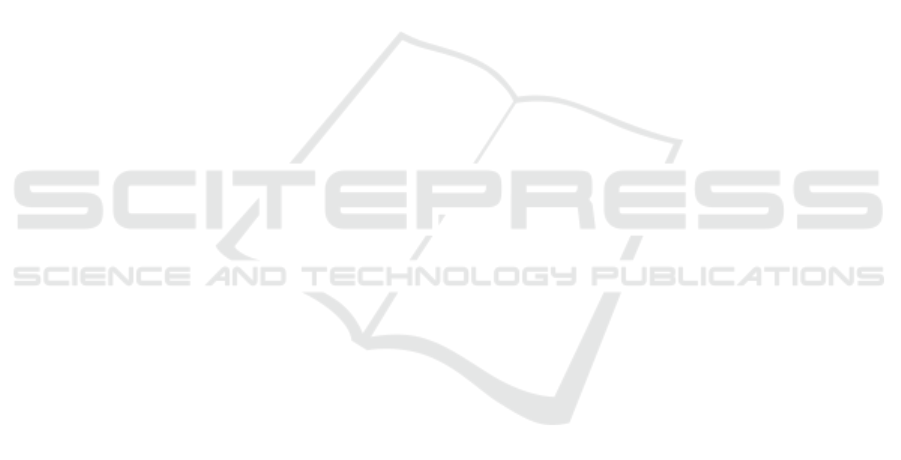
ACKNOWLEDGEMENTS
The work was funded by Russian Foundation of Basic
Research grant No. 20-01-00664.
REFERENCES
Apyari, V., Tiras, K., Nefedova, S., and Gorbunova, M.
(2021). Non-invasive in vivo spectroscopy using a
monitor calibrator: A case of planarian feeding and di-
gestion statuses. Microchemical Journal, 166:106255.
Bagu
˜
n
`
a, J. (2012). The planarian neoblast: The rambling
history of its origin and some current black boxes.
The International Journal of Developmental Biology,
56:19–37.
Barber, B., K
¨
uhn, D., Lo, A., Osthus, D., and Taylor, A.
(2017). Clique decompositions of multipartite graphs
and completion of latin squares. Journal of Combina-
torial Theory, Series A, 151:146–201.
Bay, H., Ess, A., Tuytelaars, T., and Gool, L. V. (2008).
Speeded-up robust features (surf). Computer Vision
and Image Understanding, 110(3):346–359. Similar-
ity Matching in Computer Vision and Multimedia.
Clapham, M., Miller, E., Nguyen, M., and Darimont, C.
(2020). Automated facial recognition for wildlife
that lack unique markings: A deep learning approach
for brown bears. Ecology and Evolution, 10:12883–
12892.
Dawande, M., Keskinocak, P., Swaminathan, J. M., and
Tayur, S. (2001). On bipartite and multipartite clique
problems. Journal of Algorithms, 41(2):388–403.
Duyck, J., Finn, C., Hutcheon, A., Vera, P., Salas, J., and
Ravela, S. (2015). Sloop: A pattern retrieval engine
for individual animal identification. Pattern Recogni-
tion, 48(4):1059–1073.
Elliott, S. A. and Alvarado, A. S. (2013). The history and
enduring contributions of planarians to the study of
animal regeneration. Wiley Interdisciplinary Reviews:
Developmental Biology, 2:301–326.
Flygare, S., Campbell, M., Ross, R. M., Moore, B., and
Yandell, M. (2013). ImagePlane: An automated im-
age analysis pipeline for high-throughput screens us-
ing the planarian Schmidtea mediterranea. Journal of
Computational Biology, 20(8):583–592.
Freytag, A., Rodner, E., Simon, M., Loos, A., K
¨
uhl, H.,
and Denzler, J. (2016). Chimpanzee faces in the wild:
Log-euclidean cnns for predicting identities and at-
tributes of primates. volume 9796, pages 51–63.
Fritsch, F. N. and Carlson, R. E. (1980). Monotone piece-
wise cubic interpolation. SIAM Journal on Numerical
Analysis, 17(2):238–246.
Hughes, B. and Burghardt, T. (2017). Automated visual fin
identification of individual great white sharks. Int. J.
Comput. Vis., 122(3):542–557.
Karami, A., Tebyaniyan, H., Gooadrzi, V., and Shiri, S.
(2015). Planarians: an in vivo model for regenera-
tive medicine. International Journal of Stem Cells,
8:128–133.
Lahiri, M., Tantipathananandh, C., Warungu, R., Ruben-
stein, D. I., and Berger-Wolf, T. Y. (2011). Biometric
animal databases from field photographs: Identifica-
tion of individual zebra in the wild. ICMR ’11, New
York, NY, USA. Association for Computing Machin-
ery.
Lee, V., Ruan, N., Jin, R., and Aggarwal, C. (2010). A
Survey of Algorithms for Dense Subgraph Discovery,
pages 303–336.
Liu, C., Zhang, R., and Guo, L. (2019). Part-pose guided
amur tiger re-identification. In 2019 IEEE/CVF In-
ternational Conference on Computer Vision Workshop
(ICCVW), pages 315–322.
Lu, X., Wang, Y., Fung, S., and Qing, X. (2021). I-nema: A
biological image dataset for nematode recognition.
Mestetskiy, L. (2000). Fat curves and representation of pla-
nar figures. Computers & Graphics, 24:9–21.
Mestetskiy, L. and Semenov, A. (2008). Binary image
skeleton — continuous approach. In Proceedings
of the Third International Conference on Computer
Vision Theory and Applications - Volume 1: VIS-
APP, (VISIGRAPP 2008), pages 251–258. INSTICC,
SciTePress.
Myronenko, A. and Song, X. (2010). Point set registra-
tion: Coherent point drift. IEEE Transactions on Pat-
tern Analysis and Machine Intelligence, 32(12):2262–
2275.
Peiris, T., Hoyer, K., and Oviedo, N. (2014). Innate im-
mune system and tissue regeneration in planarians:
An area ripe for exploration. Seminars in Immunol-
ogy, 26:295–302.
Peng, H., Long, F., Liu, X., Kim, S. K., and Myers, E. W.
(2007). Straightening caenorhabditis elegans images.
Bioinformatics, 24(2):234–242.
Qu, L. and Peng, H. (2010). A principal skeleton algorithm
for standardizing confocal images of fruit fly nervous
systems. Bioinformatics, 26(8):1091–1097.
Rao, K. K., Grabow, L. C., Mu
˜
noz-P
´
erez, J. P., Alarc
´
on-
Ruales, D., and Azevedo, R. B. R. (2021). Sea turtle
facial recognition using map graphs of scales. bioRxiv.
Sheimann, I. M. and Sakharova, N. (1974). On a peculiarity
of planarian digestion. Comparative biochemistry and
physiology, 48:601–7.
Tiras, K., Mestetskiy, L., Nefedova, S., and Lomov, N.
(2021). Registration of regeneration in planarians
from photographic images. Journal of Biomedical
Photonics & Engineering.
Tiras, K., Petrova, O., Myakisheva, S., Deev, A., and
Aslanidi, K. (2015). Minimizing of morphometric
errors in planarian regeneration. Fundamental Re-
search, 2:128–133.
Tiras, K. P., Nefedova, S., Novikov, K., Emelyanenko, V.,
and Balmashov, S. (2018). In vivo methods of control
of phago- and pinocytosis on the model of planarian
digestion. In Proceedings of the International Con-
ference ”Situational Centers and Class 4i Informa-
tion and Analytical Systems for Monitoring and Secu-
rity Tasks” (SCVRT2018), volume 48, pages 346–359.
Moscow-Protvino.
VISAPP 2022 - 17th International Conference on Computer Vision Theory and Applications
96