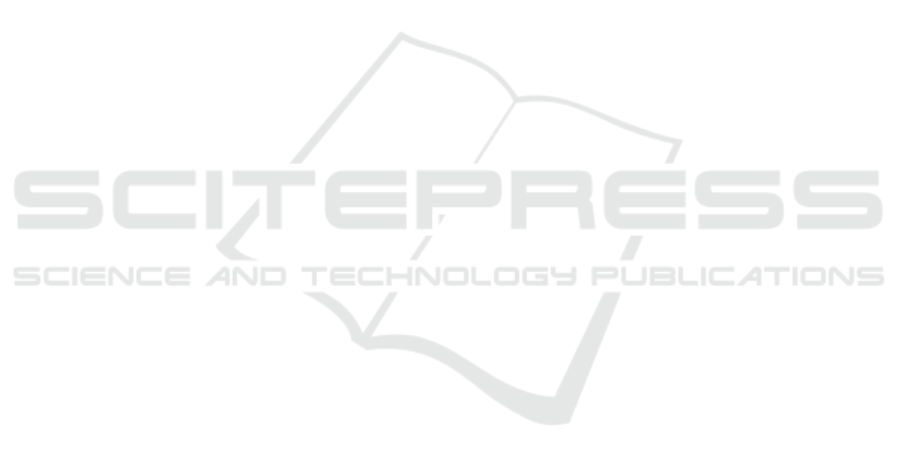
our approach should be tested in other contexts and
neural networks.
ACKNOWLEDGEMENTS
The authors gratefully acknowledge the Ministry of
Agriculture and Food which funds PNAPI through
CASDAR (the special appropriation account “Agri-
culture and Rural Development”) under project num-
ber 18 ART 1831 as well as the support and help of
Alexandre Dangl
´
eant, ITSAP (Technical and Scien-
tific Institute of Beekeeping and Pollination).
REFERENCES
Dai, J., Li, Y., He, K., and Sun, J. (2016). R-fcn: Object de-
tection via region-based fully convolutional networks.
In International Conference on Neural Information
Processing Systems. Curran Associates Inc.
Dembski J., S. J. (2020). Weighted clustering for bees de-
tection on video images. In Computational Science.
Springer.
Duan, K., Xie, L., Qi, H., Bai, S., Huang, Q., and Tia, Q.
(2020). Corner proposal network for anchor-free, two-
stage object detection. In European Conference on
Computer Vision – ECCV.
G
¨
ahlert, N., Hanselmann, N., Franke, U., and Denzler, J.
(2020). Visibility guided nms: Efficient boosting of
amodal object detection in crowded traffic scenes.
Girshick, R. (2015). Fast r-cnn. In 2015 IEEE International
Conference on Computer Vision (ICCV).
Girshick, R., Donahue, J., Darrell, T., and Malik, J. (2014).
Rich feature hierarchies for accurate object detection
and semantic segmentation.
Goldman, E., Herzig, R., Eisenschtat, A., Goldberger, J.,
and Hassner, T. (2019). Precise detection in densely
packed scenes. In 2019 IEEE/CVF Conference on
Computer Vision and Pattern Recognition (CVPR).
Kulyukin, V. and Mukherjee, S. (2018). On video analysis
of omnidirectional bee traffic: Counting bee motions
with motion detection and image classification. Ap-
plied Sciences.
Kulyukin, V. A. and Reka, S. K. (2016). Toward sustainable
electronic beehive monitoring: Algorithms for omni-
directional bee counting from images and harmonic
analysis of buzzing signals.
Law, H. and Deng, J. (2018). Cornernet: Detecting objects
as paired keypoints. In European Conference on Com-
puter Vision (ECCV).
Lin, T.-Y., Doll
´
ar, P., Girshick, R., He, K., Hariharan, B.,
and Belongie, S. (2017a). Feature pyramid networks
for object detection. In IEEE Conference on Computer
Vision and Pattern Recognition (CVPR).
Lin, T.-Y., Goyal, P., Girshick, R., He, K., and Doll
´
ar, P.
(2017b). Focal loss for dense object detection. In
IEEE International Conference on Computer Vision
(ICCV).
Liu, W., Anguelov, D., Erhan, D., Szegedy, C., Reed, S., Fu,
C.-Y., and Berg, A. C. (2016). Ssd: Single shot multi-
box detector. Lecture Notes in Computer Science.
Liu, Y., Liu, L., Rezatofighi, H., Do, T.-T., Shi, Q., and
Reid, I. (2019). Learning pairwise relationship for
multi-object detection in crowded scenes.
Magnier, B., Ekszterowicz, G., Laurent, J., Rival, M., and
Pfister, F. (2018). Bee hive traffic monitoring by track-
ing bee flight paths. In Proceedings of the 13th Inter-
national Joint Conference on Computer Vision, Imag-
ing and Computer Graphics Theory and Applications.
SCITEPRESS - Science and Technology Publications.
Magnier, B., Gabbay, E., Bougamale, F., Moradi, B., Pfis-
ter, F., and Slangen, P. R. (2019). Multiple honey bees
tracking and trajectory modeling. In Multimodal Sens-
ing: Technologies and Applications. SPIE.
Qiu, H., Ma, Y., Li, Z., Liu, S., and Sun, J. (2020). Bor-
derdet: Border feature for dense object detection. In
European Conference on Computer Vision – ECCV.
Redmon, J., Divvala, S., Girshick, R., and Farhadi, A.
(2016). You only look once: Unified, real-time object
detection. In IEEE Conference on Computer Vision
and Pattern Recognition (CVPR).
Ren, S., He, K., Girshick, R., and Sun, J. (2015). Faster
r-cnn: Towards real-time object detection with region
proposal networks. In Advances in Neural Informa-
tion Processing Systems. Curran Associates, Inc.
Sipos, T., Donk
´
o, T., J
´
ocs
´
ak, I., and Keszthelyi, S.
(2021). Study of morphological features in pre-
imaginal honey bee impaired by varroa destructor by
means of computer tomography. Insects.
Tian, Z., Shen, C., Chen, H., and He, T. (2019). Fcos:
Fully convolutional one-stage object detection. In
IEEE/CVF International Conference on Computer Vi-
sion (ICCV).
Tiwari, A. (2018). A deep learning approach to recognizing
bees in video analysis of bee traffic. PhD thesis, Utah
state university.
Tu, G. J., Hansen, M. K., Kryger, P., and Ahrendt, P. (2016).
Automatic behaviour analysis system for honeybees
using computer vision. Computers and Electronics in
Agriculture.
Wei, F., Sun, X., Li, H., Wang, J., and Lin, S. (2020). Point-
set anchors for object detection, instance segmenta-
tion and pose estimation. In European Conference on
Computer Vision – ECCV.
Xi, Y., Zheng, J., He, X., Jia, W., Li, H., Xie, Y., Feng, M.,
and Li, X. (2020). Beyond context: Exploring seman-
tic similarity for small object detection in crowded
scenes. Pattern Recognition Letters.
Zhang, K., Xiong, F., Sun, P., Hu, L., Li, B., and Yu, G.
(2019). Double anchor r-cnn for human detection in a
crowd.
Zhang, S., Wen, L., Bian, X., Lei, Z., and Li, S. Z. (2018).
Occlusion-aware r-cnn: Detecting pedestrians in a
crowd. In Computer Vision – ECCV. Springer Inter-
national Publishing.
Zhou, X., Zhuo, J., and Kr
¨
ahenb
¨
uhl, P. (2019). Bottom-
up object detection by grouping extreme and center
points. In IEEE/CVF Conference on Computer Vision
and Pattern Recognition (CVPR).
VISAPP 2022 - 17th International Conference on Computer Vision Theory and Applications
350