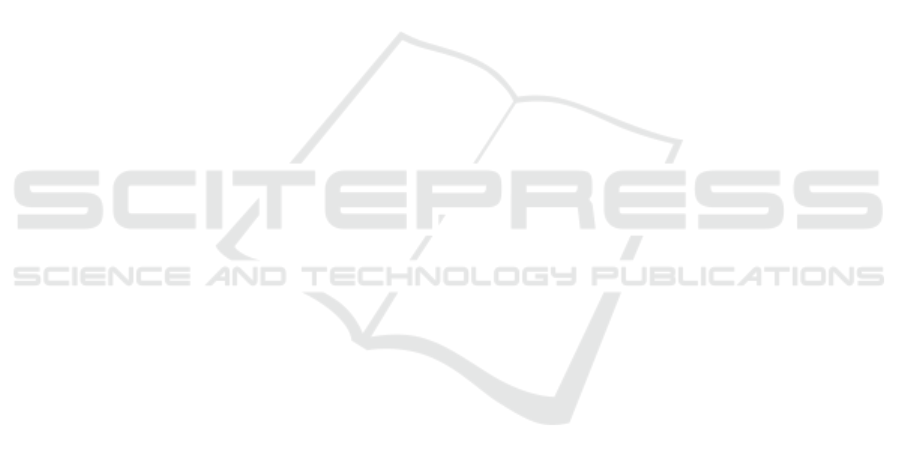
Rav
`
ı, D., Wong, C., Deligianni, F., Berthelot, M., Andreu-
Perez, J., Lo, B., and Yang, G.-Z. (2017). Deep learn-
ing for health informatics. IEEE journal of biomedical
and health informatics, 21(1):4–21.
Sadeque, F., Xu, D., and Bethard, S. (2017). Uarizona at the
clef erisk 2017 pilot task: linear and recurrent models
for early depression detection. In CEUR workshop
proceedings, volume 1866. NIH Public Access.
Schmidhuber, J. (2015). Deep learning in neural networks:
An overview. CoRR, abs/1404.7828.
Sengupta, P. and Benjamin, A. (2015). Prevalence of de-
pression and associated risk factors among the elderly
in urban and rural field practice areas of a tertiary
care institution in Ludhiana. Indian Journal of Public
Health, 59(1):3.
Silva, M. T., Galvao, T. F., Martins, S. S., and Pereira,
M. G. (2014). Prevalence of depression morbid-
ity among brazilian adults: a systematic review and
meta-analysis. Revista Brasileira de Psiquiatria,
36(3):262–270.
Stapley, E., Midgley, N., and Target, M. (2016). The ex-
perience of being the parent of an adolescent with a
diagnosis of depression. Journal of Child and Family
Studies, 25(2):618–630.
Stewart, J. C., Rand, K. L., Muldoon, M. F., and Kamarck,
T. W. (2009). A prospective evaluation of the direc-
tionality of the depression–inflammation relationship.
Brain, behavior, and immunity, 23(7):936–944.
Sunmoo, Y., Basirah, T., et al. (2014). Using a data mining
approach to discover behavior correlates of chronic
disease: a case study of depression. Studies in health
technology and informatics, 201:71.
Suzuki, M., Furihata, R., Konno, C., Kaneita, Y., Ohida, T.,
and Uchiyama, M. (2018). Stressful events and coping
strategies associated with symptoms of depression: A
japanese general population survey. Journal of affec-
tive disorders, 238:482–488.
Tadesse, M. M., Lin, H., Xu, B., and Yang, L. (2019). De-
tection of depression-related posts in reddit social me-
dia forum. IEEE Access, 7:44883–44893.
Trotzek, M., Koitka, S., and Friedrich, C. M. (2017). Lin-
guistic metadata augmented classifiers at the clef 2017
task for early detection of depression. In CLEF (Work-
ing Notes).
Tung, C. and Lu, W. (2016). Analyzing depression ten-
dency of web posts using an event-driven depression
tendency warning model. Artificial Intelligence in
Medicine, 66:53–62.
Villegas, M. P., Funez, D. G., Ucelay, M. J. G., Cagnina,
L. C., and Errecalde, M. L. (2017). Lidic-unsl’s par-
ticipation at erisk 2017: Pilot task on early detection
of depression. In CLEF (Working Notes).
Wan, Z., Huang, J., Zhang, H., Zhou, H., Yang, J., and
Zhong, N. (2020). Hybrideegnet: A convolutional
neural network for eeg feature learning and depression
discrimination. IEEE Access, 8:30332–30342.
Wang, H., Zhou, Y., Yu, F., Zhao, L., Wang, C., and Ren, Y.
(2019). Fusional recognition for depressive tendency
with multi-modal feature. IEEE Access, 7:38702–
38713.
Wang, J., Manuel, D., Williams, J., Schmitz, N., Gilmour,
H., Patten, S., MacQueen, G., and Birney, A. (2013).
Development and validation of prediction algorithms
for major depressive episode in the general popula-
tion. Journal of Affective Disorders, 151(1):39–45.
Winokur, A., Maislin, G., Phillips, J. L., and Amsterdam,
J. D. (1988). Insulin resistance after oral glucose tol-
erance testing in patients with major depression. Am J
Psychiatry, 145(3):325–330.
Witten, I. H., Frank, E., Hall, M. A., and Pal, C. J. (2016).
Data Mining: practical machine learning tools and
techniques with Java implementations. Morgan Kauf-
mann, 4 edition.
Wray, N. R., Ripke, S., Mattheisen, M., Trzaskowski, M.,
Byrne, E. M., Abdellaoui, A., Adams, M. J., Agerbo,
E., Air, T. M., Andlauer, T. M., et al. (2018). Genome-
wide association analyses identify 44 risk variants and
refine the genetic architecture of major depression.
Nature genetics, 50(5):668.
Xing, Y., Rao, N., Miao, M., Li, Q., Li, Q., Chen, X., Zhang,
Q., and Wu, J. (2019). Task-state heart rate variabil-
ity parameter-based depression detection model and
effect of therapy on the parameters. IEEE Access,
7:105701–105709.
Yang, H. and Bath, P. A. (2019). Automatic prediction of
depression in older age. In Proceedings of the third
International Conference on Medical and Health In-
formatics 2019, pages 36–44.
Yang, L., Jiang, D., He, L., Pei, E., Oveneke, M. C., and
Sahli, H. (2016). Decision Tree Based Depression
Classification from Audio Video and Language Infor-
mation. In Proceedings of the 6th International Work-
shop on Audio/Visual Emotion Challenge - AVEC ’16,
AVEC ’16, pages 89–96, New York, NY, USA. ACM.
Yanikkerem, E., Ay, S., Mutlu, S., and Goker, A. (2013).
Antenatal depression: Prevalence and risk factors in a
hospital based Turkish sample. Journal of the Pakistan
Medical Association, 63(4):472–477.
Zeng, L. L., Shen, H., Liu, L., and Hu, D. (2014). Un-
supervised classification of major depression using
functional connectivity MRI. Human Brain Mapping,
35(4):1630–1641.
HEALTHINF 2022 - 15th International Conference on Health Informatics
420