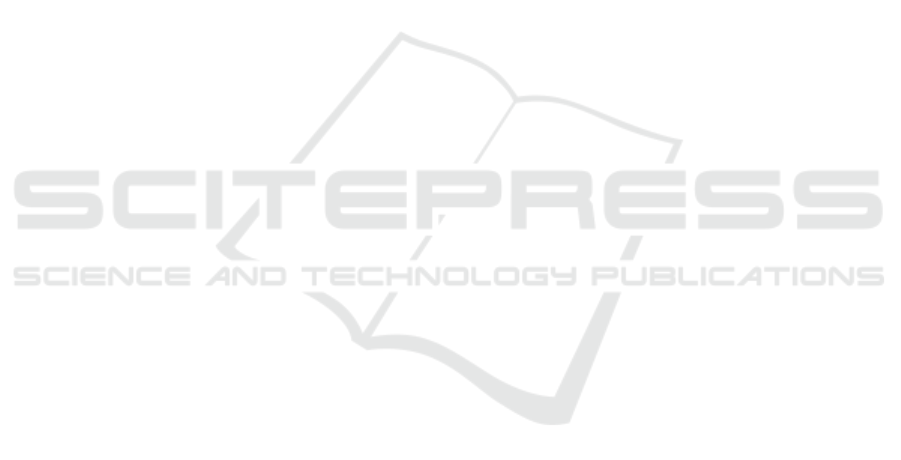
networks to classify kernel fragments into predefined
sieve sizes. These predictions allow for estimation of
CSPS with a strong correlation against physical sam-
ples. We believe SieveNet can be extended to other
domains where the PSD is also of interest, such as ag-
glomerates or medical imaging, given a definition of
appropriate sieved-based anchors.
REFERENCES
Andersson, T., Thurley, M. J., and Marklund, O. (2007).
Visibility classification of pellets in piles for sizing
without overlapped particle error. In 9th Biennial Con-
ference of the Australian Pattern Recognition Society
on Digital Image Computing Techniques and Applica-
tions (DICTA 2007), pages 508–514.
Drewry, J. L., Luck, B. D., Willett, R. M., Rocha, E. M., and
Harmon, J. D. (2019). Predicting kernel processing
score of harvested and processed corn silage via image
processing techniques. Computers and Electronics in
Agriculture, 160:144 – 152.
Duan, J., Liu, X., Wu, X., and Mao, C. (2020). Detection
and segmentation of iron ore green pellets in images
using lightweight u-net deep learning network. vol-
ume 32, pages 5775 – 5790.
Fritz, K., Koenig, D., Klauck, U., and Teutsch, M. (2019).
Generalization ability of region proposal networks
for multispectral person detection. Automatic Target
Recognition XXIX.
He, K., Zhang, X., Ren, S., and Sun, J. (2016). Deep resid-
ual learning for image recognition. In 2016 IEEE Con-
ference on Computer Vision and Pattern Recognition,
CVPR 2016, Las Vegas, NV, USA, June 27-30, 2016,
pages 770–778. IEEE Computer Society.
Heinrichs, J. and Coleen, M. J. (2016). Penn state particle
separator.
Marsh, B. H. (2013). A comparison of fuel usage and
harvest capacity in self-propelled forage harvesters.
International Journal of Agricultural and Biosystems
Engineering, 7(7):649 – 654.
Mertens, D. (2005). Particle size, fragmentation index, and
effective fiber: Tools for evaluating the physical at-
tributes of corn silages. In: Proceedings of the Four-
State Dairy Nutrition and Management Conference.
Rasmussen, C. B., Kirk, K., and Moeslund, T. B. (2021).
Anchor tuning in faster r-cnn for measuring corn
silage physical characteristics. Computers and Elec-
tronics in Agriculture, 188:106344.
Rasmussen, C. B. and Moeslund, T. B. (2019). Maize silage
kernel fragment estimation using deep learning-based
object recognition in non-separated kernel/stover rgb
images. Sensors, 19:3506.
Ren, S., He, K., Girshick, R., and Sun, J. (2015). Faster
r-cnn: Towards real-time object detection with region
proposal networks. In Proceedings of the 28th Inter-
national Conference on Neural Information Process-
ing Systems - Volume 1, NIPS’15, page 91–99, Cam-
bridge, MA, USA. MIT Press.
Sharma, K., Gold, M., Zurbruegg, C., Leal-Taixe, L., and
Wegner, J. D. (2020). Histonet: Predicting size
histograms of object instances. In Proceedings of
the IEEE/CVF Winter Conference on Applications of
Computer Vision (WACV).
Soloy, A., Turki, I., Fournier, M., Costa, S., Peuziat, B.,
and Lecoq, N. (2020). A deep learning-based method
for quantifying and mapping the grain size on pebble
beaches. Remote Sensing, 12(21).
Wu, Y., Kirillov, A., Massa, F., Lo, W.-Y., and Gir-
shick, R. (2019). Detectron2. https://github.com/
facebookresearch/detectron2.
Xu, X., Zhou, F., Liu, B., Fu, D., and Bai, X. (2019). Effi-
cient multiple organ localization in ct image using 3d
region proposal network. IEEE Transactions on Med-
ical Imaging, 38(8):1885–1898.
VISAPP 2022 - 17th International Conference on Computer Vision Theory and Applications
124