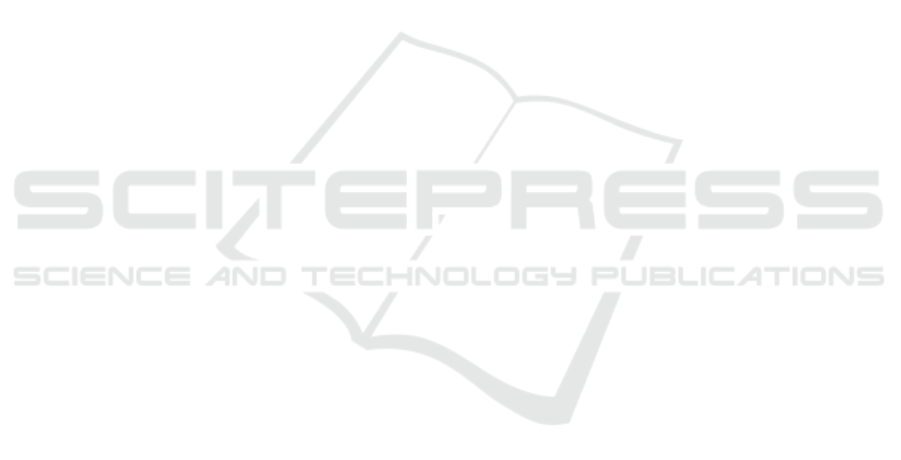
REFERENCES
Chapelle, O. and Zien, A. (2005). Semi-supervised classifi-
cation by low density separation. In AISTATS 2005.
Chen, T., Kornblith, S., Norouzi, M., and Hinton, G.
(2020a). A simple framework for contrastive learning
of visual representations. In ICML 2020.
Chen, X., Fan, H., Girshick, R., and He, K. (2020b). Im-
proved baselines with momentum contrastive learn-
ing. arXiv preprint arXiv:2003.04297.
Chintala, S. et al. (2017). Pytorch.
Codella, N. C., Gutman, D., Celebi, M. E., Helba, B.,
Marchetti, M. A., Dusza, S. W., Kalloo, A., Liopy-
ris, K., Mishra, N., Kittler, H., et al. (2018). Skin
lesion analysis toward melanoma detection: A chal-
lenge at the 2017 international symposium on biomed-
ical imaging (isbi), hosted by the international skin
imaging collaboration (isic). In ISBI 2018.
Cubuk, E. D., Zoph, B., Shlens, J., and Le, Q. (2020).
Randaugment: Practical automated data augmentation
with a reduced search space. In NeurIPS 2020.
Everingham, M., Van Gool, L., Williams, C. K. I.,
Winn, J., and Zisserman, A. (2012). The
PASCAL Visual Object Classes Challenge
2012 (VOC2012) Results. http://www.pascal-
network.org/challenges/VOC/voc2012/workshop/index.html.
Feng, Z., Zhou, Q., Gu, Q., Tan, X., Cheng, G., Lu, X., Shi,
J., and Ma, L. (2021). Dmt: Dynamic mutual training
for semi-supervised learning. CoRR, abs/2004.08514.
French, G., Laine, S., Aila, T., Mackiewicz, M., and Fin-
layson, G. (2020). Semi-supervised semantic segmen-
tation needs strong, varied perturbations. In BMVC
2020.
Hariharan, B., Arbel
´
aez, P., Bourdev, L., Maji, S., and Ma-
lik, J. (2011). Semantic contours from inverse detec-
tors. In ICCV 2011.
He, K., Fan, H., Wu, Y., Xie, S., and Girshick, R. (2020).
Momentum contrast for unsupervised visual represen-
tation learning. In CVPR 2020.
He, K., Zhang, X., Ren, S., and Sun, J. (2016). Deep resid-
ual learning for image recognition. In CVPR 2016.
Henaff, O. (2020). Data-efficient image recognition with
contrastive predictive coding. In ICML 2020.
Hung, W.-C., Tsai, Y.-H., Liou, Y.-T., Lin, Y.-Y., and
Yang, M.-H. (2018). Adversarial learning for
semi-supervised semantic segmentation. CoRR,
abs/1802.07934.
Ji, X., Henriques, J. F., and Vedaldi, A. (2019). Invariant
information clustering for unsupervised image classi-
fication and segmentation. In ICCV 2019.
Krizhevsky, A., Sutskever, I., and Hinton, G. E. (2012). Im-
ageNet Classification with Deep Convolutional Neu-
ral Networks. In NIPS 2012.
Laine, S. and Aila, T. (2017). Temporal ensembling for
semi-supervised learning. In ICLR 2017.
Li, X., Yu, L., Chen, H., Fu, C.-W., and Heng, P.-A.
(2018). Semi-supervised skin lesion segmentation via
transformation consistent self-ensembling model. In
BMVC 2018.
Liu, S., Zhi, S., Johns, E., and Davison, A. J. (2021). Boot-
strapping semantic segmentation with regional con-
trast. arXiv preprint arXiv:2104.04465.
Luo, Y., Zhu, J., Li, M., Ren, Y., and Zhang, B.
(2018). Smooth neighbors on teacher graphs for semi-
supervised learning. In CVPR 2018.
Maninis, K.-K., Caelles, S., Pont-Tuset, J., and Van Gool,
L. (2018). Deep extreme cut: From extreme points to
object segmentation. In CVPR 2018.
Mittal, S., Tatarchenko, M., and Brox, T. (2019). Semi-
supervised semantic segmentation with high-and low-
level consistency. PAMI 2019.
Miyato, T., Maeda, S.-i., Koyama, M., and Ishii, S. (2017).
Virtual adversarial training: a regularization method
for supervised and semi-supervised learning. arXiv
preprint arXiv:1704.03976.
Oliver, A., Odena, A., Raffel, C., Cubuk, E. D., and Good-
fellow, I. J. (2018). Realistic evaluation of semi-
supervised learning algorithms. In ICLR 2018.
Olsson, V., Tranheden, W., Pinto, J., and Svensson, L.
(2021). Classmix: Segmentation-based data augmen-
tation for semi-supervised learning. In WCACV 2021.
Perone, C. S. and Cohen-Adad, J. (2018). Deep semi-
supervised segmentation with weight-averaged con-
sistency targets. In Deep Learning in Medical Image
Analysis and Multimodal Learning for Clinical Deci-
sion Support.
Sajjadi, M., Javanmardi, M., and Tasdizen, T. (2016). Mu-
tual exclusivity loss for semi-supervised deep learn-
ing. In ICIP 2016.
Shu, R., Bui, H., Narui, H., and Ermon, S. (2018). A DIRT-t
approach to unsupervised domain adaptation. In ICLR
2018.
Sohn, K., Berthelot, D., Carlini, N., Zhang, Z., Zhang, H.,
Raffel, C. A., Cubuk, E. D., Kurakin, A., and Li, C.-
L. (2020). Fixmatch: Simplifying semi-supervised
learning with consistency and confidence. In NeurIPS
2020.
Tarvainen, A. and Valpola, H. (2017). Mean teachers are
better role models: Weight-averaged consistency tar-
gets improve semi-supervised deep learning results. In
NIPS 2017.
Verma, V., Lamb, A., Kannala, J., Bengio, Y., and Lopez-
Paz, D. (2019). Interpolation consistency training for
semi-supervised learning. CoRR, abs/1903.03825.
Xie, Q., Dai, Z., Hovy, E., Luong, M.-T., and Le, Q. V.
(2019). Unsupervised data augmentation. arXiv
preprint arXiv:1904.12848.
Yun, S., Han, D., Oh, S. J., Chun, S., Choe, J., and Yoo,
Y. (2019). Cutmix: Regularization strategy to train
strong classifiers with localizable features. In ICCV
2019.
Colour Augmentation for Improved Semi-supervised Semantic Segmentation
363