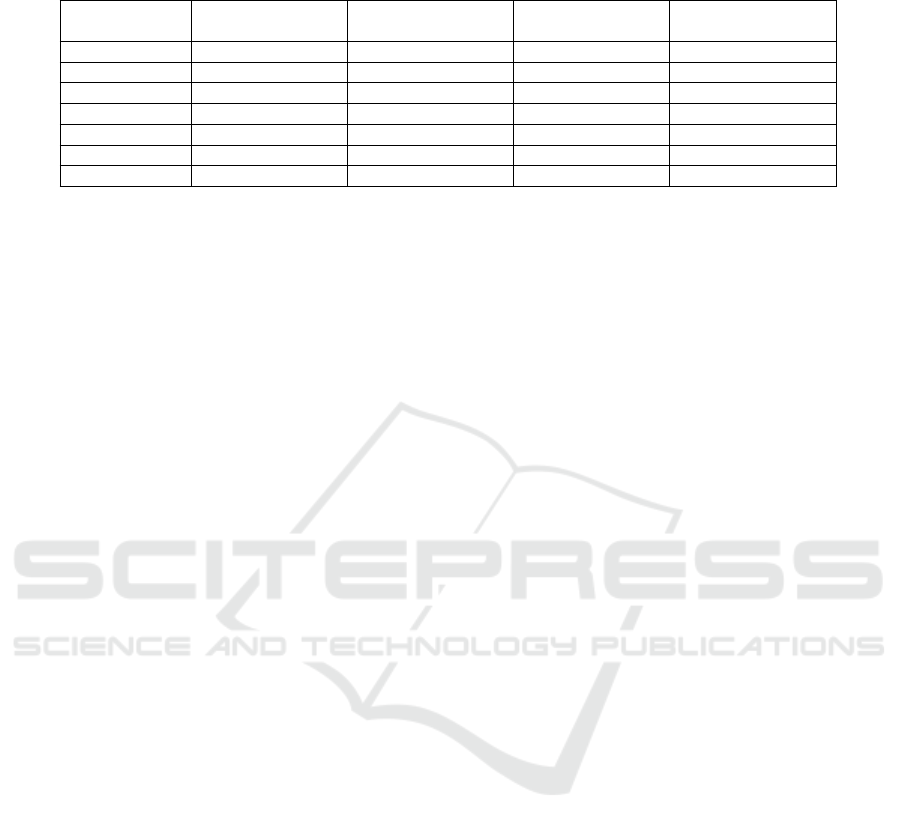
Table 6: Accuracy of individual participants when machine learning algorithm predicted correctly or incorrectly QoL (quality
of life) class for the patients. Avg. = Average, CI = Confidence interval.
With aid Without aid
Clinician Correct prediction Incorrect prediction Correct prediction Incorrect prediction
1 .765 .667 .647 .667
2 .846 .429 .846 .429
3 .722 .000 .667 .000
4 .833 1.000 .722 1.000
5 .824 .333 .824 .667
6 .769 .429 .615 .429
Avg. (95% CI) .793 (.754-.832) .476 (.208-.745) .720 (.644-.797) .532 (.264-.799)
diction would be most useful for the time point 12
months after the end of treatment, as more variance in
patients’ resilience is often observed at this point in
time.
As a follow-up study, the effect of the clinician’s
experience and test environment for the performance
should also be investigated. Previous research (Cai
et al., 2019) has shown that the aid of machine learn-
ing benefits more inexperienced clinicians. All partic-
ipants in this study were experienced clinicians. That
is, with inexperienced clinicians benefits from the aid
of machine learning predictions could be higher. Test
environment of this study did not correspond to a real
clinical environment. There were no unrelated dis-
tractions or other examinations requiring the attention
of clinicians. In noisy real clinical environment, the
aid of machine learning predictions can be higher that
should be studied.
5 CONCLUSIONS
Based on the study, the machine learning model in-
tegrated into the CDSS improved clinicians’ perfor-
mance in predicting patients’ quality of life after six
months from the baseline. Performance improved
especially in the cases where the machine learning
model was able to correctly predict patient’s QoL
value. It should be noted, however, that based on the
open-ended interview, this kind of tool is considered
useful only when resilience strengthening interven-
tions can be implemented for the patients identified
to have low predicted resilience
REFERENCES
Aaronson, N. K., Ahmedzai, S., Bergman, B., Bullinger,
M., Cull, A., Duez, N. J., Filiberti, A., Flechtner, H.,
Fleishman, S. B., and de Haes, J. C. (1993). The Eu-
ropean Organization for Research and Treatment of
Cancer QLQ-C30: a quality-of-life instrument for use
in international clinical trials in oncology. J Natl Can-
cer Inst, 85(5):365–376.
Bonanno, G. A., Galea, S., Bucciarelli, A., and Vlahov, D.
(2007). What predicts psychological resilience after
disaster? The role of demographics, resources, and
life stress. J Consult Clin Psychol, 75(5):671–682.
Bray, F., Ferlay, J., Soerjomataram, I., Siegel, R. L., Torre,
L. A., and Jemal, A. (2018). Global cancer statistics
2018: GLOBOCAN estimates of incidence and mor-
tality worldwide for 36 cancers in 185 countries. CA
Cancer J Clin, 68(6):394–424.
Cai, S. L., Li, B., Tan, W. M., Niu, X. J., Yu, H. H.,
Yao, L. Q., Zhou, P. H., Yan, B., and Zhong, Y. S.
(2019). Using a deep learning system in endoscopy
for screening of early esophageal squamous cell car-
cinoma (with video). Gastrointest Endosc, 90(5):745–
753.
Clarke, V. and Braun, V. (2014). Thematic Analysis, vol-
ume 3, pages 1947–1952.
Deshields, T. L., Heiland, M. F., Kracen, A. C., and Dua, P.
(2016). Resilience in adults with cancer: development
of a conceptual model. Psychooncology, 25(1):11–18.
Ginestra, J. C., Giannini, H. M., Schweickert, W. D., Mead-
ows, L., Lynch, M. J., Pavan, K., Chivers, C. J.,
Draugelis, M., Donnelly, P. J., Fuchs, B. D., and
Umscheid, C. A. (2019). Clinician Perception of a
Machine Learning-Based Early Warning System De-
signed to Predict Severe Sepsis and Septic Shock. Crit
Care Med, 47(11):1477–1484.
Goebel, S. and Mehdorn, H. M. (2011). Measurement of
psychological distress in patients with intracranial tu-
mours: the NCCN distress thermometer. J Neuroon-
col, 104(1):357–364.
ITU-R (2012). Itu-r rec. bt.500-13, methodology for the
subjective assessment of the quality of television pic-
tures. Report A 70000, ITU Radiocommunication
Sector.
Jang, S., Song, H., Shin, Y. J., Kim, J., Kim, J., Lee, K. W.,
Lee, S. S., Lee, W., Lee, S., and Lee, K. H. (2020).
Deep Learning-based Automatic Detection Algorithm
for Reducing Overlooked Lung Cancers on Chest Ra-
diographs. Radiology, 296(3):652–661.
Kiani, A., Uyumazturk, B., Rajpurkar, P., Wang, A., Gao,
R., Jones, E., Yu, Y., Langlotz, C. P., Ball, R. L.,
Montine, T. J., Martin, B. A., Berry, G. J., Ozawa,
M. G., Hazard, F. K., Brown, R. A., Chen, S. B.,
Wood, M., Allard, L. S., Ylagan, L., Ng, A. Y., and
Shen, J. (2020). Impact of a deep learning assistant on
the histopathologic classification of liver cancer. NPJ
Digit Med, 3:23.
Impact of Machine Learning Assistance on the Quality of Life Prediction for Breast Cancer Patients
351