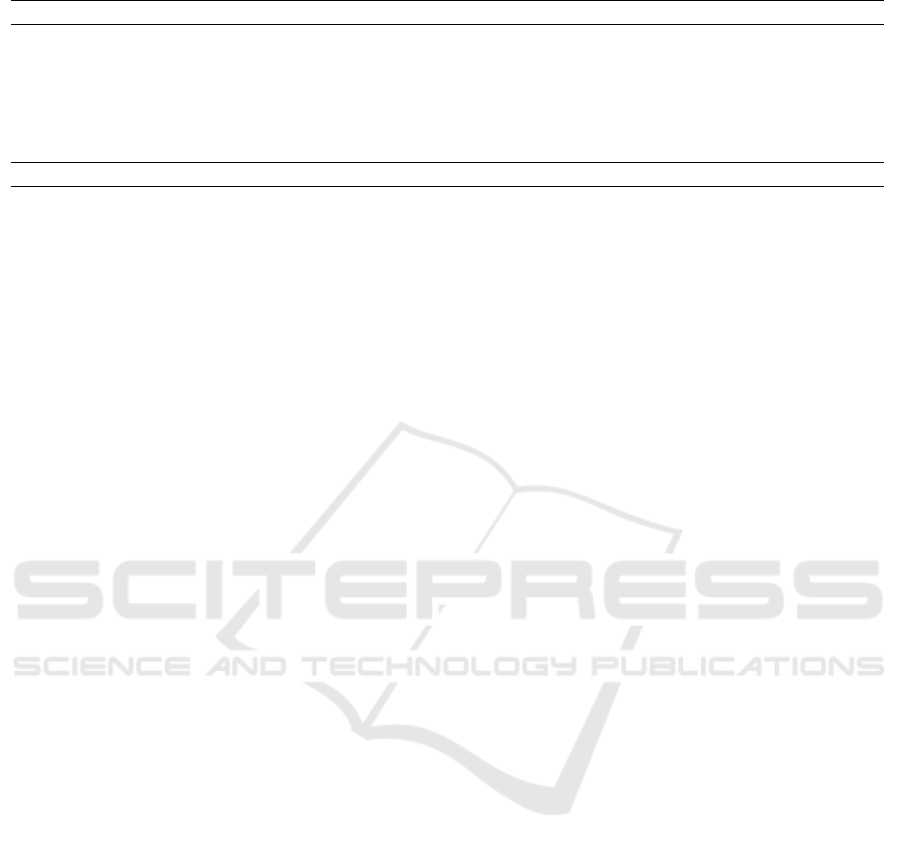
Table 5: State-of-the-art methods on DHG dataset.
Method Accuracy on DHG-14(%) Accuracy on DHG-28(%)
RNN (Chen et al., 2017) 84.68 80.32
SoCJ + HoHD + HoWR (De Smedt et al., 2016) 88.24 81.9
STA-Res-TCN (Hou et al., 2018) 93.57 90.7
TCN-Summ (Sabater et al., 2021) 93.57 91.43
ST-TS-HGR-NET (Nguyen et al., 2019) 94.29 89.4
DD-Net (Yang et al., 2019) 94.6 91.9
Our SPD Siamese Network 95.60 94.05
is enough for the network to recognize the data
classes in the future.
• Due to the feature of distinguishing between the
differences and bringing the similarities closer, it
gives a very high accuracy result (95.60%)
Thanks to these characteristics, we succeed to pro-
pose a new approach that outperforms even the ap-
proaches that were the basis of our approach.
5 CONCLUSION
In this work, we presented a new approach for hand
gesture recognition using skeletal data. The pro-
posed method consists of learning SPD matrix cou-
pled to the use of Siamese network. We have evalu-
ated the proposed approach on DHG 14/28 Dataset.
The achieved results show high accuracy outperform-
ing state-of-the-art methods.
As future work, we plan to study the impact of
using geodesic distance on the SPD matrix within
Siamese network. Besides, we intend to focus on
online recognition systems using short time sliding
windows where we could recognize gestures. Finally,
applying our approach on different datasets and dif-
ferent applications such as human action recognition
could be interesting to study its performance on dif-
ferent context.
REFERENCES
Anirudh, R., Turaga, P., Su, J., and Srivastava, A. (2016).
Elastic functional coding of riemannian trajectories.
IEEE transactions on pattern analysis and machine
intelligence, 39(5):922–936.
Bromley, J., Guyon, I., LeCun, Y., Säckinger, E., and Shah,
R. (1993). Signature verification using a" siamese"
time delay neural network. Advances in neural infor-
mation processing systems, 6:737–744.
Chen, L., Shen, J., Wang, W., and Ni, B. (2015). Video ob-
ject segmentation via dense trajectories. IEEE Trans-
actions on Multimedia, 17(12):2225–2234.
Chen, X., Guo, H., Wang, G., and Zhang, L. (2017). Mo-
tion feature augmented recurrent neural network for
skeleton-based dynamic hand gesture recognition. In
2017 IEEE International Conference on Image Pro-
cessing (ICIP), pages 2881–2885. IEEE.
De Smedt, Q., Wannous, H., and Vandeborre, J.-P. (2016).
Skeleton-based dynamic hand gesture recognition. In
Proceedings of the IEEE Conference on Computer Vi-
sion and Pattern Recognition Workshops, pages 1–9.
De Smedt, Q., Wannous, H., Vandeborre, J.-P., Guerry, J.,
Le Saux, B., and Filliat, D. (2017). Shrec’17 track:
3d hand gesture recognition using a depth and skeletal
dataset. In 3DOR-10th Eurographics Workshop on 3D
Object Retrieval, pages 1–6.
Feichtenhofer, C., Pinz, A., and Zisserman, A. (2016). Con-
volutional two-stream network fusion for video action
recognition. In Proceedings of the IEEE conference on
computer vision and pattern recognition, pages 1933–
1941.
Hou, J., Wang, G., Chen, X., Xue, J.-H., Zhu, R., and
Yang, H. (2018). Spatial-temporal attention res-tcn
for skeleton-based dynamic hand gesture recognition.
In Proceedings of the European Conference on Com-
puter Vision (ECCV) Workshops, pages 0–0.
Huang, Z. and Van Gool, L. (2017). A riemannian network
for spd matrix learning. In Proceedings of the AAAI
Conference on Artificial Intelligence, volume 31.
Huang, Z., Wan, C., Probst, T., and Van Gool, L. (2017).
Deep learning on lie groups for skeleton-based action
recognition. In Proceedings of the IEEE conference on
computer vision and pattern recognition, pages 6099–
6108.
Huang, Z., Wu, J., and Van Gool, L. (2018). Building deep
networks on grassmann manifolds. In Proceedings of
the AAAI Conference on Artificial Intelligence, vol-
ume 32.
Jayasumana, S., Hartley, R., Salzmann, M., Li, H., and Ha-
randi, M. (2013). Kernel methods on the riemannian
manifold of symmetric positive definite matrices. In
Proceedings of the IEEE conference on computer vi-
sion and pattern recognition, pages 73–80.
Koch, G., Zemel, R., and Salakhutdinov, R. (2015).
Siamese neural networks for one-shot image recog-
nition. In ICML deep learning workshop, volume 2.
Lille.
Lim, L.-H., Sepulchre, R., and Ye, K. (2019). Geometric
distance between positive definite matrices of differ-
ent dimensions. IEEE Transactions on Information
Theory, 65(9):5401–5405.
SPD Siamese Neural Network for Skeleton-based Hand Gesture Recognition
401