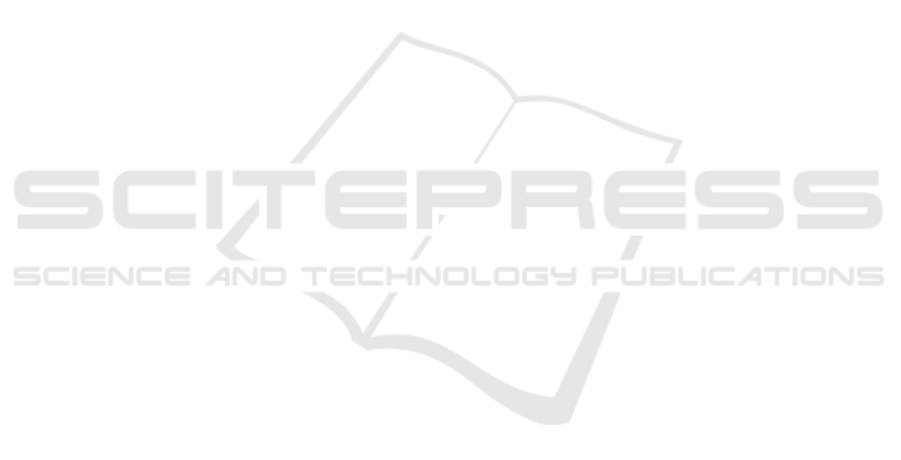
insights from cross-study comparisons and thus hav-
ing large bodies of comparable works is quintessential
to properly interpret components that influence users’
subjective evaluation.
REFERENCES
Araujo, T., Helberger, N., Kruikemeier, S., and de Vreese,
C. H. (2020). In AI we trust? perceptions about au-
tomated decision-making by artificial intelligence. AI
& SOCIETY, 35(3):611–623.
Buch, V. H., Ahmed, I., and Maruthappu, M. (2018). Arti-
ficial intelligence in medicine: current trends and fu-
ture possibilities. British Journal of General Practice,
68(668):143–144.
Cameron, G., Cameron, D. W., Megaw, G., Bond, R. R.,
Mulvenna, M., O’Neill, S. B., Armour, C., and
McTear, M. (2018). Best practices for designing chat-
bots in mental healthcare – a case study on iHelpr.
In Proceedings of the 32nd International BCS Human
Computer Interaction Conference.
Campbell, J. L. (2020). Healthcare experience design: A
conceptual and methodological framework for under-
standing the effects of usability on the access, deliv-
ery, and receipt of healthcare. Knowledge Manage-
ment & E-Learning, 12(4):505–520.
Chowriappa, P., Dua, S., and Todorov, Y. (2014). Intro-
duction to machine learning in healthcare informatics.
In Dua, S., Acharya, U. R., and Dua, P., editors, Ma-
chine Learning in Healthcare Informatics, pages 1–
23. Springer Berlin Heidelberg.
Chung, K. and Park, R. C. (2019). Chatbot-based heathcare
service with a knowledge base for cloud computing.
Cluster Computing, 22:1925–1937.
Davenport, T., Guha, A., Grewal, D., and Bressgott, T.
(2020). How artificial intelligence will change the fu-
ture of marketing. Journal of the Academy of Market-
ing Science, 48(1):24–42.
de Gennaro, M., Krumhuber, E. G., and Lucas, G. (2020).
Effectiveness of an empathic chatbot in combating ad-
verse effects of social exclusion on mood. Frontiers in
Psychology, 10:3061.
Denecke, K. and Warren, J. (2020). How to evaluate health
applications with conversational user interface? Stud-
ies in Health Technology and Informatics, 270:970–
980.
Distler, V., Lallemand, C., and Bellet, T. (2018). Accept-
ability and acceptance of autonomous mobility on de-
mand: The impact of an immersive experience. In
Proceedings of the 2018 CHI Conference on Human
Factors in Computing Systems, CHI ’18, pages 1—-
10, New York, NY, USA. Association for Computing
Machinery.
Dorsey, E. R. and Topol, E. J. (2020). Telemedicine 2020
and the next decade. The Lancet, 395(10227):859.
Dua, S., Acharya, U. R., and Dua, P., editors (2014). Ma-
chine Learning in Healthcare Informatics, volume 56
of Intelligent Systems Reference Library. Springer
Berlin Heidelberg.
Edison, S. and Geissler, G. (2003). Measuring attitudes to-
wards general technology: Antecedents, hypotheses
and scale development. Journal of Targeting, Mea-
surement and Analysis for Marketing, 12:137–156.
Gruson, D., Helleputte, T., Rousseau, P., and Gruson, D.
(2019). Data science, artificial intelligence, and ma-
chine learning: Opportunities for laboratory medicine
and the value of positive regulation. Clinical Biochem-
istry, 69:1–7.
Gustafson, P. E. (1998). Gender differences in risk per-
ception: Theoretical and methodological erspectives.
Risk Analysis, 18(6):805–811.
Hoff, K. A. and Bashir, M. (2015). Trust in automation:
Integrating empirical evidence on factors that influ-
ence trust. Human Factors, 57(3):407–434. PMID:
25875432.
Kieffer, S. (2017). Ecoval: Ecological validity of cues
and representative design in user experience evalua-
tions. AIS Transactions on Human-Computer Interac-
tion, 9(2):149–172.
Law, E. L.-C., van Schaik, P., and Roto, V. (2014). At-
titudes towards user experience (UX) measurement.
International Journal of Human-Computer Studies,
72(6):526–541.
Lee, J. D. and See, K. A. (2004). Trust in automation:
Designing for appropriate reliance. Human Factors,
page 31.
Lew, G. and Schumacher, R. M. (2020). AI and UX: Why
Artificial Intelligence Needs User Experience. Apress.
Longoni, C., Bonezzi, A., and Morewedge, C. K. (2019).
Resistance to medical artificial intelligence. Journal
of Consumer Research, 46(4):629–650.
Norman, D. A. (2005). Emotional Design: Why We Love
(or Hate) Everyday Things. Basic Books, 3rd edition.
Panesar, A. (2019). Machine Learning and AI for Health-
care: Big Data for Improved Health Outcomes.
Apress, 1st edition.
Schrepp, M. and Thomaschewski, J. (2019). Design
and validation of a framework for the creation of
user experience questionnaires. International Journal
of Interactive Multimedia and Artificial Intelligence,
5(7):88.
Sharan, N. N. and Romano, D. M. (2020). The effects of
personality and locus of control on trust in humans
versus artificial intelligence. Heliyon, 6(8):e04572.
Smyth, J. D. (2017). The SAGE Handbook of Survey
Methodology, pages 218–235. SAGE Publications
Ltd.
Sundar, S. S. (2020). Rise of Machine Agency: A Frame-
work for Studying the Psychology of Human–AI In-
teraction (HAII). Journal of Computer-Mediated
Communication, 25(1):74–88.
Zeitoun, J.-D. and Ravaud, P. (2019). L’intelligence arti-
ficielle et le m
´
etier de m
´
edecin. Les Tribunes de la
sant
´
e, 60(2):31–35.
User Reception of Babylon Health’s Chatbot
141