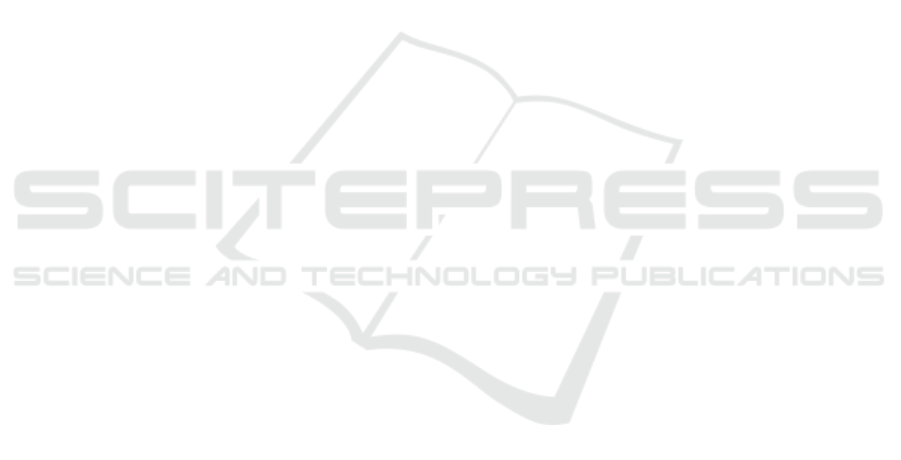
accuracy, in contrast to upsampling to match the
size of HR images. We also compared the accuracy
when using high-frequency and low-frequency com-
ponents extracted from the upsampled LR images in
simulation experiments. We confirmed that the low-
frequency components, which correspond to down-
sampled images, are likely to contain many informa-
tive features.
In future work, we intend to develop a re-
sampling method for further increasing person re-
identification accuracy and expand the evaluation
on various datasets containing person appearances
changes. We also need to analyze the relevance of
our findings to the image generation process, such as
optical systems in real environments.
REFERENCES
Aghdam, O. A., Bozorgtabar, B., Ekenel, H. K., and Thi-
ran, J. (2019). Exploring factors for improving low
resolution face recognition. In Proceedings of the
IEEE/CVF Conference on Computer Vision and Pat-
tern Recognition Workshops, pages 2363–2370.
Dong, C., Loy, C. C., He, K., and Tang, X. (2015). Image
super-resolution using deep convolutional networks.
IEEE Transactions on Pattern Analysis and Machine
Intelligence, 38(2):295–307.
He, K., Zhang, X., Ren, S., and Sun, J. (2016). Deep resid-
ual learning for image recognition. In Proceedings of
the IEEE Conference on Computer Vision and Pattern
Recognition, pages 770–778.
Hennings-Yeomans, P. H., Baker, S., and Kumar, B. V. K. V.
(2008). Simultaneous super-resolution and feature ex-
traction for recognition of low-resolution faces. In
2008 IEEE Conference on Computer Vision and Pat-
tern Recognition, pages 1–8.
Hermans, A., Beyer, L., and Leibe, B. (2017). In defense
of the triplet loss for person re-identification. CoRR,
abs/1703.07737.
Jiao, J., Zheng, W. S., Wu, A., Zhu, X., and Gong, S. (2018).
Deep low-resolution person re-identification. In Pro-
ceedings of the Thirty-second Association for the Ad-
vancement of Artificial Intelligence Conference on Ar-
tificial Intelligence.
Jing, X. Y., Zhu, X., Wu, F., You, X., Liu, Q., Yue, D.,
Hu, R., and Xu, B. (2015). Super-resolution person
re-identification with semi-coupled low-rank discrim-
inant dictionary learning. In Proceedings of the IEEE
Conference on Computer Vision and Pattern Recogni-
tion, pages 695–704.
Li, W., Zhao, R., and Wang, X. (2012). Human re-
identification with transferred metric learning. In Pro-
ceedings of the Asian Conference on Computer Vision,
pages 31–44.
Nishiyama, M., Nakano, S., Yotsumoto, T., Yoshimura,
H., Iwai, Y., and Sugahara, K. (2016). Person re-
identification using co-occurrence attributes of physi-
cal and adhered human characteristics. In Proceedings
of the International Conference on Pattern Recogni-
tion, pages 2085–2090.
Shi, Y. and Jain, A. K. (2019). Probabilistic face embed-
dings. In Proceedings of the IEEE International Con-
ference on Computer Vision, pages 6902–6911.
Szegedy, C., Vanhoucke, V., Ioffe, S., Shlens, J., and Wo-
jna, Z. (2016). Rethinking the inception architecture
for computer vision. In Proceedings of the IEEE Con-
ference on Computer Vision and Pattern Recognition,
pages 2818–2826.
Szegedy, C., Vanhoucke, V., Ioffe, S., Shlens, J., and Wo-
jna, Z. (2016). Rethinking the inception architecture
for computer vision. In Proceedings of the IEEE Con-
ference on Computer Vision and Pattern Recognition,
pages 2818–2826.
Wang, X., Yu, K., Wu, S., Gu, J., Liu, Y., Dong, C., Qiao,
Y., and Loy, C. C. (2018). Esrgan: Enhanced super-
resolution generative adversarial networks. In Pro-
ceedings of the European Conference on Computer
Vision Workshops, pages 63–79.
Wang, Z., Hu, R., Yu, Y., Jiang, J., Liang, C., and Wang,
J. (2016). Scale-adaptive low-resolution person re-
identification via learning a discriminating surface. In
Proceedings of the International Joint Conferences on
Artificial Intelligence, volume 2, page 6.
Wang, Z., Miao, Z., Wu, Q. M. J., Wan, Y., and Tang, Z.
(2014). Low-resolution face recognition: a review.
The Visual Computer, 30(4):359–386.
Weinberger, K. Q. and Saul, L. K. (2009). Distance met-
ric learning for large margin nearest neighbor clas-
sification. Journal of Machine Learning Research,
10(Feb):207–244.
Zhang, K., Gool, L. V., and Timofte, R. (2020). Deep un-
folding network for image super-resolution. In Pro-
ceedings of the IEEE Conference on Computer Vision
and Pattern Recognition, pages 3217–3226.
Zheng, L., Shen, L., Tian, L., Wang, S., Wang, J., and Tian,
Q. (2015). Scalable person re-identification: A bench-
mark. In Proceedings of the IEEE International Con-
ference on Computer Vision, pages 1116–1124.
Zheng, W., Mang, Y., Fan, Y., Xiang, B., and Shin’ichi,
S. (2018). Cascaded sr-gan for scale-adaptive low
resolution person re-identification. In Proceedings of
the International Joint Conference on Artificial Intel-
ligence, pages 3891–3897.
Zhong, Z., Zheng, L., Cao, D., and Li, S. (2017). Re-
ranking person re-identification with k-reciprocal en-
coding. In Proceedings of the IEEE Conference
on Computer Vision and Pattern Recognition, pages
3652–3661.
Zhou, K. and Xiang, T. (2019). Torchreid: A library
for deep learning person re-identification in pytorch.
CoRR, abs/1910.10093.
Zhou, K., Yang, Y., Cavallaro, A., and Xiang, T.
(2019). Omni-scale feature learning for person re-
identification. In Proceedings of the IEEE/CVF In-
ternational Conference on Computer Vision, pages
3701–3711.
Zou, W. W. W. and Yuen, P. C. (2011). Very low resolu-
tion face recognition problem. IEEE Transactions on
Image Processing, 21(1):327–340.
VISAPP 2022 - 17th International Conference on Computer Vision Theory and Applications
358