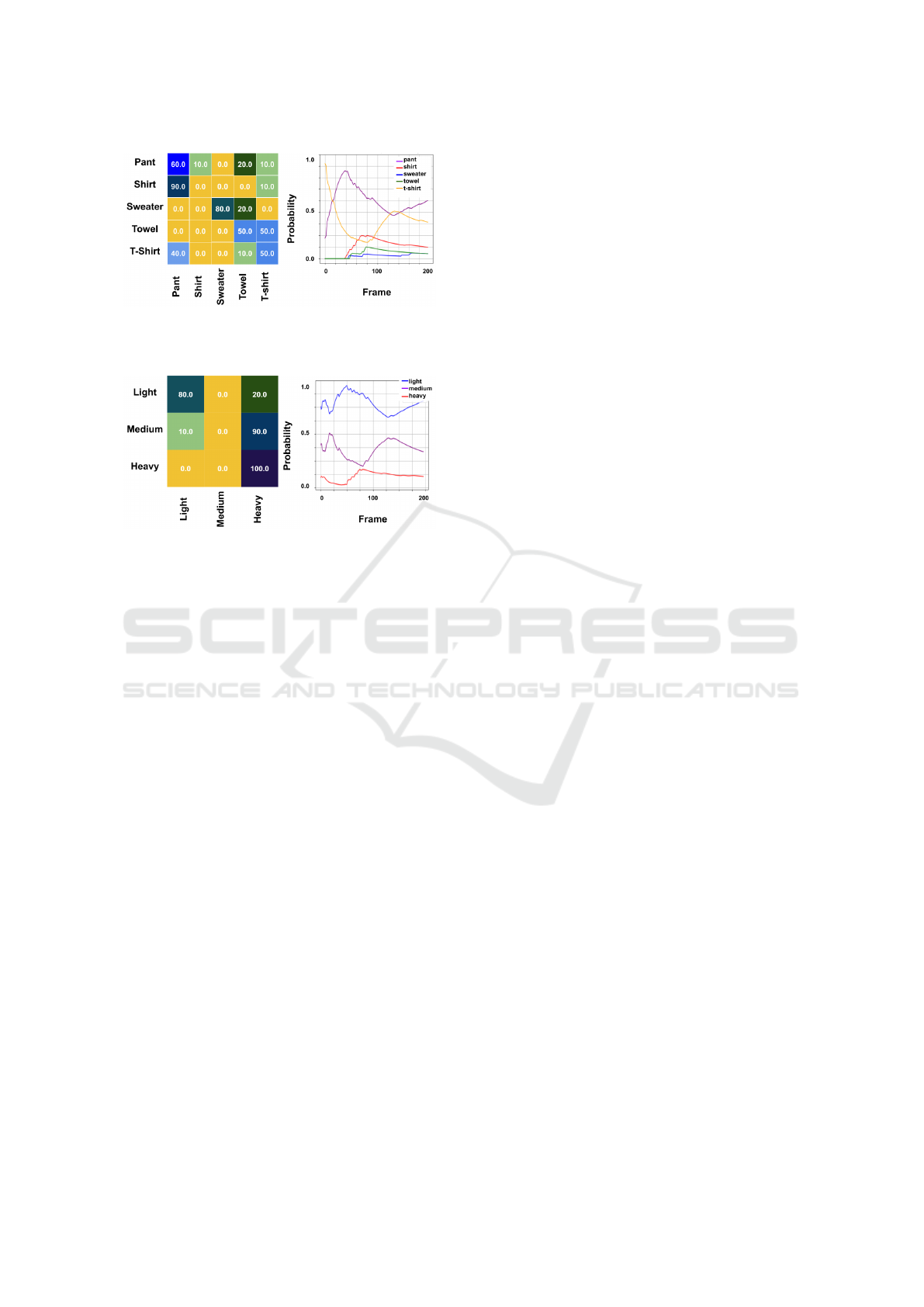
Figure 2: Continuous shape prediction (Left: Moving Aver-
age Confusion Matrix; Right: Moving Average over a video
sequence).
Figure 3: Continuous weight prediction (Left: Moving Av-
erage Confusion Matrix; Right: Moving Average over a
video sequence).
5 CONCLUSIONS
From the ablation studies we have conducted, depth
images have a better performance over RGB images
because depth captures the garment topology prop-
erties of garments. That is, our network was able
to learn dynamic changes of the garments and make
predictions on unseen garments since depth images
have a prediction accuracy of 48% and 60% while
classifying shapes and weights, accordingly. We also
show that continuous perception improves classifica-
tion accuracy. That is, weight classification, which is
an indicator of garment physical properties, observes
an increase in accuracy from 48.3% to 60% under a
continous perception paradigm. This means that our
network can learn physical properties from continu-
ous perception. However, we observed an increase
of around 1% (from 47.6% to 48%) while continu-
ously classifying garment’s shape. The marginal im-
provement while continuously classifying shape in-
dicates that further manipulations, such as flattening
(Sun et al., 2015) and unfolding (Doumanoglou et al.,
2016) are required to bring a unknown garment to a
state that can be recognised by a robot. That is, the
ability to predict dynamic information of a piece of
an unknown garment (or other deformable objects) fa-
cilitates robots’ efficiency to manipulate it by ensur-
ing how the garment will deform (Jim
´
enez and Tor-
ras, 2020; Ganapathi et al., 2020). Therefore, an un-
derstanding of the dynamics of garments and other
deformable objects can allow robots to accomplish
grasping and manipulation tasks with higher dexter-
ity
From the results, we can also observe that there
exist incorrect classifications of unseen shirts be-
cause of their similarity in their materials. There-
fore, we propose to experiment on how to improve
prediction accuracy on garments with similar mate-
rials and structures by allowing a robot to interact
with garments as proposed in (Sun et al., 2016). We
also envisage that it can be possible to learn the dy-
namic physical properties (stiffness) of real garments
from training a ’physical-similarity network’ (Phys-
Net) (Runia et al., 2020) on simulated garment mod-
els.
REFERENCES
Bhat, K. S., Twigg, C. D., Hodgins, J. K., Khosla, P. K.,
Popovi
´
c, Z., and Seitz, S. M. (2003). Estimating cloth
simulation parameters from video. In Proceedings of
the 2003 ACM SIGGRAPH/Eurographics symposium
on Computer animation, pages 37–51. Eurographics
Association.
Bohg, J., Hausman, K., Sankaran, B., Brock, O., Kragic,
D., Schaal, S., and Sukhatme, G. S. (2017). Interac-
tive perception: Leveraging action in perception and
perception in action. IEEE Transactions on Robotics,
33(6):1273–1291.
Davis, A., Bouman, K. L., Chen, J. G., Rubinstein, M., Du-
rand, F., and Freeman, W. T. (2015). Visual vibrome-
try: Estimating material properties from small motion
in video. In Proceedings of the ieee conference on
computer vision and pattern recognition, pages 5335–
5343.
Doumanoglou, A., Stria, J., Peleka, G., Mariolis, I., Petrik,
V., Kargakos, A., Wagner, L., Hlav
´
a
ˇ
c, V., Kim, T.-
K., and Malassiotis, S. (2016). Folding clothes au-
tonomously: A complete pipeline. IEEE Transactions
on Robotics, 32(6):1461–1478.
Ganapathi, A., Sundaresan, P., Thananjeyan, B., Balakr-
ishna, A., Seita, D., Grannen, J., Hwang, M., Hoque,
R., Gonzalez, J. E., Jamali, N., Yamane, K., Iba, S.,
and Goldberg, K. (2020). Learning to smooth and fold
real fabric using dense object descriptors trained on
synthetic color images.
He, K., Zhang, X., Ren, S., and Sun, J. (2016). Deep resid-
ual learning for image recognition. In Proceedings of
the IEEE conference on computer vision and pattern
recognition, pages 770–778.
Jim
´
enez, P. and Torras, C. (2020). Perception of cloth in
assistive robotic manipulation tasks. Natural Comput-
ing, pages 1–23.
Krizhevsky, A., Sutskever, I., and Hinton, G. E. (2012). Im-
agenet classification with deep convolutional neural
VISAPP 2022 - 17th International Conference on Computer Vision Theory and Applications
354