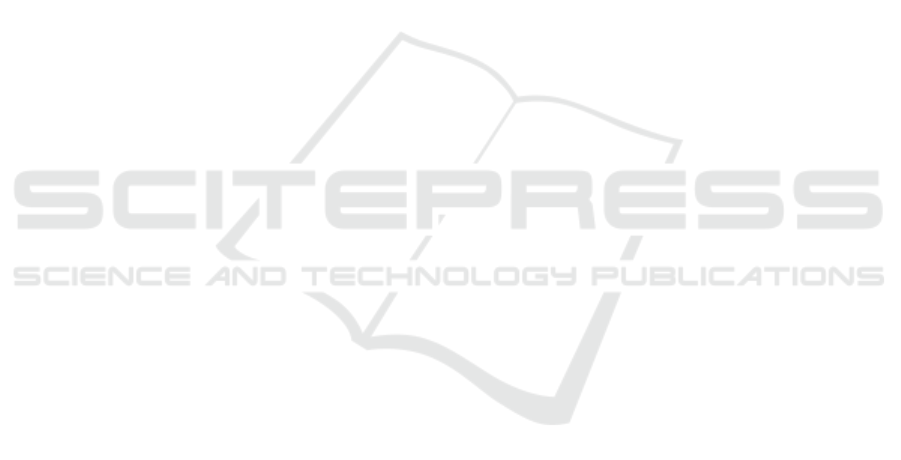
Conference on Computer Vision and Pattern Recogni-
tion (CVPR), volume 1, pages 539–546.
Deng, J., Dong, W., Socher, R., Li, L.-J., Li, K., and Fei-
Fei, L. (2009). Imagenet: A large-scale hierarchical
image database. In 2009 IEEE Conference on Com-
puter Vision and Pattern Recognition (CVPR), pages
248–255.
Deng, J., Guo, J., Xue, N., and Zafeiriou, S. (2019). Ar-
cface: Additive angular margin loss for deep face
recognition. In Proceedings of the IEEE/CVF Con-
ference on Computer Vision and Pattern Recognition
(CVPR), pages 4690–4699.
Geng, C., Huang, S.-J., and Chen, S. (2020). Recent ad-
vances in open set recognition: A survey. IEEE Trans-
actions on Pattern Analysis and Machine Intelligence
(TPAMI).
He, K., Zhang, X., Ren, S., and Sun, J. (2016). Deep resid-
ual learning for image recognition. In Proceedings of
the IEEE conference on computer vision and pattern
recognition (CVPR), pages 770–778.
Hendrycks, D. and Gimpel, K. (2017). A baseline for de-
tecting misclassified and out-of-distribution examples
in neural networks. In 5th International Conference
on Learning Representations (ICLR).
Krizhevsky, A. (2012). Learning multiple layers of features
from tiny images. University of Toronto.
LeCun, Y., Cortes, C., and Burges, C. (2010). Mnist hand-
written digit database. ATT Labs [Online]. Available:
http://yann.lecun.com/exdb/mnist, 2.
Liu, W., Wen, Y., Yu, Z., Li, M., Raj, B., and Song, L.
(2017). Sphereface: Deep hypersphere embedding for
face recognition. In Proceedings of the IEEE con-
ference on computer vision and pattern recognition
(CVPR), pages 212–220.
Mensink, T., Verbeek, J., Perronnin, F., and Csurka, G.
(2013). Distance-based image classification: Gener-
alizing to new classes at near-zero cost. IEEE Trans-
actions on Pattern Analysis and Machine Intelligence
(TPAMI), 35(11):2624–2637.
Netzer, Y., Wang, T., Coates, A., Bissacco, A., Wu, B., and
Ng, A. Y. (2011). Reading digits in natural images
with unsupervised feature learning.
Perera, P., Morariu, V. I., Jain, R., Manjunatha, V.,
Wigington, C., Ordonez, V., and Patel, V. M.
(2020). Generative-discriminative feature representa-
tions for open-set recognition. In Proceedings of the
IEEE/CVF Conference on Computer Vision and Pat-
tern Recognition (CVPR).
Rippel, O., Mertens, P., and Merhof, D. (2020). Model-
ing the distribution of normal data in pre-trained deep
features for anomaly detection. In 2020 25th Inter-
national Conference on Pattern Recognition (ICPR),
pages 6726–6733.
Scheirer, W. J., de Rezende Rocha, A., Sapkota, A., and
Boult, T. E. (2013). Toward open set recognition.
IEEE Transactions on Pattern Analysis and Machine
Intelligence (TPAMI), 35(7):1757–1772.
Sun, X., Yang, Z., Zhang, C., Ling, K.-V., and Peng, G.
(2020). Conditional gaussian distribution learning for
open set recognition. In Proceedings of the IEEE/CVF
Conference on Computer Vision and Pattern Recogni-
tion (CVPR), pages 13480–13489.
Tan, M. and Le, Q. (2019). Efficientnet: Rethinking model
scaling for convolutional neural networks. In Inter-
national Conference on Machine Learning (CIML),
pages 6105–6114.
Wang, H., Wang, Y., Zhou, Z., Ji, X., Gong, D., Zhou, J.,
Li, Z., and Liu, W. (2018). Cosface: Large margin co-
sine loss for deep face recognition. In Proceedings of
the IEEE conference on computer vision and pattern
recognition (CVPR), pages 5265–5274.
Wang, J., song, Y., Leung, T., Rosenberg, C., Wang, J.,
Philbin, J., Chen, B., and Wu, Y. (2014). Learning
fine-grained image similarity with deep ranking. Pro-
ceedings of the IEEE Computer Society Conference on
Computer Vision and Pattern Recognition (CVPR).
Wang, M. and Deng, W. (2021). Deep face recognition: A
survey. Neurocomputing, 429:215–244.
Wen, Y., Zhang, K., Li, Z., and Qiao, Y. (2016). A discrim-
inative feature learning approach for deep face recog-
nition. In Leibe, B., Matas, J., Sebe, N., and Welling,
M., editors, European conference on computer vision
(ECCV), pages 499–515. Springer International Pub-
lishing.
Yoshihashi, R., Shao, W., Kawakami, R., You, S., Iida, M.,
and Naemura, T. (2019). Classification-reconstruction
learning for open-set recognition. In Proceedings of
the IEEE/CVF Conference on Computer Vision and
Pattern Recognition (CVPR), pages 4016–4025.
Applying Center Loss to Multidimensional Feature Space in Deep Neural Networks for Open-set Recognition
365