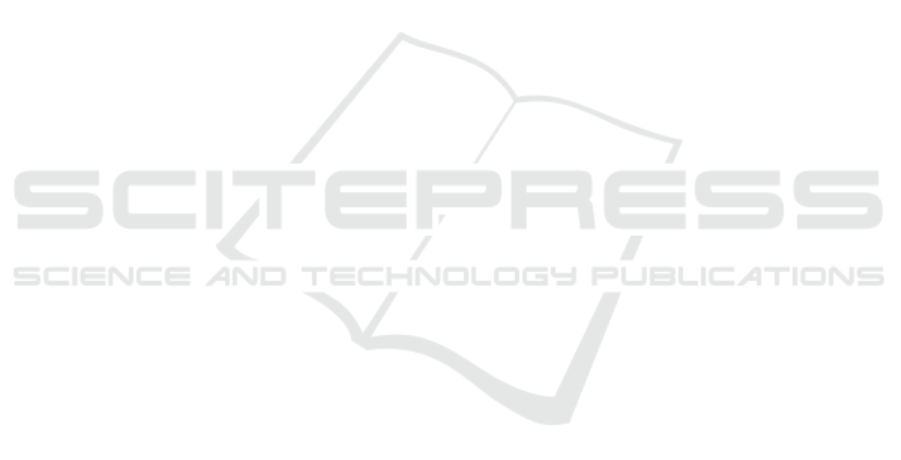
might be because the V-PCC codec utilizes at least 6
projection planes which are redundant for single-view
point clouds. The normal vector segmentation in the
V-PCC algorithm should have been able to take care
of this issue, however, the results suggest otherwise.
G-PCC achieves higher BD PSNR gains for geometry
which suggest that G-PCC is better suited for com-
pressing single-view point clouds.
As seen in Figure 3, MPEG’s G-PCC outperforms
both Draco and V-PCC on both MIA-Heritage-Low
and Washington in terms of both geometry and colour
reconstruction quality. For the Washington dataset
Draco performs also most identical to the G-PCC and
has a higher BD PSNR gain than V-PCC for geometry
reconstruction quality. Both the MIA-Heritage-Low
and the Washington datasets consist of sparse point
clouds where the distances between occupied voxels
are large, see Figure 1. For G-PCC the octree data
structure was chosen since it was made to compress
sparse point clouds well which seems to be confirmed
by the results obtained in this paper.
It is worth noting that while G-PCC outperforms
Draco on the MIA-Heritage-Low and the Washington
datasets, it does so by a margin that is smaller than
those obtained on 8iVFB-v2 and MIA-Heritage. This
might suggest that Draco is also suitable for compres-
sion of sparse point clouds. Both Draco and G-PCC
outperform V-PCC heavily when compressing geo-
metric information in sparse point clouds. Further-
more, Figure 3 shows that G-PCC performs well on a
wide variety of datasets and does so consistently, e.g.
G-PCC performs almost similar on dense object and
dense scene datasets.
5 CONCLUSION
This paper proposes a set of binary properties to de-
scribe point clouds and argues that point cloud com-
pression methods should be evaluated on a diverse set
of datasets with different properties. To this end, an
evaluation framework with an associated open source
evaluation pipeline has been proposed with publicly
available datasets.
Furthermore we also propose the MIA-Heritage
dataset as a static dense point cloud compression
dataset benchmark, as well as a metric for surface
density to evaluate whether a point cloud is sparse or
dense.
The evaluation of 3D compression methods finds
that V-PCC provides good reconstruction quality on
dense static and dense dynamic point clouds. It per-
forms the strongest on objects but also outperforms
Draco and G-PCC on dense scenes in terms of recon-
struction quality. V-PCC is outperformed by G-PCC
and Draco on sparse datasets, with Draco and G-PCC
performing somewhat equally. Furthermore, the re-
sults suggest that V-PCC is challenged on single-view
datasets in terms of geometric reconstruction quality.
REFERENCES
Bello, S. A., Yu, S., and Wang, C. (2020). Review: deep
learning on 3d point clouds. CoRR, abs/2001.06280.
Bjøntegaard, G. (2001). Calculation of average psnr differ-
ences between rd-curves.
Bruder, G., Steinicke, F., and N
¨
uchter, A. (2014). Poster:
Immersive point cloud virtual environments. In 2014
IEEE Symposium on 3D User Interfaces (3DUI),
pages 161–162.
Cao, C., Preda, M., and Zaharia, T. (2019). 3d point cloud
compression: A survey. In The 24th International
Conference on 3D Web Technology, pages 1–9.
Chen, L.-C., Hoang, D.-C., Lin, H.-I., and Nguyen, T.-H.
(2016). Innovative methodology for multi-view point
cloud registration in robotic 3d object scanning and
reconstruction. Applied Sciences, 6(5).
d’Eon, E., Harrison, B., Myers, T., and Chou,
P. A. (2017). 8i voxelized full bodies-a vox-
elized point cloud dataset. ISO/IEC JTC1/SC29
Joint WG11/WG1 (MPEG/JPEG) input document
WG11M40059/WG1M74006, 7:8.
Fan, Y., Huang, Y., and Peng, J. (2013). Point cloud com-
pression based on hierarchical point clustering. In
2013 Asia-Pacific Signal and Information Processing
Association Annual Summit and Conference, pages 1–
7.
Graziosi, D., Nakagami, O., Kuma, S., Zaghetto, A.,
Suzuki, T., and Tabatabai, A. (2020). An overview of
ongoing point cloud compression standardization ac-
tivities: video-based (v-pcc) and geometry-based (g-
pcc). APSIPA Transactions on Signal and Information
Processing, 9:e13.
Gu, S., Hou, J., Zeng, H., Yuan, H., and Ma, K.-K. (2019).
3d point cloud attribute compression using geometry-
guided sparse representation. IEEE Transactions on
Image Processing, 29:796–808.
Huang, Y., Peng, J., Kuo, C.-C. J., and Gopi, M. (2008).
A generic scheme for progressive point cloud cod-
ing. IEEE Transactions on Visualization and Com-
puter Graphics, 14(2):440–453.
Ingale, A. K. and J., D. U. (2021). Real-time 3d reconstruc-
tion techniques applied in dynamic scenes: A sys-
tematic literature review. Computer Science Review,
39:100338.
JPEG (2020). Final call for evidence on jpeg pleno point
cloud coding. ISO/IEC JTC 1/SC 29/WG 1 (ITU-T
SG16).
Kim, J., Im, J., Rhyu, S., and Kim, K. (2020). 3d motion
estimation and compensation method for video-based
point cloud compression. IEEE Access, 8:83538–
83547.
VISAPP 2022 - 17th International Conference on Computer Vision Theory and Applications
392